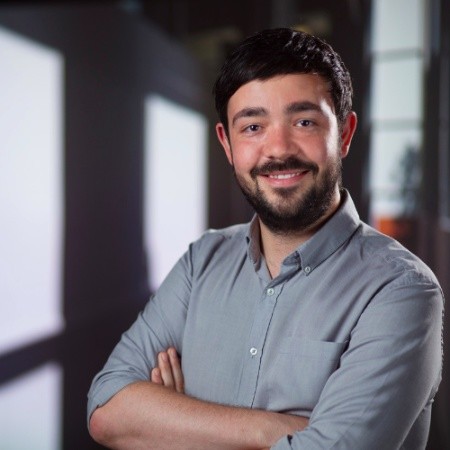
Our guest today is Michael Taylor.
Mike is the co-founder of Vexpower, a platform that hosts simulator-based courses for data-driven marketers who want to be more technical. Prior to this, Mike also co-founded and led the team at Ladder.io.
This episode has 2 parts, this audio interview, which is followed by a video walkthrough on Youtube. The audio interview you’re listening to provides background and context, and in the video Mike takes you through a screen-share of LightweightMMM, how it’s configured, and shows how to use and run it.
You can check out the video here: https://youtu.be/oRYN0V6sPlM
I’m excited to present this very inspiring and fascinating episode today.
**
Notes:
Mobile Growth Lab
In a few days, we will open the doors to the third edition of our workshop series The Mobile Growth Lab, which will help you break the shackles of ATT’s measurement and performance losses and win in a post-IDFA world.Sign up for the waitlist here: https://mobilegrowthlab.com/
We have a growing community of mobile marketers!
The Mobile Growth Lab Slack: A community that was a part of our workshop series – The Mobile Growth Lab, is now open to the general public. Join over 200 mobile marketers to discuss challenges and share your expertise. More details are available here: https://mobileuseracquisitionshow.com/slack/
If you’re ready to join the growing community, fill this form: https://forms.gle/cRCYM4gT1tdXgg6u5


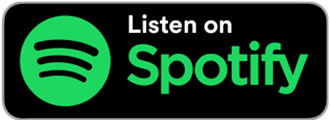

ABOUT MICHAEL: LinkedIn | Google’s LightweightMMM Course | Vexpower
ABOUT ROCKETSHIP HQ: Website | LinkedIn | Twitter | YouTube
KEY HIGHLIGHTS
🌰 How is Facebook’s Robyn different from traditional MMMs
🧃 Why Facebook’s Robyn seems to more acceptable than traditional MMMs
🤿 Robyn’s open source makes it an easier model to learn
🕹 The entry of Google’s LightweightMMM
🛠 Understanding the Bayesian model, and how it’s different.
👾 A Bayesian model is more descriptive when compared to an MMM on Excel
KEY QUOTES
How Facebook got into the MMM business
There’s a lot of room for interpretation with MMM. You can basically hack your way to whatever result that you want. And they’re all hired by traditional brand marketers who are very keen on hearing the message that TV ads are working. They don’t really care that much about digital. So I think that Facebook saw and asked them this specifically. From my take on the market, Facebook saw this and said, “Hey, if we know that Facebook Ads work, and even post iOS 14, we know that they’ll continue to work. How do we get people to understand that? And how do we make sure that we take the bias out of doing the MMM process?”
The difference between an excel model & Robyn
So if you put a variable into an Excel model, or into a normal MMM model, and that model isn’t statistically significant, normally, the analyst would then choose whether to leave it in or remove it or change it in some way. With Robyn, it just automatically shrinks to zero in terms of effect. So if you have a channel that’s not statistically significant, at least if everything’s kind of working, okay, then it will remove it from the model automatically for you.
The difference between a Bayesian model and Robyn
The reason why Facebook with Robyn is building 10,000 models, it’s because it’s trying to sink the ship. So it’s trying to find the right models out of the 1000s and 1000s of models that are wrong. A Bayesian does that in a different way. Actually, the way it does it is, it simulates the physics of a ball rolling down a hill. So imagine you have a chart and you have a correlation. So you have a line chart and two variables are correlated. That’d be like the simplest model, when it finds the edge of the line, it will roll balls down the hill, to find where the line is drawn.
There’s more information available with Bayesian models
If you’ve heard Bayesian, you’ve heard someone talk about priors. A really simple example is whenever I run a marketing mix model in Excel, quite often it will say, Facebook ads drove negative revenue and the coefficient will be negative. And you’re like, that’s not possible, my campaign wasn’t that bad that it decreased sales. So with Bayesian, you can say, I know that this campaign didn’t decrease sales, but I also know that it probably didn’t drive 100% of my sales. So I know that it’s somewhere in between.
FULL TRANSCRIPT BELOWShamanth
I’m very excited to welcome Mike Taylor, the founder of Vexpower to the Mobile User Acquisition Show. Mike, welcome to the show.
Mike Taylor
Nice to be here.
Shamanth
Excited to have you, Mike. For folks listening, this is a slightly different format from our traditional episodes. Mike is going to take us through a walkthrough, which is on YouTube, and we will link to that. This interview is to provide context and background before diving into the YouTube video to understand exactly what we’re talking about.
We’re going to talk about solving iOS measurement challenges. One of the things that’s been top of mind for us, and certainly something we’ve learned from you, Mike, has been MMMs or media mix models.
Let’s start by having you tell us about Facebook’s Robyn, how that works and how it’s different from traditional MMMs.
Mike Taylor
MMMs have been around since the 1960s. It’s not a new technique. And usually they would do this on a computer. Now we could do it on Excel, or a lot of people will use custom R scripts. R is a statistical programming language. So what’s different about what Facebook has done as they entered the market is that they realized, I think that if you just leave it to the analysts, you leave it to the people who are doing MMM, they can be biased.
A lot of these traditional MMM consultants, agencies, freelancers and specialists: they are very good at what they do. But
There’s a lot of room for interpretation with MMM. You can basically hack your way to whatever result that you want. And they’re all hired by traditional brand marketers who are very keen on hearing the message that TV ads are working. They don’t really care that much about digital. So I think that Facebook saw and asked them this specifically. From my take on the market, Facebook saw this and said, “Hey, if we know that Facebook Ads work, and even post iOS 14, we know that they’ll continue to work. How do we get people to understand that? And how do we make sure that we take the bias out of doing the MMM process?”
So that’s really the headline for them. Removing human bias and automating it for really anyone to try this type of analysis. So they made a couple of stylistic choices because of that: they used retrogression, which is a machine learning algorithm, which is a bit more advanced than what one would normally do in Excel with regular linear regression. It’s still related, but what it does is, it automatically does feature selection for you.
So if you put a variable into an Excel model, or into a normal MMM model, and that model isn’t statistically significant, normally, the analyst would then choose whether to leave it in or remove it or change it in some way.
With Robyn, it just automatically shrinks to zero in terms of effect. So if you have a channel that’s not statistically significant, at least if everything’s kind of working, okay, then it will remove it from the model automatically for you.
That’s one stylistic choice they made. The other thing they did was, because we don’t need a computer the size of a room, my laptop can build 10,000 models, and take a couple of hours to analyze this. The computing power is much better now and much cheaper now than it used to be. Taking advantage of that, they’re using Nevergrad, which is an evolutionary algorithm that basically starts building models and it uses natural selection between these models to almost grow towards the right solution. So they’re always trying to make the model more accurate, and trying to make the model more plausible, meaning, it’s not recommending something that’s really wild compared to what you’re currently spending. Therefore, it’s doing a lot of that selection for you. Rather than having an analyst build 100 models and then choosing the best one, it’s building 10,000 models for you automatically and then giving you maybe 100 of the best to choose from.
Shamanth
Yeah, and it’ll also tell you what the accuracy of those models are, when it comes back historical forecast versus actuals. In my limited experience, it is one of the things that we have found valuable.
Mike Taylor
Exactly. But I think actually, the biggest thing that they’ve done is just lend credibility to this as an approach. I was investigating MMMs already, because I had seen there were issues with ad blockers with some of my clients. I was working with a lot of developer tools and things. 30-40% of the people visiting the website had ad blockers on so you can’t actually attribute that traffic. And then you have GDPR on top and ITP, intelligent tracking prevention was something that came out on Safari a while back where all the cookies get deleted after seven days. So, last click was already dead when iOS 14 came in. I was already exploring it.
But then when Facebook came out with this model, it was them kind of reaching down from the heavens and saying, “Okay, I anointed this technique as a plausible thing to use.” And I think that’s what got a lot of people interested, because before that point, it wasn’t on a lot of people’s radar. The people that had tried it, didn’t quite understand it, or hadn’t really investigated it, didn’t have a statistics background. So I think now, it’s created a lot of interest.
Shamanth
I agree. Part of what I think made it more widely used, it’s just that it’s much more usable than traditional models. Folks on our team are able to use it. It’s very powerful and it’s been democratized.
Mike Taylor
Exactly. It’s also just open as well. When I was trying to learn MMM, it was very hard to find anyone who would tell me anything. Because what they see is the secret sauce. I had a few really generous people. There is Dr. Grace Kite from Magic Numbers. She was really great. Took me through a lot of training that they give to new staff. I was also talking to a few other people I was working with on a freelance basis. And they were showing me what they did. I was just trying to learn from what they did. It was really hard actually, to even get any information.
I was reading all these obscure statistics papers. So it was tough. And then obviously, when Robyn came out, it was completely open source. So you could actually poke into the code and see what was happening, why they were doing certain things. And they actually provided a lot of documentation, the team has been really good at answering queries as well. So I think that’s another thing that brought us like the Silicon Valley openness to what can be quite a shady industry.
Shamanth
Just to switch gears a bit, and this is what your video is going to be about: tee us up and tell us what Google is doing. And how is that different from what Facebook’s doing?
Mike Taylor
Google has entered the arena now. And I was really waiting for that. Just to kind of caveat it, It is an unofficial library, LightweightMMM. Basically a side project of one of their engineers. But it’s a great entry. The thing that I was really excited about was when I was trying to learn, even pre-Robyn, I found all these kinds of papers from Google, about how to do Bayesian MMM, and they were too complicated for me to understand. They wrote six of those papers in 2017. And it was all similar people at Google writing them. So they’re actually ahead of Facebook on MMM, but they just never released a tool for people to use. And I don’t know why, now that this has come out. It’s actually referencing those papers, it’s actually built on those same principles. So the Bayesian chain algorithm they use is really different. In practice and in theory, to what Facebook is doing with Robyn, Facebook is much closer to a traditional MMM. It’s some cool machine learning stuff sprinkled on top. I think Bayesian is fundamentally different in terms of what it can do, and how it does it.
Shamanth
Help us understand how so. I have some understanding what Bayesian models are. I am not so sure everybody listening will. So help us understand what Bayesian paradigms or Bayesian models are, and how that applies to what you’re talking about.
Mike Taylor
I had to read a bunch of statistics books and talk to smart people. I really learned Bayesian from Michael Kaminsky from Recast. He has been my mentor, and he built the data science team at Harry’s. They obviously use this in house and then now he has a marketing mix modeling tool that he’s using. He basically walked me through this. The example that really helped me understand Bayesian was if you think about what you’re trying to do when you’re building a model. It’s kind of like the game Battleship.
Basically, you have a grid, you have maybe a hundred squares in the grid and you can’t see the opponent’s grid. But you place your chips on the grid, and you take turns firing. So I’ll say, I want to fire on grid, d3. And if I missed you tell me I miss, if I hit you tell me I hit. The way you win the game is like, when you get a hit, you fire lots of times around that place where you got the hit, and then hopefully, you sink the ship. But obviously, you can’t see the ship yourself.
I think the model is a lot like that.
The reason why Facebook with Robyn is building 10,000 models, it’s because it’s trying to sink the ship. So it’s trying to find the right models out of the 1000s and 1000s of models that are wrong. A Bayesian does that in a different way. Actually, the way it does it is, it simulates the physics of a ball rolling down a hill. So imagine you have a chart and you have a correlation. So you have a line chart and two variables are correlated. That’d be like the simplest model, when it finds the edge of the line, it will roll balls down the hill, to find where the line is drawn.
That’s my explanation of it and it does it super efficiently. I think one thing that’s really different about the way that they do it is all of the parameters of the model being estimated by this. Whereas with Facebook, it’s two different algorithms. It’s the actual model that has been built by retrogression and then saturation. So what if the channels get inefficient when you spend more money: that’s been modeled by Nevergrad, that evolutionary algorithm. So the difference of Bayesian is, all of that is kind of self contained in the model. It’s all being estimated together at the same time.
Shamanth
That definitely makes a lot of sense. Again, I’m not a programmer, but at a high level, having used and seen Robyn, and certainly, as somebody that’s excited to dive into Google’s LightweightMMM, yeah this is definitely exciting and interesting.
Mike Taylor
I think all of that is really esoteric, I don’t know which one’s more efficient. I can’t work out the math myself. But, what it lets you do, which is different, is that you can tell the algorithm where to roll the balls. So if you know that there’s gold buried in the hill over there, you can say, throw all the balls on that hill.
Shamanth
What is an example of that?
Mike Taylor
They call that priors.
If you’ve heard Bayesian, you’ve heard someone talk about priors. A really simple example is whenever I run a marketing mix model in Excel, quite often it will say, Facebook ads drove negative revenue and the coefficient will be negative. And you’re like, that’s not possible, my campaign wasn’t that bad that it decreased sales. So with Bayesian, you can say, I know that this campaign didn’t decrease sales, but I also know that it probably didn’t drive 100% of my sales. So I know that it’s somewhere in between.
That’s a practical example but you can get much more complex. Like you can say, I know that there’s diminishing returns in this channel, or, I know that with TV, there’s going to be a lag effect. So you can be a lot more descriptive to the model and incorporate some of your domain knowledge into the model, so that it leads to much more plausible results.
Shamanth
That makes a lot of sense.
This is perhaps a good place for us to let folks jump off into your video on YouTube, so they can find out more about LightweightMMM.
This is a good place for us to wrap up the audio, but before we do that, Mike, can you tell folks how they can find out more about you and everything you do.
Mike Taylor
I’m the founder of Vexpower, it’s a simulator based training. So essentially, we make up fake companies and give you tasks to do for their company. So it’s like on the job training. Bayesian MMM is one of the things that we teach. We have a course on how to get up and running with LightweightMMM. We have another course on how to do that same thing with Robyn, and lots of other courses. So definitely check that out if you want to learn how to do this yourself.
Shamanth
Wonderful. And we’ll link to that in the show notes as well. But for now, this is a good place for us to wrap. Thank you so much, Mike, for taking the time to talk to us.
You can check out the video here: https://youtu.be/oRYN0V6sPlM
A REQUEST BEFORE YOU GO
I have a very important favor to ask, which as those of you who know me know I don’t do often. If you get any pleasure or inspiration from this episode, could you PLEASE leave a review on your favorite podcasting platform – be it iTunes, Overcast, Spotify, Google Podcasts or wherever you get your podcast fix. This podcast is very much a labor of love – and each episode takes many many hours to put together. When you write a review, it will not only be a great deal of encouragement to us, but it will also support getting the word out about the Mobile User Acquisition Show.
Constructive criticism and suggestions for improvement are welcome, whether on podcasting platforms – or by email to shamanth at rocketshiphq.com. We read all reviews & I want to make this podcast better.
Thank you – and I look forward to seeing you with the next episode!