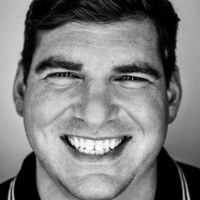
This episode features Eric Seufert, founder at Heracles, founder at QuantMar, and founder at MobileDevMemo.com . Eric is perhaps one of the smartest people in mobile user acquisition today – in this episode Eric walks us through how to model out an app’s DAU.
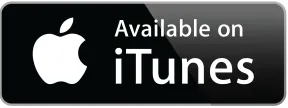
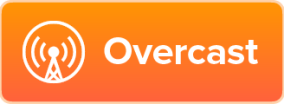
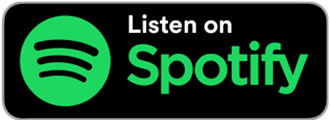

ABOUT ERIC: LinkedIn | Twitter | Heracles Media | MobileDevMemo | QuantMar
High growth, low growth, no growth: systematic growth with DAU replacement
ABOUT ROCKETSHIP HQ: Website | How Things Grow | LinkedIn | Twitter
KEY HIGHLIGHTS
🔒Why a systematic modeling of growth is crucial to an app.
🏈The key functions of a growth team.
🔌What inputs Eric recommends for a systematic modeling of growth
👉🏽How to think about the DAU gap to be closed — and the UA effort required to close it
🤔How granular or high-level a model should be — how Eric recommends thinking about this
🔎Applying retention profiles to different user groups to track growth
📍How to identify shortfalls to hit certain DAU or revenue targets
KEY QUOTES
The reason to have a quantitative system for app growth
If you think about a growth team that isn’t managing growth to some standard or some projection or some business requirement, then they’re just doing stuff right there – just bringing new users into the app or to the product – but without any kind of eye towards a business objective.
The core objectives of an mobile growth team
So, if you think about what a growth team does is really two things. One, it exploits some set of channels to the new users and cool products, and it optimizes those – that’s the ongoing thing. And then, the other thing is, it builds towards some models or some expectations of revenue growth.
Using user behaviour to predict revenue
When you break that user base out into atomic units, which are users, you can group them in ways that help you understand how those groups are going to make money, and how the revenue is going to change over time. Also, how those groups are going to decay and shrink over time just as the natural sort of via gravitational pull of retention – retention decay.
How to think about granularity
I think the way you do that is to determine which of those dimensions are actionable from a user acquisition standpoint. Because if you think about this exercise as a way to come up with an imperative for UA, everything about this exercise is basically telling me how many new users that you bring into my target. So, the whole point of this is to tell me, “hey, I need this many users tomorrow, this many users the day after that, all the way through to the end of the month to hit my revenue target.” That’s my user acquisition roadmap so to speak, that’s my user acquisition imperative.
Scientific targeting
Now when you predict these user bases out by groups, you can be strategic about, “well, actually I only need to acquire this many Facebook users to find out how they monetize and retain versus acquiring as many users on Google because they retain and monetize worse, I can get to my goal.” You know, with this shorter path, going with the higher quality users is probably going to cost more, but you bake all of this into a broader P&L model, and then you just get certain scientific results such as how you break this out and optimize the composition.
FULL TRANSCRIPT BELOWShamanth: I’m very excited to welcome Eric Seufert to the Mobile User Acquisition Show. Eric, welcome to the show!
Eric: Thank you so much for having me.
Shamanth: Glad to have you on the show. In this episode, we are going to talk about one of the very many things that you are very good at, one of the very many things that you are considered an expert in, which is applying analysis and analytics to mobile apps. Specifically, we are going to talk about how to model out DAU for an app, and we will talk about what that means. But before that, can you tell us why it is important to systematically manage growth for an app via a quantitative model?
Eric: That’s a very good question, and I think you kind of answer it in the way that you asked it. Why would you want to systematically manage growth?
If you think about a growth team that isn’t managing growth to some standard or some projection or some business requirement, then they’re just doing stuff right there – just bringing new users into the app or to the product – but without any kind of eye towards a business objective.
I think, and it is depending on the sort of elevation that a person sits within a growth team or within a company, this may or may not be something that they have to worry about, but like myself, having kind of managed a bunch of growth teams: in my career, being able to execute against some sort of business objectives, which is yoked to the overall success and growth of the company, is the imperative of someone who leads the growth team.
So, if you think about what a growth team does is really two things. One, it exploits some set of channels to the new users and cool products, and it optimizes those – that’s the ongoing thing. And then, the other thing is, it builds towards some models or some expectations of revenue growth.
And for mobile-first companies, user acquisition is singularly that engine of growth – it is the source of the users. You might have other stuff on top of that, you might have ASO, an out-of-home strategy, and brand building stuff. But for mobile-first companies like that, this singular driver of revenue growth is the user acquisition. So, you can’t be doing that in a non-methodical, non-systematic way because then there’s no way to ensure or scientifically implement that growth model that you have which is made up of those business objectives.
Shamanth: Yeah, and it’s so crucial to have that be quantified and very objectively laid out, as you pointed out. What would you recommend be the key inputs or elements to such a quantitative model that a company or an app might use to project out DAU growth?
Eric: Sure, so I wrote an article about this a few months ago. It’s called, “High Growth, Low Growth, No Growth,” and it kind of goes through it. I shared a bunch of code, and you can run the code yourself – it’s a python script, and it’s running these simulations. I kind of walked through that problem set in the post. The idea would be, we kind of have some sense of how the user base is on any given day. If you just look at the user base as a whole, as a model, you’d say, “Okay, this user base generates this much money a day.”
So maybe yesterday, the user base generated this much money, and my goal is to make that much money times two by the end of the year or the month. Well, if I know, on a daily basis, that’s how the user base changes in size, I can kind of guess…build some primitive model to see if I’m going to get to that times two value by the end of the month. Then,
when you break that user base out into atomic units, which are users, you can group them in ways that help you understand how those groups are going to make money, and how the revenue is going to change over time. Also, how those groups are going to decay and shrink over time just as the natural sort of via gravitational pull of retention – retention decay.
Once you know that, if you said, “okay, I’ve got this model, if I broke it out into the atomic units, which are like cohorts grouped by acquisition source, grouped by country, grouped by platform, grouped by whatever, and I see this user base as it was yesterday.” If I didn’t add any new users, I could understand, just based on my understanding of the historical precedent of retention for those groups: how many of these users would exist by the end of the month.
From there you can see, “okay, well, this is how much money I’m going to have, given that now your users came into the user base – only these users existed are grouped in all these different ways – groups in ways that allow me to apply retention profiles to them. I could see how my revenue is going to shrink over time or how it is going to evolve over time, and I can know or at least project and estimate how much money that user base will generate at the end of the month. And then, I could see what’s the shortfall or gap? And once I know the gap, if I know the retention profiles, then I have a rough idea of how these different groups monetize, and I understand how many new users have to be added every day in order to hit my revenue target. And then, I can estimate how much that costs and work that into an overall P&L model and company model. That’s starting from the atomic unit of the user, you sort of roll up all the way to, “hey, we have a business here, this is what the business is like.”
Shamanth: Right. So, I like that you’re phrasing ‘the atomic unit of the user.’ So you have a certain number of users coming in from different sources, and they decay over time according to the gravitational pull of the retention curve. And you would say, “this many users remained after X number of days, and this is the shortfall we need to make up for to hit a certain DAU target.” That sounds about right?
Eric: Yeah, pretty much.
Shamanth: As a follow-up question on that, Eric. As you said, the users are the atomic unit. So to speak, you can break down by the different dimensions you said, right? So you can break down by country, by source, or even by campaigns. But, I’m curious though. How do you decide what level of granularity makes sense for a specific app? I asked that because you could basically say, “well I’m going to pick users who come in from one specific creative and maybe that’s only five users today, and that’s not very meaningful.” And you might also say, “well, I’m going to take all users coming into an app which could be 10,000 users all of whom have very different properties, right?” So, how do you think about the level of granularity at which you recommend breaking down the atomic unit of users to model out the DAU in the manner that you just described?
Eric: That’s a great question.
I think the way you do that is to determine which of those dimensions are actionable from a user acquisition standpoint. Because if you think about this exercise as a way to come up with an imperative for UA, everything about this exercise is basically telling me how many new users that you bring into my target. So, the whole point of this is to tell me, “hey, I need this many users tomorrow, this many users the day after that, all the way through to the end of the month to hit my revenue target.” That’s my user acquisition roadmap so to speak, that’s my user acquisition imperative.
Now, I’ve got this imperative, which is essentially some set of marching orders about how many new users that you need to bring in. What I want to do is break that user base out into these groups that are targetable on a UA basis, so that I can analyze their retention profiles and their monetization profiles kind of independently. And then, that way I’ll be able to build this model in a way that agglomerates all that up into that top line number that I need to add.
You could think about as, “okay well, if my users from the US that I acquired on Facebook monetize and retain much better than users that I acquired from Germany in Google AdWords, then I need fewer of those to hit my goal.” And then, you can figure out the best mix of those users based on cost. The very easiest implementation, or the least kind of skated implementation of this, is to take the whole user base again, as a monolith, and then just say, “okay well, this user base shrinks this much every day without any new users. So, I need to add this many new users at the sort of global level.” The problem with that is you don’t really know what the composition of that user base is.
Let’s say that 30% is made up of Facebook users, 30% made up of AdWords users, and 30% made up of UAC users, you’d have to try to somehow match that composition in a way that you acquired users.
Now when you predict these user bases out by groups, you can be strategic about, “well, actually I only need to acquire this many Facebook users to find out how they monetize and retain versus acquiring as many users on Google because they retain and monetize worse, I can get to my goal.” You know, with this shorter path, going with the higher quality users is probably going to cost more, but you bake all of this into a broader P&L model, and then you just get certain scientific results such as how you break this out and optimize the composition.
Shamanth: I think that’s a very helpful way of thinking about it in terms of how actionable all of this granularity is from a user acquisition perspective, and I think that’s something that’s a reminder that I certainly need from time to time because it’s easy to dive into the models. But I think it’s helpful to zoom out and think about what is it that we are trying to achieve through these models as it worked. Cool, Eric. So, I think that was extremely instructive. So thank you for being on this episode of the Mobile User Acquisition Show.
Eric: Yup. Thank you so much for having me.
A REQUEST BEFORE YOU GO
I have a very important favor to ask, which as those of you who know me know I don’t do often. If you get any pleasure or inspiration from this episode, could you PLEASE leave a review on your favorite podcasting platform – be it iTunes, Overcast, Spotify or wherever you get your podcast fix. This podcast is very much a labor of love – and each episode takes many many hours to put together. When you write a review, it will not only be a great deal of encouragement to us, but it will also support getting the word out about the Mobile User Acquisition Show.
Constructive criticism and suggestions for improvement are welcome, whether on podcasting platforms – or by email to shamanth at rocketshiphq.com. We read all reviews & I want to make this podcast better.
Thank you – and I look forward to seeing you with the next episode!