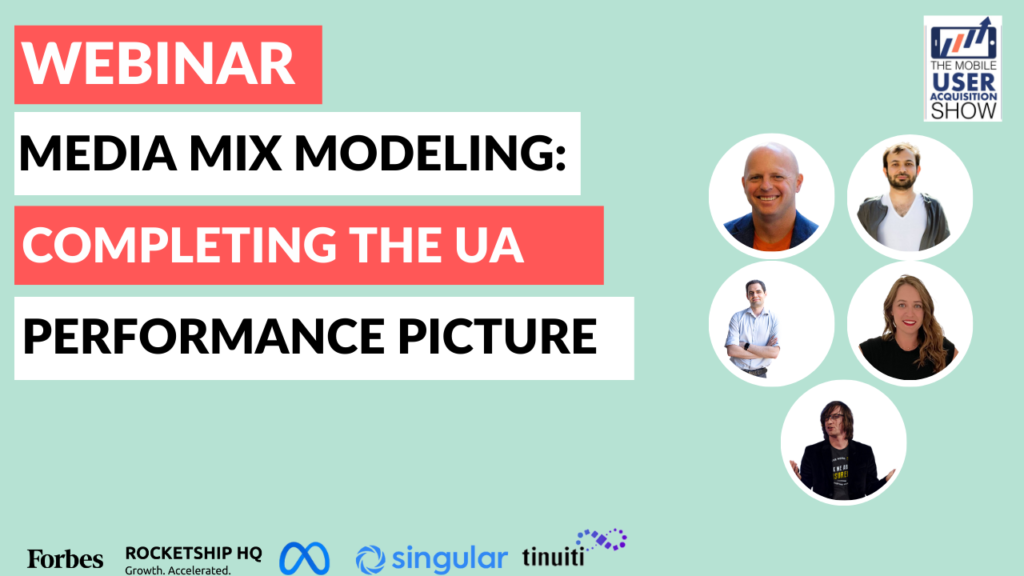
Is MMM a complete solution for mobile marketers out there?
Today’s episode is the recorded version of a webinar organized by Singular that had a star panel with John Koetsier – Writer at Forbes, Igor Skokan – Marketing Science Director at Meta, Liz Emery – VP, Mobile + AdTech Solutions at Tinuiti, Eran Friedman – Co-founder & CTO at Singular and myself.
We discuss all about MMM and how marketers are using it as part of their toolkit, how it has evolved from being a traditional tool used by large companies to something that’s seeing increasing interest and adoption in the mobile growth industry post-ATT.
We also talked about how smaller companies can begin implementing MMM without spending a bomb on it and give some real-world examples based on some of our wins as well.


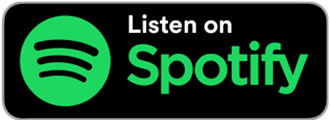

PANELISTS: John Koetsier | Igor Skokan | Liz Emery | Eran Friedman | Shamanth Rao
ABOUT ROCKETSHIP HQ: Website | LinkedIn | Twitter | YouTube
KEY HIGHLIGHTS
💡What is Media Mix Modeling and incrementality?
🎈How MMM has evolved to be used for digital marketing
🛤 Is it for small, medium or large companies?
🧗🏿♀️ Challenging elements of MMM, and incrementality for smaller teams.
🏌🏽♂️Elements of MMM or incrementality that are easy to implement, even for the small teams.
📝What are the stakes to get started with MMM?
🍦There’s more interest in the adoption of MMM.
🎯What can MMM deliver if all the data is in the right place?
⛳️Where would you not use MMM?
🧭How does MMM & incrementality fit into the concept of hybrid measurement?
🌎Real-world examples of using MMM
🌪The role of first party data.
🧳When will MMM be a core part of the majority of digital marketers’ toolkits?
📖Key takeaways on MMM & incrementality
KEY QUOTES
A classic way to do incrementality testing
A classic way to do incrementality testing is you run an A/B test against an audience when one group sees an ad and another one doesn’t see an ad and basically tries to wrestle through whether the user should sign that we actually increment that to get better results.
The mess post ATT
We really were prompted to move to MMM just because post-ATT, everything’s such a huge mess. We talked in one of the earlier conversations about how we were seeing CPAs, like 1000+, 500+, and it’s just not meaningful. That’s what prompted us.
Who is MMM really for?
If you’re a large company, you can invest in this in different ways, you can customize it in very detailed ways when you’re putting together MMMs but when you’re a smaller company, you can start small and still grow it.
The challenge of MMM and Incrementality for smaller teams
I think the hardest thing for smaller teams is the data requirements. It’s not having the data, but is that data available? Is that data clean in a way that you can use it? Because MMM is not what we like to call a push-button solution. As much as we would love it to be a push-button solution. There’s a lot of manual work that goes into it.
Increasing interest in adoption of MMM
Most of us mobile marketers grew up, if you will, with the D7 ROAS and act based on deterministic attribution. That’s been around for a decade. That’s what the reporting has always featured. Now if you’re like, we’re going to look at the share of budgets, or we’re going to run five different models, it’s not even like speaking that same language. Also, it just requires a different skill set.
Real world examples of MMM
For something like Google, where you’re just basically trusting Google-reported numbers on iOS, numbers look wildly optimistic and numbers look crazy because of Firebase, they’re the referee and the player. Obviously, numbers look very, very good. It turned out, we were spending way more than we should have been. When we did cut the budget, we just saw no change in the overall trials baseline. We just cut the budget by 66% because the analysis said Google wasn’t driving anything incremental. Nothing moved and that was just a huge win.
FULL TRANSCRIPT
John
Welcome, everybody. This is going to be a wonderful webinar. We’re talking about Media Mixed Modeling. We’re talking about completing the user acquisition performance picture. It’s super exciting. We have an amazing panel and obviously a super hot topic. We all know, app tracking transparency from Apple was kind of the catalyst, but we should have known earlier that there’s been a trend to privacy, which means granular deterministic marketing data tied to an individual, it’s either gone, or it’s going away quickly.
It doesn’t matter if you’re talking about IDFA or about the Google Ad ID, about third-party cookies, it’s been replaced by aggregated data, sometimes deterministic, sometimes modeled. That transition so far on iOS has been pretty messy. It has forced marketers to think about other ways of getting the performance data that they absolutely need.
What’s working? One of those, of course, that they’re thinking about is a 50-year-old technology or process or idea, Media Mix Modeling. Today, in the context of performance, and mobile marketing, we’re going to ask and answer, what can it do? What can’t it do? How are marketers using it right now? And where does it fit in the mobile marketers’ toolkit long term?
We have Liz Emery, who’s the VP of mobile & Ad Tech Solutions at Tinuiti. We have Shamanth Rao, he is the Founder and CEO of Rocketship HQ. He does amazing work that he’s going to talk about. We also have Igor Skokan, who is the Director of Marketing Science for Meta. And last but not least, we have Eran Friedman, who’s a CTO and Co-founder at Singular, having built some of the highest throughput data systems on the planet.
Okay, here’s what we’re going to do today, we’re going to briefly define our terms. What are we talking about when we’re talking about MMM? What are we talking about when you’re talking about incrementality? We’re going to list the technologies and solutions you need to even think about getting started. We’re going to share what it can do, about how accessible it is, who can use it, what you need for it, and maybe how accessible it will be for even small teams in the future.
We’re also going to look at real-world usage. We will also answer your questions. So if you have questions, we want to hear them.
Before we get started, Eran can you please introduce Singular?
Eran Friedman
Yeah, of course, John. Singular is the next generation of analytics solutions. Our product unifies data from marketing sources connected with the best measurement tools from SKAdnetwork to Media Mix Modeling and provides insights to drive growth. We work with the top brands in the industry. You can see some of the logos here and always feel free to write to us if you’d like to chat.
John Koetsier
Thank you so much.Now to give our panelists a sense of how to approach their answers. Let’s begin with the first poll right now. It’s about what you’re doing right now.
The poll is, are you doing Media Mix Modeling right now?
Let’s see what those results are. No – 25%. Thinking is 28%. Working on it for the future And 9% wizards.
Eran, kick us off by giving us traditionally, what do we mean by Media Mix Modeling and incrementality?
Eran Friedman
Media Mix Modeling, sometimes referred to as MMM, is a methodology that has existed for a long while now. Traditionally, it was used by large advertisers like Fortune 500 companies who are basically trying to model the effect of their media spending on their overall revenue sales or general performance using statistical methods. Think about this as kind of like a top-down measurement framework. It takes aggregated spending broken down by channel or country or any kind of segmentation that you have, like what’s happening in the market or any special events that you had, and then try to output the estimated ROI essentially on your marketing spends, and can also be helpful with budget allocation, all kinds of interesting insights on how are you performing and what should you do.
Now incrementality measurement that you’ve mentioned, is kind of a connected question on trying to understand how truly incremental is your media spend. Or in other words, if you wouldn’t have run that campaign, what would be the results then?
A classic way to do incrementality testing is you run an A/B test against an audience when one group sees an ad and another one doesn’t see an ad and basically tries to wrestle through whether the user should sign that we actually increment that to get better results.
But if you think about how those are tied together, you can think about two ways.
One way MMM, kind of thinking about the top-down measurement, can try to answer incrementality questions like whether your actual spending is truly incremental or not. But you can also use incremental testing to help your MMM model, so if you have some testing that you’re doing, you can feed that information to your MMM, to calibrate it and make it more accurate. So both of these kinds of play very well together essentially.
John Koetsier
Igor, maybe pop in here, you’re doing some super interesting work at Meta on MMM, anything to add?
Igor Skokan
At Meta we have long advocated incrementality. The first time I mentioned incrementality measurement, it was the gold standard for making sure organizations recognize the effectiveness of their campaigns and their marketing activities.
We’ve seen successful advertisers who have applied incrementality throughout the funnel using the different tools that are available for it, they are the ones that we have seen perform better. So incrementality for us is the North Star.
On the other side at its core, it’s an experiment, a testing control experiment.
We also think about incrementality as a spectrum. So on one hand, we have randomized control trials that are giving us the precise causality of marketing to outcomes. And then on the other side, we have some of the most basic attributions and let’s say counting MMM would be somewhere in the model incrementality. So, MMM can be calibrated with incrementality. It gives the baseline as an incremental piece in a modeled way. The approach is the source of incrementality from a different perspective. But it can be and should be calibrated with ground truths obtained by experiments.
We also sometimes refer to it as a Marketing Mix Model, because MMM itself actually is much more powerful. Even the marketing bit, It’s more like holistic business modeling. It goes way beyond media, even way beyond marketing into business drivers, price distribution, even weather, all kinds of things that impact business can be included in the model and should be included in the modeling.
John Koetsier
Love it. I’m always conflicted. Is it media? Is it marketing, I swap back and forth between the two.
Shamanth, I’d like you to ping in here. These are old technologies and techniques, but you’re actually adapting them for digital marketing, and mobile user acquisition, how have you evolved them to make that work?
Shamanth Rao
We really were prompted to move to MMM just because post-ATT, everything’s such a huge mess. We talked in one of the earlier conversations about how we were seeing CPAs, like 1000+, 500+, and it’s just not meaningful. That’s what prompted us.
How are we adapting this – there’s a lot of media data that is already available and especially if you’re talking to mobile apps, you don’t need to rely on weather, gas prices, consumer price index, or what have you. Like Igor was saying, in an ideal world, all of that would be essential. But if you’re downloading a mobile app, the most critical actions typically happen in the first couple of days, the first purchase or the trial, if you will. So really, adapting traditional media mix modeling to mobile or digital is a lot easier than it would be for Nike or Coke or what have you.
John Koetsier
Liz, is there anything to add because you’re using that tool as well?
Liz Emery
I always like to level this saying that measurement used to really be based on and sometimes still is individual platform dashboards or siloed views that don’t always account for external factors, don’t account for what other channels are influencing it, different things like that. So these platform dashboards have the appearance of working or that they have everything figured out in quotations there.
But over time, these solutions have become less and less reliable, especially with signal loss impacting the last touch, for example, just adding on to what everyone said here is that we’re in this privacy by-default world, and we’re gonna continue to see more. We’re three months away from a couple of different state-level legislations coming into effect. So without user-level data, we’re seeing the expansion of channels. These new models have this renewed spotlight and in my mind, it makes sense to be executing on these models to be thinking about these, because it closes the gap, and how these things have changed, they become easier to execute. There are new ways to do it, which we’ll touch on more here. But it’s just a very interesting time, especially in the mobile user acquisition world to invest in these different models.
John Koetsier
Liz, I want to stick with you for a second because the history of MMM as Eran mentioned is Fortune 500 companies, they have huge spend, massive teams that can look at lots of different data and all this stuff. It’s evolved beyond that. But who is MMM really for? Is it small, medium or large companies?
Liz Emery
If this were multiple choice, I would select all of the above. Especially in the privacy-by-default landscape. How I look at it and how we look at it is that
If you’re a large company, you can invest in this in different ways, you can customize it in very detailed ways when you’re putting together MMMs but when you’re a smaller company, you can start small and still grow it.
There are ways to approach it. As I said, these models have changed and adapted to the ecosystem we’re in now than when MMM first came out and there was this gold standard but you needed X, Y, and Z to do it. It’s a lot more customizable right now.
John Koetsier
Jump in Igor. I know you want to.
Igor Skokan
I totally agree. MMM is changing in the capable hands of the modern data science teams and they are being transformed into this modern powerful tool and maybe concurring here like every generation discovers rock and roll. MMM is becoming a very popular tool. We see it works remarkably well, for all businesses. It’s very customizable and highly scalable. And there is progress to make it evermore open, transparent, and democratized. We have many examples of a lot of small companies who have adopted this as a tool and through the work we do on the open source project Robyn, we see a lot of this in smaller in-house teams adopting lessons and being able to generate meaningful insights in just a few days with relatively low pull.
John Koetsier
Shamanth sounds like time to get you involved here because you’re actually doing this. You’re using Robyn, you’re doing a bunch of interesting things. What are the particularly challenging elements of MMM, and incrementality for smaller teams?
Shamanth Rao
For smaller teams it’s that they typically don’t want to work with third-party tools, because that can be somewhat expensive. If they want to run an MMM in-house, getting it set up is, I think, a big lift. It’s not impossible, we’ve done it with zero programmers on our team, and we’ve worked with an external consultant who had a complete non-programmer team to get it set up. So it’s definitely possible, but it’s a bit of a heavy lift. Once you have it up and running, really make sure you’re able to troubleshoot and make sure the model reflects something that’s actually useful and helpful for your analysis. That’s the other big piece.
So you do need somebody that is dedicated to running these models and setting them up. That person doesn’t have to be a programmer.
John Koetsier
I’m taking that with a little grain of salt because I’ve heard you talk about using Python and you’re doing some sophisticated data science. I get it that somebody else has done the hard lifting, but you’re not super non-technical, either.
Liz, let’s turn to you. Same sort of question, what are the particularly difficult parts of MMM, and incrementality for smaller teams?
Liz Emery
I think the hardest thing for smaller teams is the data requirements. It’s not having the data, but is that data available? Is that data clean in a way that you can use it? Because MMM, is not what we like to call a push button solution. As much as we would love it to be a push-button solution. There’s a lot of manual work that goes into it.
So I would say that the data piece is probably the heavier lift that I see for smaller teams that we work with and even they’re working to set things up.
For incrementality, the biggest thing I say is that smaller teams, smaller clients, you can do this, like you can do this kind of stuff, like all day. I recommend that you do it if you have the opportunity to run incrementality whether that’s always on or one-off. The biggest thing is that this model doesn’t take into account certain external factors that MMM does. It’s really hard like the cause and effect, there are a lot of variables that aren’t always super clear. So with incrementality, I would say you want to use it in conjunction with other models. Remember, it can be directional because it’s hard to isolate everything with incrementality. So I would say just having the right lens when you run an incrementality as a smaller team is probably the biggest challenge because it’s a model you can run right now. It’s a model you can run tomorrow, they’re quick & executable. Just make sure that as a small team, you have the right lens on the data you’re receiving.
John Koetsier
It is super interesting Liz, I would just say one thing that I would add is that if you’re going to do some incrementality, you’re going to go hardcore with that, talk to your entire company, like your leadership structure and say, we are pausing this stuff here, be aware so that when we try this we know what’s gonna actually happen here. It may have a detrimental impact initially, we’re gonna get good data.
Eran, I saw you nodding while Liz was talking about data I had to come to you.
Eran Friedman
I have to echo everything Liz said. First is getting the data right to really cover everything from MMM. I know one of the important things in MMM is that you need to get all the factors basically included. So if you’re kind of only taking in some of the main channels but you’re actually running some inference from marketing at the same time and they’re not in the model, you’re gonna get some written numbers without realizing that there’s another factor just changing everything.
So it’s true to keep in mind. It’s important to have 100% of your spending covered, and your results as well, and then once you have that, I think part of the next challenge is understanding what kind of external factors can really affect things that are even beyond your control.
Igor mentioned that earlier, in a traditional sense, it was like, whether the weather has changed, for example, or holiday seasons, in the app ecosystem, those things can be very different.
Whether a particular app got featured, and that kind of shifted some of the effects of your spend, for example, or the joint CPMs in industry.
So due to market trends out there, that can also affect your numbers, and might be challenging to understand how they contribute to the model. So definitely a lot of things to keep in mind to get to the best MMM model there.
John Koetsier
I love the one about influencers. You paid a guy two months ago, the video came out on TikTok three months later, and then it went viral four months later. Igor, are there elements of MMM or incrementality that are easy to implement, even for the small team?
Igor Skokan
I’m just going to keep this brief, I would say just get started and organize your data. Like Eran and Liz said, with incrementality testing, just start getting into the habit of doing it. Once you have some data and once you have some tests, the tech exists. As Shamanth was saying, you need to be able to run some code, but folks with normal level of skills are able to run this kind of analysis, as long as you have the data and the tests ready at hand.
So I think it’s just one of those things to get started and collect the data, organize the data and get going.
John Koetsier
Shamanth, let’s bring you in, how do you just get started? What’s a good starting point for a small team?
Shamanth Rao
You can build basic spreadsheet-based models. That’s always the best starting point. We have a couple of YouTube videos on very basically walking people through how to build a spreadsheet-based model. Once you’re past that you can use open-source stuff like Robyn, which Igor was mentioning.
We use Robyn pretty much consistently across all of our bigger accounts. It’s really straightforward. Once you have it set up, you can have it running even with somebody that isn’t a hardcore programmer, really. I would say those would be the first couple of steps. And for people who kind of doubt it, we have YouTube videos, you can check out how to set up Robyn and how to set up a spreadsheet-based MMM as well.
John Koetsier
MMM in three easy steps. You’ve got great videos, I do recommend them.
Igor, let’s turn to you. We’ve talked about confounding variables, and the economy tanks. How do you separate out all these confounding variables that can change your marketing without not even talking about the spend that you’re doing?
Sometimes when you look at incrementality testing, you think, I gotta turn everything off and just do this one thing. Or you think about the complexities of all you’re saying is just, go heavy here, then go heavy there. That’s an easier approach.
Igor Skokan
You can do regions, depending on like, different games, and different portfolios, you can look at different ways to do this and try to introduce variability in your dataset.
John Koetsier
That’s an easy way to start. We’re almost ready to start talking about some of the best and worst-case scenarios that we can expect from these solutions.
Eran, let’s end this section by saying if somebody wants to start the MMM journey, what are the stakes for getting started? What do they need?
Eran Friedman
You want to start by making sure you have all the data. So you need to make sure how the aggregated spend is, make sure it covers everything that you can imagine and need to be accurate as possible with as much data as possible and enough history to rely on.
You want to obviously have your total performance. So usually in our eyes will be coming from cohort revenue, for example for users to actually understand the effect and any additional external factors, if you can think of any. Those three components are going to be input to the media mix modeling that you’re building.
With that, then there’s like a variety of open-source tools and frameworks that can help you come to get started. Some of these are actually for example, Robyn by Meta, which is a great framework, there are other alternatives as well. But basically, as everyone said here, start with something and you can definitely iterate moving forward. Obviously, for a Singular customer, this is something that we are also working on, and we’d be happy to explore this together.
John Koetsier
The second poll is going to be around incrementality. How do you account for incrementality?
Let’s pull those answers up. What have we got?
We don’t – 21%, guesstimation – 35%, full deal that is higher than I anticipated – 37% and 7% – you have some sort of SAAS tool and or tech partner.
So Shamanth, I want to start with you. We want to talk about, hey, there’s a lot of interest in the promise of privacy-safe aggregate measurement. MMM that we’ve been talking about. As you said before, there’s more interest in adoption. Why is that?
Shamanth Rao
I think
most of us mobile marketers grew up, if you will, with the D7 ROAS and act based on deterministic attribution. That’s been around for a decade. That’s what the reporting has always featured. Now if you’re like, we’re going to look at the share of budgets, or we’re going to run five different models, it’s not even like speaking that same language. Also, it just requires a different skill set.
As I said, we’re not programmers, but we had to muck around with code. We had to understand which models to use, we had to understand what kind of inputs go in and what kind of output gets out. So it’s just a different skill set altogether, which I think is why there’s been much more interest, the interest is just because the normal deterministic isn’t working. It’s such a huge mess. But there’s still some hesitation in adoption, just because it’s just a different ball game altogether.
John Koetsier
Okay, Liz, turning to you. In the best case scenario, you have all the data you need, you got most of your confounding variables sorted all that stuff. What can MMM deliver? What can you promise your CEO that you’re gonna get?
Liz Emery
Using MMM the best fit is that you can use your past marketing performance to influence your future ROI. So not only that, but you can do that by optimizing your allocation by channel by tactic and that’s not just the digital landscape that’s traditional as well. So it’s that full spectrum influence in terms of optimizing promotions pricing competitors spend, you just have so much insight at your disposal when you do your MMM as like a best case scenario to influence overall. At Tinuiti, we recommend MMM across the board. So it’s obviously a strong fit for mobile and mobile app clients. Obviously you always hear me talking about privacy, that’s where we’re headed, it just makes sense. Doesn’t matter small, medium or large.
John Koetsier
Shamanth, what level of accuracy can you attain?
Shamanth Rao
It’s very much a function of the volume of data. But I would say even for smaller clients we work with, we see R squared of like, 90-92%. That’s like the minimum we try to look at. So I would say that we do see very strong accuracy that informs a lot of our decisions.
John Koetsier
Igor, what can MMM deliver that you can’t get elsewhere?
Igor Skokan
Achieving parity with what currently the UAs see, is probably like the first step. MMM is somewhere in between your tools left and the last touch. But I think it has really started to become really interesting, once you start digging into the other things that MMM can give, competitive Intel, insights on game launches and cannibalization from within the portfolio, things that we couldn’t probably measure before. Also the macro-level impact.
First of all, you get what you used to get, but then as a plus, you start getting other things. But I have to say it here again and I think it’s going to be in one of those slides at the end – what is the key insights or key takeaways: MMM is not a silver bullet, there is no silver bullet. We believe that advertisers need a mix of complementary solutions. That is attribution, experimentation and modeling mixed with approaches and every company needs to figure out what works for them.
There is not even a single template or the right balance between these methodologies. Every company should figure out what works for them, for their budget, for their strategy and tactics. MMM is a powerful tool, but it’s not the only one and should be used in a complementary fashion. So that’s the best case. MMM can provide strategic and tactical support; it can have more channels with more sensitivity. It can be agile, you can run it three times per week, and you can try it out every day. Depends on how much you actually need it. Echoing what both Eran and Liz just said a minute ago. The right balance needs to be achieved bond for each individual company, in fact,
John Koetsier
Eran turning to you. Where does MMM not fit? What does it not do?
Eran Friedman
I think part of the challenge with MMM is with a very granular, very tactical kind of decision-making. So it’s difficult to get insights for example on the creative level. Of course, if you have a lot of data, if you’re running like creatives at skate or you’re having to quicken everything like running with tests, then you can optimize things. But generally speaking, usually, it would be easier to seek a solution from the other methods such as doing this attribution, versus MMM for the operational day stuff. That’s kind of the challenge.
I think it really ties in with what Igor said earlier. If you want to really get the most holistic solutions, you’re gonna need to use multiple methods to get the most accuracy and the most insight. Sometimes getting MMM to kind of understand your holistic understanding, but you need to kind of calibrate it and add additional insights from the bottoms-up approach. That’s what I’ve seen works the best.
John Koetsier
Liz, same question, where would you not use MMM?
Liz Emery
A couple of little things to add to it is that like branding and messaging impact aren’t included within the MMM world as it stands right now. The customer journey is a little bit murky in the MMM world. One of the biggest caveats is that it’s getting better, it’s progressing but it can be biased on offline channels. Because if you think about when it was built, what existed in that timeframe to where we are now, like I said, I’ve always liked MMMs as a gold standard. It used to be the gold standard of how we do things. It’s evolving. But there are still those biases that we’re tweaking that you have to pay attention to as you’re setting these things up. Like in our marketing science team with Tinuiti when we build these things, we’re making sure to account for certain biases as we set up the factors that go into it.
John Koetsier
Eran, I want to turn you, Singular has recently introduced this concept of hybrid measurement. What is that? And how does MMM and incrementality fit into that?
Eran Friedman
Gadi, our CEO recently published a blog post on our view on what we call the hybrid measurement approach. The idea, which really connected with everything is that with all the different methods out there, if you tried to zoom out, there’s SKad network, probabilistic, device level data, incrementality testing and MMM. Again, there isn’t a single framework, there isn’t a silver bullet that you can really run your entire corporate marketing operation on.
So as we look into the future, instead of relying on a single method to base all your work on, in our view, the future is gonna have to require multiple methodologies. And it’s really the combination of these solutions that are going to provide the most actionable and insightful measurements. So think about the data and signals available from all these networks for all these measurement methods, from a unified infrastructure that can really enable you, basically the best of our things like relying on kinds of the bottoms-up methods, like the deterministic attribution or SKAd network or anything, because you need that for their operation to work and to category things. You need to rely on entirely testing and that can be your MMM framework on top of that.
The combination of these is gonna provide the best insights moving forward, and that’s kind of where we see the industry is headed.
John Koetsier
The third poll is going to be about what you’re planning to do in the next 12 to 24 months. Question is – will you be doing MMM and or incrementality in the next 12 to 24 months?
Wow. 97% of you are doing it & planning it.
Okay, now we’re moving into real life. Shamanth can you share a real-world example that you used MMM and what you learned from it.
Shamanth Rao
This is for the subscription app we work with. We’ve had some fairly significant spending, and we ran the MMM to understand what the ideal share of spend should be, and what the true value of trials was. We use trials just because that’s what happens in the first 24 hours. It’s a much better set of dependent variables than let’s just say revenues or purchases which can be influenced by renewals. So we started with a trial.
What we realized was
For something like Google, where you’re just basically trusting Google-reported numbers on iOS, numbers look wildly optimistic and numbers look crazy because of Firebase, they’re the referee and the player. Obviously, numbers look very, very good. It turned out, we were spending way more than we should have been. When we did cut the budget, we just saw no change in the overall trials baseline. We just cut the budget by 66% because the analysis said Google wasn’t driving anything incremental. Nothing moved and that was just a huge win. That’s one that comes to mind.
John Koetsier
What a win. Absolutely. You’re spending potentially millions of dollars a month on a place that’s not actually driving incremental activity and you learned.
Liz, a real-world example.
Liz Emery
In my role, I work across mobile apps and I also work across a lot of cross-channel clients. We had one that was in the FinTech space. As all the different changes happen with signal loss, especially in the mobile world, all of a sudden, our price points and how things looked, didn’t look so great, just because of all the new limitations and changes. We were able to leverage and this goes back to the conversation earlier about those hybrid solutions, we were able to actually leverage a combination of an MMM that we had built with them out of Tinuiti, some incrementality tested and projected models to close that gap.
As we implemented these models, we were able to change exactly where they were executing in terms of their budgets to get a much higher return, and we were able to actually prove which channels work. We were also able to take into account environmental factors that set up our client to go to their board and have this conversation in a much stronger way, because not only was it COVID, recession and unemployment numbers and all these different things at the time period, but on top of that, there was a signal loss.
So we were able to use everything we had at our disposal by doing that unified measurement, or combination of solutions to close that gap. It was a major success for that client internally with their leadership. Also for us at Tinuiti to prove like, hey, here is the value when you use the right combination of measurement solutions for you. Because there is no one size fits all, you need this little bit of everything.
John Koetsier
Impressive! Also Liz, a role for first-party data in this?
Liz Emery
There’s just a renewed spotlight on the power of first-party data as signal loss continues to increase. But data has always been the strongest signal in my mind or more than the most important signal. And basically, data is the foundation. Marketing has really shifted in the last 10 years. 10 years ago, we relied on third-party signals and now we’re shifting back. So it’s just that renewed spotlight, we’re moving back to a time where it’s a stronger foundation than we’ve been walking on for 10 years now.
John Koetsier
Eran, that’s gotta be huge because if you have an SDK in somebody’s app, and you can see actual use, actual purchase, actual signups, all that stuff that they’ve instrumented, then you know, okay, stuff is happening out here in the world. Here’s what’s actually happening inside, correct?
Eran Friedman
Exactly. First-party data is critical to ensure the accuracy of your model. We can use that for so many things, including drilling for tests for ground control and making sure that your home is as accurate as possible. First-party data is gold. The more you have it, the better it is for everything, basically.
John Koetsier
Igor, one of the challenges with MMM is you’ve got confounding variables that you have to adjust for. How do you ensure that when you’re adjusting for some of these you’re just artificially tweaking your model to fit reality
Igor Skokan
Our models have been traditionally built by an analyst and by hand, very crafty people have been spending days and maybe even weeks, trying to test different variables and combinations of the different transformations and so on.
We see that the data-driven or semi-automated from ultimate MMM can help reduce this kind of decision complexity and can actually minimize the human bias in certain instances. We try to run all kinds of combinations of the variables and the transformations to find the right you know, sort of combination of it.
We’re not fully there yet so that it could be fully decoupled from the human’s skill set and try to look at the entire system at least for now, and find that sort of balance between it. We massively minimize it from the craft-only black box type solution to a much more open, transparent, and customized, semi-automated experiment, calibrated system. But the real answer to this question, in my opinion, is whether you don’t do it in-house or through a partner, it is really important to put actionability at the core. So request forecasting and simulations to be done to verify the accuracy of models over time, because only that’s how you’re going to find that it actually works.
Often we get asked, how can we show that the modeling itself is worth the investment, for example. We say that the only words are if you take positive actions of the results of the modeling, and that means that when you do it in-house or through a partner, we have to be clear about why we’re modeling and then put actionability at the core. And is it a planning tool to help you budget sessions? Is it the campaign measurement tool? Do you want to understand the macro factors? Is it a defense mechanism for your finance team? I know it’s going to be all sorts of things but making sure that we’ve seen the very best operations when actionability is at the core. Frequent simulations are made to verify accuracy over time.
John Koetsier
Everybody can make the past make sense. If you can make predictions about the future that have truthfulness and accuracy and you can test that and see that boom, then you know, you’ve done something impressive.
We’re nearing the end. But we have a couple of important things left, we’re going to ask for predictions on MMM from all panelists, we’ve got one more poll, we’ve got a key takeaway from each marketer and most importantly, we’re going to answer some of your questions.
The question is this, when will MMM be a core part of the majority of digital marketers’ toolkits? Liz?
Liz Emery
We’re seeing it now. Even if it’s just a conversation on budgets and planning inputs, we expect by the end of 2023, for it to be a core piece.
John Koetsier
Igor?
Igor Skokan
I think we have seen significant evolution over the last 12 to 18 months specifically, we foresee the same going forward. So in the next 12 to 18 months, I think this will become a more firm core part of digital marketer solutions.
John Koetsier
Excellent. Shamanth?
Shamanth Rao
Definitely see significant adoption into the new year. A lot of folks are already asking us and actually using MMM-based insights in their day-to-day report. As Igor suggested, it’s really one part of the puzzle in which we do look at channels, reported numbers, and complement it with MMM reported numbers, which are already a part of our reporting cadence. And I definitely see that becoming increasingly the case as we go forward.
John Koetsier
Excellent. Eran?
Eran Friedman
So I think it’s hard for me to imagine that the majority of marketers would move towards MMM in 2023. But definitely just based on the poll results received that there is huge interest there and I definitely predict that we are going to see a massive shift toward it in 2023 for sure.
John Koetsier
Wonderful. Thank you.
For you, Igor, what is your key takeaway on MMM and incrementality?
Igor Skokan
I think it’s really about the rapidly changing environment, we see advertisers leveraging many different tools, with many different levels of aggregation speed, biases, data holes, and calibrating them against each other. This alone requires a sort of agile approach and a diversity of analytical skills. But the benefits are really to performance improvement across the whole. So use what you have every advantage to find the balance between these methodologies to be best positioned for success.
John Koetsier
Excellent. Liz, your key takeaway?
Liz Emery
What I remember writing was that there’s no one size fits all solution right now. In the privacy-by-default landscape that we’re in, you’re going to need a combination of durable solutions. And like I said, you don’t have to break the bank doing this. I think that’s one of the biggest misconceptions. This is going to cost me so much money, and I need to stick in my lanes. There are ways to do this but still, have the right combination of solutions for your size of business.
John Koetsier
Wonderful. Shamanth?
Shamanth Rao
Try to understand what the true value of your marketing spending is because it’s definitely not what the dashboards tell you. Definitely not after ATT.
John Koetsier
I love the second part of yours, which is to learn to let go of the certainty of deterministic measurement. It is interesting, I was talking to a marketer about that a couple of weeks ago, and they’re saying this kind of freeing. Eran, your key takeaway?
Eran Friedman
Connecting with what everyone said so far. So it’s not gonna be like a single measurement method here, it’s not going to be a method that’s gonna save everything, you’re really gonna have to use a combination of signals, kind of our approach of the hybrid measurement that we strongly believe in and think about how you can get more and more and trying to get to have that totalistic decision from it.
John Koetsier
Excellent. Thank you so much.
Now we turn to the questions from the audience.
What techniques are available to validate an MMM mode? Igor answered that he said, make predictions if they come true, you validated it, keep making predictions and see how it goes right?
How can you incorporate in-memory incrementality in daily optimization of campaigns? Eran kind of answered and said, that’s not exactly what it’s for, try and stick to staying away from that.
I will ask this one. It’s for Liz, what legislation were you referring to? You mentioned, several states were bringing out privacy focus legislation briefly, what were you talking about there?
Liz Emery
In January of next year, you’re gonna have at least four or five state-level legislation coming into effect and being enforced. An easy example to find is the CCPA. That’s in California.
How I look at things is that it’s basically bringing us closer to GDPR in Europe, these legislations are cracking down, especially on the data front. So I would say paying attention to those and making sure you’re compliant is something you should be doing now if you’re not because all this feeds your models. So locking those things down. There are agencies and companies that can help you with that. The other one I would say is that thinking about I always say this, it’s a really good comparison is that Europe is very much opt-in you have to opt into being tracked, whereas the US as we call it, opt-out, so it’s a very unique landscape, but I would say pay attention to state-level Colorado, Virginia, California, three off the top of my head.
John Koetsier
Oh man, if it’s not complicated enough, now you have to worry about what state somebody comes from to be compliant. Excellent, great.
Eran, turning to a question for you: how can my MMP help with MMM?
Eran Friedman
We’re already providing the unified infrastructure. You have the data so you’re getting part of the spend across all your channels you’re getting the results that you’re having, the revenue or the performance KPIs that you’re looking for, and want to see are the market trends, any kind of external factors that might affect your numbers?
So basically, as we said, we can read the entire stack from providing the data, to providing even the insights based on the model results. We’re happy to talk more about that if you’re interested to learn more about our approach.
John Koetsier
Great answer and greatly brief. That’s wonderful on both counts.
Sherry Lin is asking: Does MMM work during extraordinary circumstances, like a pandemic? We’ll give that to Igor.
Igor Skokan
Last summer, we actually published a blog post and an article about how modeling can be set up for extraordinary events. In the forecast things, no one could have forecast what is going to happen, but you can definitely account for it in the model that you are building. So there are various ways various data sources, and various techniques can be deployed to account for the impact of the pandemic, itself. I’m going to send you the link, maybe you can pass it on to Sherry.
John Koetsier
We will add that to the blog post, and we’ll send that right to Sherry. If you find the link, you can put it in chat here as well.
Shamanth, can MMM be used for media testing insights so can you make predictions or assumptions and then test them with MMM?
Shamanth Rao
Yes. You had to make the assumption, of course, that your historical performance is a good predictor of your future performance. Like in the Google example that I gave, we were like, according to the model, Google’s just not adding any trials and we turned it off. And it was Astrid. So yes, assuming your future is similar to your past – Yes.
John Koetsier
Excellent. MMM can be used for retail and brick-and-mortar sales as well. I’m guessing that answer is yes. Liz, am I accurate?
Liz Emery
Yes. One, I would say I look at the factors of MMM as three buckets: marketing factors like your media and your non-media, non-marketing factors like economics, competitor, performance, weather, holidays, what’s going on in the world. And then the third bucket is called the error bucket because no model is perfect. So there’s always a margin for error. But yes, I am 100% with that question.
John Koetsier
If you’re looking at spending by the network as a factor. How do you deal with the ebb and flow of organic users for your app? I’m gonna send that one to Shamanth.
Shamanth Rao
You can factor in organics as an independent variable in your models, and at least Robyn which is what we use, so you kind of should absolutely use non-paid variables.
John Koetsier
Excellent. Well, I think we’re going to end there because frankly, we’ve run out of time, but I have to say, first of all, thank you, attendees, for joining. I really do appreciate it. Thank you for your questions, ones we didn’t answer will be answered on the Singular blog. I have to thank each panelist. This has been amazing. It’s been incredible.
Thank you all. Have a wonderful day and see you next time.
A REQUEST BEFORE YOU GO
I have a very important favor to ask, which as those of you who know me know I don’t do often. If you get any pleasure or inspiration from this episode, could you PLEASE leave a review on your favorite podcasting platform – be it iTunes, Overcast, Spotify or wherever you get your podcast fix. This podcast is very much a labor of love – and each episode takes many many hours to put together. When you write a review, it will not only be a great deal of encouragement to us, but it will also support getting the word out about the Mobile User Acquisition Show.
Constructive criticism and suggestions for improvement are welcome, whether on podcasting platforms – or by email to shamanth at rocketshiphq.com. We read all reviews & I want to make this podcast better.
Thank you – and I look forward to seeing you with the next episode!