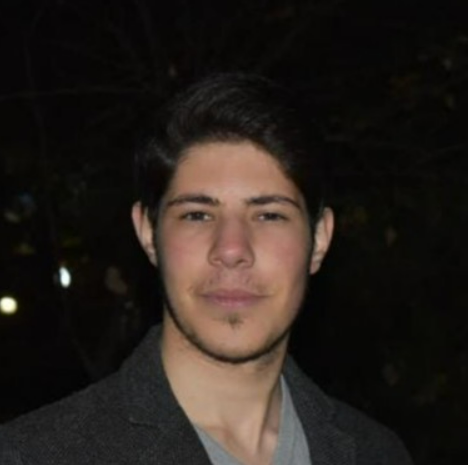
Our guest today is Sami Biçer, Growth Manager at MagicLab.
In this episode, we’ll embark on a journey into the world of ad monetization, focusing specifically on the topic of latency. We dissect the intricacies that govern the time it takes for ads to load, unraveling the critical balance between user experience and revenue generation.


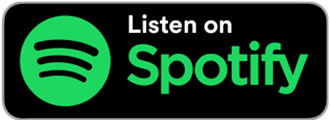

About Sami: LinkedIn | MagicLab
ABOUT ROCKETSHIP HQ: Website | LinkedIn | Twitter | YouTube
KEY HIGHLIGHTS
🎯 Precise Event Measurement: The Bedrock of Analysis
📊 Defining Key Data Points
⚖️ Balancing Acts: eCPM vs. Impressions, More vs. Less Placements
🔍 Finding the Optimal Through A/B Testing
📈 The Results
Latency in ad monetization describes the time it takes to load a single ad. In a particular waterfall, it is measured by the time it takes between an ad request and the time when it is ready to be shown to the user, which is when it is loaded.
Checking the latency is not just about the incremental revenue; it is also about the overall user experience. For example, I think one of the main reasons for the low IMPDAU(Impressions Per Daily Active User) on the rewarded ads is the failure to watch the ad, which is caused by long load times.
You’ll find latency etched in the analytics dashboards, and you’ll see custom metrics integrated by publishers in their apps to measure it. But when it comes to translating these numbers into actionable insights that can genuinely make a difference in your bottom line, that’s where the complexity unfolds.
In an ideal world, we could envision a scenario where all users load ads precisely at the same time, and these ads seamlessly align with the predetermined checkpoints set by the app publisher. Unfortunately, the reality is a far cry from this utopian vision. Latency is influenced by a myriad of factors, each contributing its own unique complexity. These factors include the geographical location of the user, the number of ad placements within the waterfalls, the networks these placements are affiliated with, the specific operating system in use, the degree to which the game is optimized, and so on.
Accounting for these factors in a meaningful way can seem like an insurmountable challenge.
In light of these complexities, data analysis allows us to make sense of the chaos and derive valuable insights from this web of attributes. However, achieving meaningful analysis hinges on a few critical factors.
First and foremost, the event measurement methods within the app must be precise. These measurements serve as the building blocks for all subsequent analyses. Without accurate data at this foundational level, the entire analysis becomes compromised.
Equally important is the choice of analytical tools. In our experience at MagicLab, we’ve found that leveraging the capabilities of Google Analytics and BigQuery has proven to be highly effective in dissecting and understanding the data. These tools offer the necessary depth and versatility required to unlock the insights hidden within the numbers.
To navigate the complex landscape of latency, it’s crucial to define specific data points and events that warrant tracking. These data points serve as signposts along the user’s journey within the app. Some of the key timestamps we monitor include:
· The initiation of ad requests from the mediation platform.
· The readiness of an ad for display.
· The user’s arrival at an ad checkpoint.
· Whether the user successfully watches the ad or fails the attempt.
This granular data forms the foundation upon which we build our understanding of latency. It allows us to examine the time intervals between critical events:
· The time elapsed between a request for an ad and its load in successful displays.
· The time elapsed between a request for an ad and its load in failed displays.
We observed that in an average waterfall, in terms of the number of placements, the mean time in the first and the second metrics on the interstitial side was around 10 seconds and 50 seconds respectively.
Through our analysis, we saw that in the majority of cases, missed opportunities in interstitial ad checkpoints were primarily attributed to the failure to load the ad itself. Conversely, nearly all loaded ads before the ad checkpoints were subsequently shown to users, providing a clear delineation between these two distributions.
When these two distinct metrics are combined, a bell curve emerges. The mean of this curve is essentially shaped around the mean ad load times of the users. The time between consecutive ad checkpoints is then compared to this value.
One of the critical insights we gleaned from this analysis is the delicate balancing act between eCPM and impressions. It’s a pivotal decision point when determining the number of ad placements within a particular waterfall.
Opting for a high number of placements in the waterfall enables targeting users within the left-hand side of the mean of the curve. Increased number of placements often yield higher eCPMs, at the expense of missed impressions from the users of the right-hand side of the curve.
Conversely, choosing to target users within the right-hand side of the curve yields lower missed opportunity rates and a higher number of impressions. However, this strategy often comes at the cost of a slight drop in eCPM.
To strike a near-optimal balance, we embraced the power of A/B testing. We initiated the process by significantly reducing the number of placements in the first batch and incrementally increased them with each subsequent run. The objective was to observe when the first negative impact surfaced.
It’s important to note that the near-optimal number of placements isn’t a universal constant. It varies based on factors such as the user’s country, the specific ad unit in question, and the characteristics of the waterfall itself. Additionally, seasonality exerts its influence through eCPM fluctuations, causing the optimal point to shift slightly across all waterfalls.
With all these variables at play, one might be inclined to feel overwhelmed. However, as we discovered, once you’ve established the right testing structure and embraced the power of data-driven decision-making, the results can be truly remarkable.
Across our diverse portfolio of apps, we witnessed positive results. In particular, we noted an impressive approximate 5 to 20% ARPDAU increase across Interstitial, Rewarded, and Banner ad units.
In conclusion, managing latency in your waterfalls can be quite complicated theoretically, but with the correct set of rules and testing structure, the incremental revenue it generates would be quite remarkable.
A REQUEST BEFORE YOU GO
I have a very important favor to ask, which as those of you who know me know I don’t do often. If you get any pleasure or inspiration from this episode, could you PLEASE leave a review on your favorite podcasting platform – be it iTunes, Overcast, Spotify, or wherever you get your podcast fix. This podcast is very much a labor of love – and each episode takes many many hours to put together. When you write a review, it will not only be a great deal of encouragement to us, but it will also support getting the word out about the Mobile User Acquisition Show.
Constructive criticism and suggestions for improvement are welcome, whether on podcasting platforms – or by email to shamanth@rocketshiphq.com. We read all the reviews & I want to make this podcast better.
Thank you – and I look forward to seeing you with the next episode!