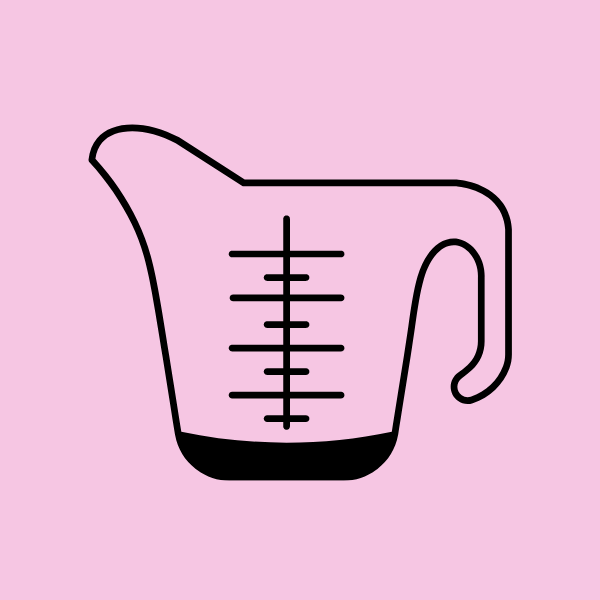
We’ve talked about Marketing Mix Models(MMMs) on this podcast before – and they can absolutely be an effective tool to combat the measurement loss post ATT.
One challenge that we faced and heard about has been that MMMs and incrementality based models are really hard to implement.
As it turns out, that does not have to be the case – and we can very much build an MMM using spreadsheets, as our Senior Mobile Marketing Manager Virendra Shekhawat found out.
In today’s episode, we describe how we built an MMM using spreadsheets for post-ATT measurement. To dive in deeper, you can look at a Youtube video where Virendra walks you through an actual case study and explains the approach in much more detail. Although you can certainly go deeper using code and solutions like Facebook’s Robyn, this is a great way to get started in your efforts to measure performance more clearly post ATT.
Based on the model by Mike Taylor in his blog post: https://www.saxifrage.xyz/post/econometrics-gsheets
Link to Youtube video here: https://bit.ly/mmm-spreadsheet-model
**
Note: We have a growing community of mobile marketers!
The Mobile Growth Lab Slack: A community that was a part of our workshop series – The Mobile Growth Lab, is now open to the general public. Join over 200 mobile marketers to discuss challenges and share your expertise. More details are available here: https://mobileuseracquisitionshow.com/slack/
If you’re ready to join the growing community, fill this form: https://forms.gle/cRCYM4gT1tdXgg6u5


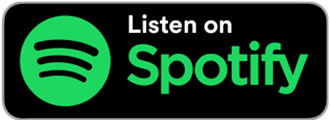

ABOUT ROCKETSHIP HQ: Website | LinkedIn | Twitter | YouTube
KEY HIGHLIGHTS
🪆 The challenges with MMM implementation
🖼 Mapping inputs and outputs
📨 The LINEST function in Excel
📟 Calculating contribution of each channel
⚖️ How accurate is your model?
🔭 Forecasting the future
FULL TRANSCRIPT BELOWINTRODUCTION
We’ve talked about Marketing Mix Models(MMM) and how they can help inform incremental impact of user acquisition channels, especially in the absence of deterministic data post iOS 14. We’ve also talked about some of the challenges in making Marketing Mix Models – and the key one among them is that they can be very effort and resource intensive, or that they will require third party tools.
As we found out in our own explorations, this does not necessarily have to be a limitation. We found out that we could very much build a basic econometric model for Marketing Mix Modeling using Google spreadsheets with surprisingly high R-square(or predictive power). We were also able to use Facebook’s open source framework Robyn to get results surprisingly easily.
Today we’ll talk about the spreadsheet based approach, how we did it – and what our results were.
Because a spreadsheet cannot be depicted over audio(yet!), we have a presentation with an actual walkthrough of a spreadsheet with actual data – presented by Senior Mobile Marketing Manager at RocketShip HQ, Virendra Shekhawat. You can check that out on Youtube via the link in the show notes below.
While you can see the actual walkthrough on Youtube, I’ll describe the overall approach in this audio, so you can get a conceptual understanding of what we’ve done.
Let’s jump in.
Our problem statement, if you will, is to identify how much each of our inputs(or marketing channels) contributes to the output(purchases, trials or transactions). Historically of course in the presence of an IDFA, you could tell which users came from which source – and how many users were contributed by each source(although that certainly had limitations because last click measurement is imperfect too).
What our spreadsheet model will do is basically look at historical trends of inputs and outputs(spends and purchases across different channels in the past) – and provide an estimate of which channels were the most and least valuable, so we can invest accordingly in the future. (What Facebook’s Robyn can do in addition to this is to tell you what your ideal mix of spends should be – and we’ll cover that in a subsequent video).
For now, we’ll focus on our spreadsheet model, which assumes that the outputs and inputs have a linear relationship. In other words, the outputs(purchases, transactions, trials) are equal to the sum of products of a constant and spend on each channel.
First we add inputs and outputs by week in a spreadsheet – so: we have spends by week for every channel, and revenue or transactions by week in your spreadsheet.
We then use the LINEST function in Excel to calculate the equation of a straight line that best fits our data. Again, you can see a detailed walkthrough in the Youtube video – but you should get an equation in the form
Purchases = (Constant 1 * spend on channel 1) + (Constant 2 * spend on channel 2) + (Constant 3 * spend on channel 3) + Baseline etc.
The baseline represents the ‘organic’ contribution or the amount of purchases that you get if you had no spend.
Now that you know the equation above, you can calculate the historical contribution of each channel – by multiplying each constant with historical spend for that week. So: constant 1 * spend on channel 1 divided by purchases should give you the percentage of purchases that were contributed by channel 1.
This is all good – but how accurate is the methodology? How do we know if this is all reliable at all?
We can find out. There are 2 ways to assess the reliability of your model.
One. Calculate the expected outcomes(purchases etc.) using the equation you came up with. How close are the expected outcomes in the past to actual outcomes? If they are close, you should have strong predictive power.
Two. Calculate the R-squared or the coefficient of determination. How high is it?
We’ve found fairly strong predictive strength in our models – but you should test these for yourself, because each product is different.
Once you’ve built your model and ascertained the predictive power of it, what else could you do? You can play around with different scenarios. What happens to your total number of purchases if you double spend on channel 1? Or triple spend on channel 3? You can basically simulate different scenarios and see which of these gets you the strongest CPAs.
Again, this is a starting point – but it’s still enormously beneficial and way better than using SKAN or flying blind.
Check out the detailed walkthrough on Youtube – and let us know if you have feedback or questions.
The video is here: https://bit.ly/mmm-spreadsheet-model
A REQUEST BEFORE YOU GO
I have a very important favor to ask, which as those of you who know me know I don’t do often. If you get any pleasure or inspiration from this episode, could you PLEASE leave a review on your favorite podcasting platform – be it iTunes, Overcast, Spotify or wherever you get your podcast fix. This podcast is very much a labor of love – and each episode takes many many hours to put together. When you write a review, it will not only be a great deal of encouragement to us, but it will also support getting the word out about the Mobile User Acquisition Show.
Constructive criticism and suggestions for improvement are welcome, whether on podcasting platforms – or by email to shamanth at rocketshiphq.com. We read all reviews & I want to make this podcast better.
Thank you – and I look forward to seeing you with the next episode!