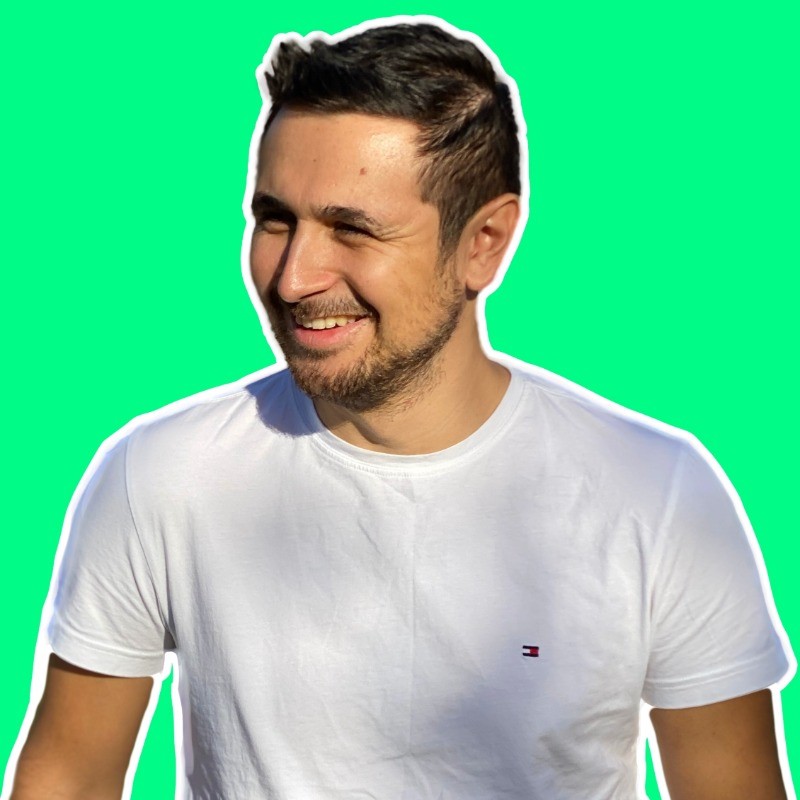
Our guest today is Alexei Chemenda, Founder and CEO at Poolday.ai, a platform that uses AI actors to generate videos.
In today’s episode, we discuss the evolution and current trends of AI-based creators in video ads.
Alexei shares insights from his experience, including the challenges and breakthroughs in producing AI-driven ad content, and how it is making testing and iteration faster and easier.


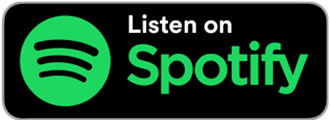

About Alexei: LinkedIn | Poolday.ai |
ABOUT ROCKETSHIP HQ: Website | LinkedIn | Twitter | YouTube
KEY HIGHLIGHTS
🗒 AI creators transform video ads with realistic presentations.
📈 Rapid technology advancements meet the growing demand for video content.
📍AI’s evolution enhances emotional engagement in advertising.
🔐 Efficient content creation challenges traditional methods.
✂️ Custom AI models democratize access to sophisticated tech.
🔍 AI enables the mass production of varied ad creatives.
✏️ Testing small changes yields significant performance differences.
📌 AI fosters innovative approaches to personalized marketing.
FULL TRANSCRIPT BELOWSHAMANTH:
I’m excited to welcome Alexei Chemenda to the Mobile User Acquisition Show. Alexei, welcome to the show.
ALEXEI:
Thank you. I’m excited to be here.
SHAMANTH:
I’m excited to chat with you also because I think we’ve known each other for a very long time, nearly 10 years.
I’m happy to be speaking today because I have seen a sneak peek into what you’re building, and that is something you’re going to talk about today, which is videos and ad creatives that feature AI-based creators.
Excited to dive into all the things AI and AI creators today.
ALEXEI:
I’m excited as well. I feel like we’ve evolved a long way, and I’m glad to be here.
SHAMANTH:
For the last year or so, since AI-based presenters came on the horizon, they’ve generally come across as robotic, unemotional, and unreal, and that’s been changing lately. So, what, in your opinion and understanding, is precipitating this change?
ALEXEI:
There’s been a couple of things. The video format in the world has been scaling fast. And so, people need to produce more videos. At the same time, technology has been moving fast as well. You look at companies like ElevenLabs producing audio.
Technology has been making a lot of progress. And really, the combination of the need for more videos and technology making good progress is where we see a lot of improvement happening from purely making the videos more realistic, with the humans more realistic. And that combined with the fact that it’s harder and harder to work with content creators, they’re less and less reliable.
And so, it’s kind of a perfect storm for those very realistic videos to come along and those AI actors to be creating as many emotions as a human would. And it’s an exciting journey, on the marketing side.
SHAMANTH:
And technologically, what’s changed to make this happen that wasn’t possible before?
ALEXEI:
What changed technologically is a lot of it. If you look at companies like HeyGen, for example, or Synthesia, they’ve been working at it for a good amount of time. They’ve only been pretty popular recently, but they’ve been working hard on the R&D side for a while.
And really, the realization from people that AI can be leveraged authentically only started when OpenAI released ChatGPT. OpenAI is about a decade-old company. But they’ve been sitting in the dark, producing their hard work hidden from the world.
And when they unleashed ChatGPT, I think people realized, okay, there is something very real here. The manipulation of LLMs is something that small teams can do too, and then you get a whole number of small teams working on this and who found ways to improve it. You’ve had companies like Hugging Face emerge to help simplify access to custom models to LLMs, and I think the culmination of that is you have OpenAI, Hugging Face, you have small teams working on this, and this is why now a lot more people are investing time and money into this.
SHAMANTH:
And I would also imagine that as many of these models have more training data the fidelity and accuracy of the output just improves and increases to be more and more realistic, right? This was just earlier on. They just wouldn’t have as much training data to produce anything realistic or believable.
This is where the need for videos, and the fact that videos are emerging, are great because the video is a very rich data set. If you look at it, there are typically three years of data set you can have: text as the first, image as the second, and video as the third. You can add voice and so on in there, but generally, at a high level, those three layers are a different order of magnitudes of how much information they carry.
ALEXEI:
And if the world had gone towards more Twitter or text formats, then the level of richness of the data is very small. If you look at video then all of a sudden, we get an immense data set, and yeah, the first videos produced by AI were crappy, but that’s what you need. You need to have that and test and learn to videos.
SHAMANTH:
Sure. But I would also point out that the atomic units of videos are harder for an LLM to build on as compared to text, or with enough training data, an LLM could complete a sentence in text easily as compared to building a video. So, I imagine that there is also a layer of complexity in that. That’s harder to mount for an LLM.
Is that something you would agree with, what do you think?
ALEXEI:
Yes, but there are ways to circumvent it. If you think about creating a video from scratch and you have to generate 60 frames a second for 30 seconds, then you have a lot of frames to generate. There’s a lot of compute power or server costs associated with it. Now, if you think about which parts of the video can I come up with versus which parts of the video I need to generate? And you start to isolate variables that are not needed from an AI perspective, all of a sudden, you go from a very high complexity to a medium to high complexity.
You isolate the variables where you need the AI to generate, and if you look at your text, yes, it’s easier for an AI to generate text, the next word to be completed than the next frame, but even that, there are layers in there.
How big of a text do you want the AI to generate and how big of a context window do you want to give to the AI?
We see OpenAI playing with this context window and how much context can you give to have the AI work. And so they started small and they’re expanding, and same for video. You start with certain parts. And then that makes it easier to process.
SHAMANTH:
That’s so fascinating. And you know, the more I dive into what happens under the hood, it makes sense, but it’s still somewhat unreal and still hard to believe, which is why we live in such exciting times. It’s not just a cool, sexy tool. It is yielding meaningful marketing results, And you know, the reason we’re talking about AI-based creators is not because they are sexy or great or fancy, but because they can drive performance.
You’ve worked with human creators, and now with AI creators. How are you seeing performance differentials between the two?
What do you see as being determinants of the performance differentials?
ALEXEI:
What we notice is that we’ve run a few experiments at large scale, one of which is interesting.
We have taken two ways to create an AI persona or an AI content creator. The first is to take a real person and create their digital copy, to create an AI version of themselves that looks like them and talks like them. And the other is to create a person from scratch that doesn’t look like any real person out there. We’ve done both. And what we’ve measured is, the experiment we’ve run is taking an AI actor and taking the human version of that AI actor—or the other way around, rather, to take the human actor and take the AI replica—and we’ve tested at scale to see the performance of these two different versions of one person.
Interestingly, the performance initially, if you look at three, or six months ago, the performance was subpar for the AI actor because the voice was still not super realistic. The movements were not realistic. And then gradually, we continue improving on it.
Then the results have been staggering; the AI actor consistently outperforms the human. But to be clear, when we compare two videos, one human, and one AI, both perform in a very similar fashion. What makes the AI actor perform better is that now, all of a sudden, instead of creating one video with a human and one video with an AI actor, you get to produce one video with a human and at the same time, a hundred videos with an AI actor.
And chances are, as evidenced by the data, chances are one of those videos will outperform the original video made by the AI actor or the human. So, I think that the key learning for us is, that we’ve looked at AI actors not just as a way to reduce costs or improve efficiency, but also as just a way to test more things that you wouldn’t have tested otherwise and test that otherwise with human creators.
SHAMANTH:
That’s a very great point, and it is something I’ve seen in our fairly limited experience as well, in that I don’t think the performance can be attributed to the fact that this person’s a human or not, especially at this stage when AI actors are increasingly realistic.
You could even argue that with a lot of AI actors, a user would know and see and understand that this is an AI actor and they wouldn’t mind that this is an AI actor. And I think there could be some ads like that, but I think you’re right. What AI does do is just allow you to test a lot more variables. In the past, you would test one variable. Now, you’re testing 10 or more. The cost of testing just goes down. So, it’s not so much humans or AI, but it’s just what the AI is enabling and unlocking.
What are some of the variables you guys test, and what do you see that makes the biggest difference in performance?
ALEXEI:
The emotion is one. What kind of emotion does the actor transmit? What kind of words, keywords specifically per app, are performing well? What sort of hair color? What look?
I do see a world in which every single mobile app and every single company out there has an AI ambassador if you will, or an AI mascot that they use. Today, you get the Tony Parkers or the Tiger Woods of this world who get sponsorship of this scenario with Serena Williams who are brand ambassadors for certain companies. Tomorrow, I do see AI people being that way. And so, I see companies creating their version of what they think works best or what they test works best. Those are the main variables, and obviously, then if you look at the video in general, the typical usual suspects like what music you put in, what call to action, what hook—we’re doing a lot for the TikTok ecosystem.
And we see being able to just swap out some words with other words just makes a huge difference. The first one to three seconds, the hook, is where we see a lot of power just by A/B testing a lot of different things, a lot of different sentences, and a lot of different words used. And the looks are certainly very powerful.
Suppose, in a meditation app they want to create an environment in which the AI actor is very uniquely placed. And that can be, oh, let’s try this actor that works great for us but on the beach in a very relaxing setting, or a yoga studio, or in a massage room. And so, you get to just experiment with a lot of different backgrounds that make a lot of sense for performance.
SHAMANTH:
And as you said, just the sheer number of tests you can run becomes very powerful.
Speaking of the number of tests you can run, some advertisers might argue, yes, AI can allow them to generate hundreds of variations very quickly, but it’s just not so realistic or practical to be testing those, especially given the Bayesian nature of the testing algorithms where the top ad gets the most spend, and everything else kind of languishes, or the top three or four, or the top 20 percent get the most spend, and everything else just doesn’t get enough conversions.
So, if you have hundreds, is there a risk that the vast majority just don’t get to spend or learn? And I would imagine that problem gets exacerbated if you have a high AOI product with a high CPA. Because I’ve worked with products that have a $100 or $150 CPA, and to be testing those rigorously, to get enough conversions, to get enough learnings, is a real challenge.
So, what do you think about that? How do you suggest and recommend testing high-volume creatives?
ALEXEI:
There are two answers to this. The first is the short term and one is the long term. The short term is, we use a platform like TikTok. You said something really interesting. You said, how do you test accurately? In a way that doesn’t get one winner-takes-all-all kind of format.
TikTok is very good for a winner-take-all format, which means you upload 10 videos in an ad set or an ad group, and one of those will eat up 95%. If you’ve only put a budget at the ad group level, only one of those videos will get maybe 90% or 95% of the spend.
And people are frustrated with this. And whenever I talk to marketers they ask – how do I get every other video to spend? And I like to flip the other question, like, why would you want the other videos to spend? If that particular video is working well, then you want to scale it up, and produce more.
And the way we’ve been testing, we’ve produced north of a hundred thousand videos in the last 12 months. The way that we’ve produced videos and tested videos is by letting TikTok figure it out.
So, if we produce 50 videos, we create three or so ad groups, we upload 15 videos per ad group, and TikTok only picks one winner. If that winner is going to be a unicorn ad and it’s going to outperform the rest and drive growth profitably, great. So then, my question becomes, how do I reduce the cost of generating 50?
And that’s when AI comes in. But for testing, we’re embracing that, and we’re letting TikTok figure out. They’re great at predicting what’s going to work or what’s not going to work. And most of our videos, get $3 of spend, and they get cut out, and that’s okay because we gave TikTok what it needed to figure out which one is going to work best.
These learnings from TikTok, once the TikTok algorithm has spit out a video that works great, then we can reuse it on, the usual suspects on Google, and I’m loving them, on Meta, and so on. You kind of need to tweak a little bit, but again, this is where AI can come in to help you tweak accordingly. So that’s the short-term answer.
And then the long-term answer is, I do see a world in which every platform will allow for one-to-one marketing at scale. And we currently think of videos as, oh, I need to find a top performer. But really, I don’t want a top performer. I want a video that’s very relevant to you.
And that video might be very different from the one that’s very relevant to me. And so I think eventually, the platforms will allow for one-to-one marketing at the scale of billions of users where every single person sees something different that’s relevant to them. And then you no longer think about winner-takes-all all, but you think about how to make sure that the videos are super relevant.
SHAMANTH:
Just to double-click on one of the things you said, I agree that the winner-takes-all-all is a very valuable signal. If the algorithm is giving spend to one single ad, that’s a good signal. It just means that the ad can scale. That means users can and will resonate with that.
A couple of follow-ups on that: Is there a risk of false positives or false negatives in that? I’ve certainly seen instances where the algorithm gives a ton of spend in the first level of test to one ad, and spending doesn’t always correlate with strong performance CPA.
Similarly, something that spent three dollars and just got cut out could be a very high performer, which ties into the other aspect of what I said earlier, which is, what if the target CPA is extremely high? Are you still optimizing for a 150 CPA with 10 conversions or more? What do you think about these dynamics of the testing mechanics?
ALEXEI:
It’s something we spend a lot of time on. What you described is what happens across the board where yes, some videos that are only getting a few dollars and getting cut, there are some winners there. The way that we at least identify those winners, is we let, in this case, focus initially our efforts on TikTok before going to other platforms. So on TikTok, we let the winner emerge. That winner is then taken out of the ad group and tested on a different ad group, on a different campaign that is more at scale with more optimized data points, and we give it more budget.
I always think of the other way around, or if the video cannot be spent, it doesn’t matter how good it is. You will not be able to scale it. So then the first barrier to entry is, how do we make sure that the video can get spent? And that’s not up to us, it’s up to TikTok or Applovin or Meta or the other platforms.
So once we identify a video that can get scale, great, let’s give it some scale and then you can surface. But the main issue we’ve seen with mobile marketers is that they’re not getting good spending. So the performance is horrible, or they’re not able to get good spend.
So the first problem to solve is, how do we get the creatives to scale? Once you are at scale, you can collect more data points, and you can figure out go/no-go on that particular video. If it’s a go, great, continue putting it in a BAU (business as usual) campaign.
If it’s not a go, kill it. But for the remaining videos that were in the ad group, you continue testing them, you continue producing more.
And you give each one a chance to see, okay, is there one that can still outgrow?
That’s sort of how we think of it. And certainly, there are false positives and false negatives, which is why we want a multilayered approach to testing. But the first layer for us is testing, can you even scale the creative? And do the early signals of the performance make sense from your profitability or CAC goals?
SHAMANTH:
A hundred percent makes sense. And for that first level of testing, where you have 15 ads, what sort of budgets do you typically have, and how many conversions, if that’s what you’re looking at, are you waiting for before you make a decision?
ALEXEI:
This is on a case-by-case basis. We typically look at, if we look at an app that has a CPI goal usually, they have robust goals or some sort of down-funnel proxy, and that’s a valid event. But if we look at a proxy like CPI, a lot of apps are in the 1 to 5, between 1 and 10, let’s say, and then some are on the higher end target at CACs.
The first thing we look at, like I said, we use TikTok to surface things, and then we deploy to other platforms. So for TikTok, we look at, is it getting spent? Is it getting a good click-through rate? Because without clicks, there’s not going to be any profitable conversions, at least on TikTok.
And then we’re looking at the number of conversions, dependent on what the CAC is, and we try to stay within a few times of total spend. Okay. So at least a few times the CAC. So if it’s a $5 CPI sort of proxy, we can afford to drive hundreds of installs and evaluate afterward. If it’s a CAC target of say $150, or $200, some are thousand-dollar CAC targets.
Now then, we try to stay within two to three times that. It’s really on a case-by-case basis. And I would say the lowest number we’ve looked at is typically two to three times the CAC for larger CACs.
SHAMANTH:
I think that makes sense. And that mirrors our approach with high CAC products where sometimes it’s just not financially efficient to be testing to $150, $200, or higher CACs.
Would you test separately on Meta, or are you just taking the winners from TikTok and moving it to Meta? Why or why not?
ALEXEI:
It’s a great question. We don’t start with Meta because we see Meta as not being, aside from everything that’s happening right now, for the last you know, 30 days, CACs are going insane on Meta and issues with the platform and so on, which I’m sure you’re aware of.
But aside from what’s going on right now, the creative testing methodology for TikTok, for us, just works best because it’s able to quickly churn out most of the videos and winner takes all. And Meta is not as strong with this. However, once we surface those videos, we put them on Meta; we don’t go through the same process.
Meta, AppLovin, Google, or Unity, platforms, they get a selected, refined number of videos. We do the big testing on TikTok. We keep the top five or ten—obviously, it depends on the number of videos produced originally. And then those top are deployed to other channels, and we’ve measured proximity scores.
So what is the likelihood of a video performing well on, let’s say, AppLovin, if it has performed well on TikTok? And the proximity scores to TikTok, the highest one, is Meta and AppLovin. We haven’t measured a proximity score for Unity, but we will soon.
This tells us that if a video works well on TikTok, there are high chances of it performing well on AppLovin or Meta, but it’s not a one-to-one relationship. That’s why we need more than one video to be deployed to Meta, from TikTok. At least, three to five, to find one that will work on Meta.
And by the way, the other way around is not true. Like, the proximity score is a one-way score. So if a video works great on Meta, obviously it doesn’t mean at all that it will work great on TikTok, and chances are it will not. So that’s why we measure the proximity score from TikTok to other channels.
SHAMANTH:
For sure. And if I might ask, the auction mechanics are very similar on Meta and TikTok. There’s a very similar base here and spend allocation mechanism. It’s a very similar winner-take-all dynamic. So in what way, other than the fact that TikTok is very optimized for virality, other than that, why would you not test on Meta given the similarity of the auction dynamics?
ALEXEI:
Basically, it’s how fast you can get to a video that is killed or won. And TikTok is just much faster for us, at least. I’d be curious, if you have other instances, I’m happy to share notes there.
What we’ve seen is TikTok is much faster at churning out. So, they go through the same process, but TikTok just instantly kills. And they have a lot of predicting capabilities that are under the hood. They’re not surfacing this to the account, but when you upload a video to TikTok, they know if they’re going to spend or not before you even spend on it. That’s sort of why we use TikTok in the first place, and to save time on duration cycles. I’m curious if you use Meta for testing purposes; that’s something we want to learn.
SHAMANTH:
We have leaned on Meta just because that’s by default. But I think the one reason we’ve not considered TikTok actively was just because Meta has stronger monetization signals than TikTok. Which is to say, for a lot of products, TikTok has very strong cost per install. TikTok is great at driving install, but if you do a side-by-side comparison of Meta versus TikTok, the cost per purchases or the cost per downstream event, trial purchase, ROAS tends to be stronger for Meta. And that has been the primary reason we stayed with Meta.
But I would also say we haven’t tested on TikTok aggressively enough just yet. So, that could certainly be something for us to think about and test as well.
ALEXEI:
I agree with you on what we see. It depends on the vertical, but some verticals are really just outperforming on TikTok. But I agree with you that Meta is really strong from a down-funnel perspective. And I wonder if you were to do your testing on TikTok, I wonder if you would be able to extract videos that are good to then test on down-funnel optimization.
I agree with you, and I’m just wondering if there’s something to TikTok specifically for creative testing, regardless of the down-funnel.
SHAMANTH:
Interesting that you mentioned that because one of the accounts I’m working with does that, and that’s just because they have TikTok creative challenges, and they’re like, we have like these tons of videos, and we are forced to test this. Therefore, let’s just test these on TikTok and move the winners to Facebook, but that’s been enforced by the constraints of the TikTok creative challenge.
But from everything you’re talking about, I think it absolutely makes sense to run the test on TikTok in the way that you’re describing. And you’re right, and this is something we are going to test out now, and since we’re talking about it now, it could be worth it, running a test for an up-funnel event on TikTok even to surface what the algorithm likes.
And that could be just even installs, and run just the winners on Meta, use that TikTok algorithm to surface winners and then, I think that could be a good approach, that could be an interesting approach.
ALEXEI:
Yes. A hundred percent.
SHAMANTH:
This is one of the questions I wanted to ask you because we do spend a lot of time thinking about the testing mechanics and dynamics and about the best ways to be tested.
I have something that I want to take away and test right now with the testing on TikTok especially with AI-based creators. That’s certainly an opportunity.
You did mention you’ve tested over a hundred thousand ads. What have been the most surprising or unexpected lessons and discoveries in your tests so far?
ALEXEI:
What have been the most surprising learnings from our testing of a hundred thousand videos? I would say it’s the insanity at which one word or one small change can make in terms of what is the output at the end. And so, the impact of changing one word on the video performance.
This to me is something completely unbelievable. The benefit of using AI actors is that it’s very easy to then tweak. In the past, what we had to do was we had to call the content creator back or the actor back, and we had to ask them to rerecord, but they have the same makeup, you need to make sure they’re wearing the same clothes, otherwise, it’s not an A/B, it’s really B testing capability, but here, you get the exact same video, and you change one word, or you change the first sentence, or you change something very very small, and it’s the power of those small changes which I’m not pretending like this one word is make or break for the marketing strategy of the company but for the algorithms on the platforms like TikTok and Meta and so on, that one word can trigger something a little bit more different on the user side.
On the consumer side, if the user is triggered, maybe they leave a comment, maybe they download. It’s not even that they will download because of the word, it’s that they pay more attention to that specific video. They give you a slightly longer opportunity to close them. So if a user is watching a video for three seconds, you don’t have any opportunity to close them. If you get them to watch until seven seconds, okay, you’re having a conversation.
So, this is probably the one aspect we did not foresee is the impact of those small changes and therefore the importance to run those unique combinations at a large scale. And that’s been mind-blowing.
SHAMANTH:
Yes. I can imagine. And it’s only because you are running so many tests that you’re able to surface these opportunities.
ALEXEI:
I didn’t throw in a data point there, but I’m happy to throw in an experiment. We run across many different verticals at scale. There was a 10x delta between one video and its immediate iteration. And immediate iteration is you change only one item, and this one item, in this case, was the first few words pronounced.
There’s a 10x difference,or we’ve measured a 10x difference on the cost per install. We didn’t go as far as ROAS and so on because those are private information that we can’t necessarily use as much, but for CPI, it’s a 10x difference by changing the first three words of the video, and that’s what I mean by mind-blowing, right? A 10x difference is like you get a CPI of 15 or 1.5. That’s completely game-changing.
SHAMANTH:
My follow-up to that was, how do you keep track of these experiments? And how do you decide what variables to test? Because I would imagine a lot of the variables you’re testing may not be that meaningful or significant. How are you tracking these things?
ALEXEI:
That’s how we’ve built the platform. So, Pooldata.ai is exactly that. It’s generating videos using AI actors but then also centralizing this experiment’s data. And the way to think about centralizing experiment’s data is, depending on how large of a company you are, if you’re a very large company, you have a huge amount of data set, this looks more sophisticated.
If you’re on the smaller side, it’s not reasonable to expect a marketer at a smaller company to drive a thousand experiments using very sophisticated variables. Because the reality is, your data is not going to follow, and the volume of data required is not going to follow.
And so, what we’ve done is, for the simpler experiments, we have certain variables that we’ve just measured as having a higher coefficient, a higher weight importance in the video, and those we surface. And we say basically, here are the 100 videos you’ve produced, or here are the 10 videos you’ve produced, and among those videos, here are the different variables that haven’t changed. And this is the outcome, aka here are the variables that work well for you. So like, this AI actor works well for you, music this, X, Y, and Z, and for the sophisticated ones, we can essentially use computer vision to extract information from the videos to say, well, here are the patterns that are extracted, and we can go through a lot more variables.
For the simpler experiments, there are typically four to five variables that we see as being the most weighted variables. Those are, the hook, the actor, the thumbnail.
People ask me, what the hell is a thumbnail on TikTok? The thumbnail is the very first frame that a user sees. So, it’s the very first image that the user sees. So, that thumbnail, you can keep the same video but change the thumbnail, meaning the very first frame. And typically, it means, like, for example, the background, right? It’s the very first frame that you see, and that you can iterate a lot on. So, the actor, the hook, the base story, obviously the background or a thumbnail, and then the metadata like captions, and you know, the editing style.
Those are probably, dumbed down or simplified, those are the main variables that matter. And then there are a lot more, like the pace, the emotion, that like, you can go pretty sophisticated.
SHAMANTH:
When you talk about the background and ambiance, would it be accurate to say one of the few things that AI can’t do yet is having somewhat customized background which could be actors cooking in the kitchen and showing a brand of cookware? Is that among the things that AI can’t do yet, or am I mistaken on that?
ALEXEI: It can. It’s more sophisticated, but it can. There are exciting ways, like we have clients who want AI actors at the gym lifting weights or like very realistic scenes. So, there are ways to go around it. And it goes back to what I was saying earlier, which is, whenever you have a complex problem, like breaking down into smaller problems and then see what you need AI for, see what you don’t need AI for, and you can sort of work around that. But the power of A/B testing using very realistic scenes like in a kitchen or in a gym or whatever is not a theoretical maybe down the line, it’s live already, and the technology is already here and available.
SHAMANTH:
Amazing. It reminds me of this quote, “The future is here, it’s just not evenly distributed.” And I think about that a lot. The other quote I think about “Any sufficiently advanced technology is indistinguishable from magic.”
This is perhaps a good place for us to wrap, but before we do that, can you tell folks how they can find out more about you and everything you do?
ALEXEI:
You can find us at poolday.ai. We are a self-serve platform that allows marketers to generate tens or hundreds of videos using AI actors in a matter of minutes. And leveraging this capability for A/B testing at scale to really find winners that help them, you know, increase ROI on UA. We are going to be at MAU in the first week of April. And so I’m happy to see any marketer there. Otherwise, my email is alex@poolday.ai. Anyone can hit me up, and I’m happy to answer any questions.
SHAMANTH: We’ll certainly link to poolday.ai, and this is perhaps a good place to wrap. Thank you so much for chatting with me today.
ALEXEI: Thank you so much for the invite. Have a good one.