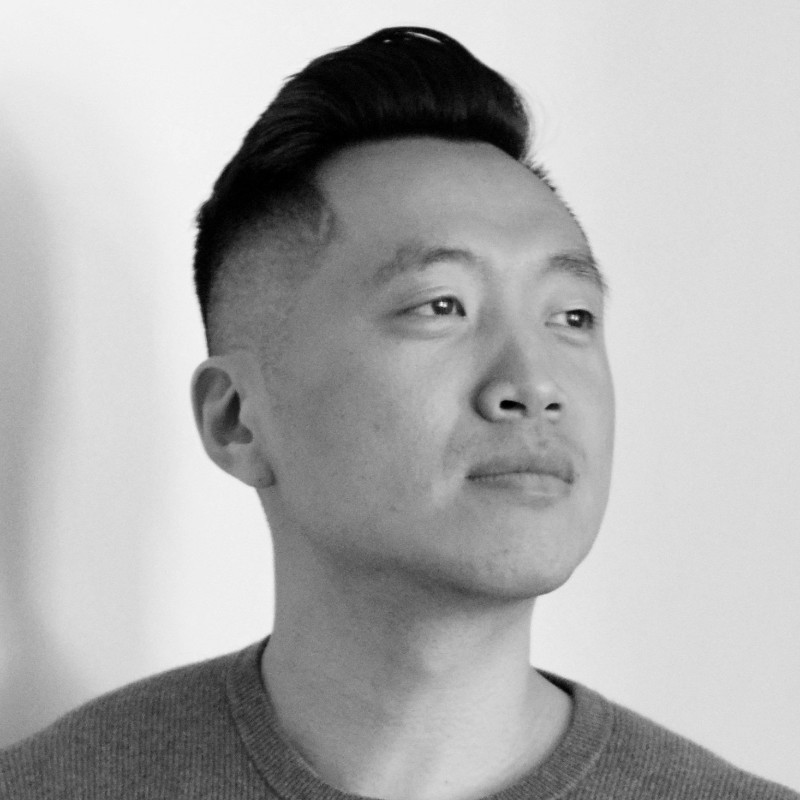
In one of our previous episodes, we discussed how media mix modelling might not be the best bet post-IDFA. However, some of you have reached out to say that it isnt quite as black and white – and that media mix models could be more widely applicable than what we’d outlined.
To throw some more light on this, we have Brian Krebs, founder and CEO of MetricWorks, who has seen first-hand the success of media mix modelling for mobile apps, especially at levels of scale far smaller than that of most CPG brands, in which space these models have traditionally been used. In today’s episode, Brian shares with us some of the ways in which these models are specifically applicable to mobile marketing campaigns – and argues that these measurement tools are not only useful post-IDFA, but also provide advantages over other measurement tools used by mobile marketers.
**
Note: We’re excited to announce the second edition of our live workshop series, Mobile Growth Lab, to help marketers, leaders and execs prep for a post-IDFA world!
In the first edition, we helped over 40 attendees:
- See the map
- Prepare the groundwork
- Move forward
- Find acceleration
We’ll cover all that (updated for the reality of the post-IDFA world) and many requested topics like web-based flows, creative strategy, conversion values, prep for iOS 15, and more.
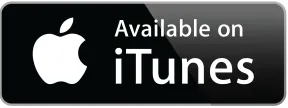
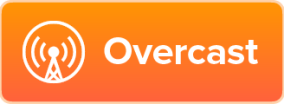
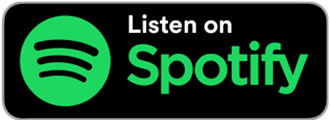

ABOUT BRIAN: LinkedIn | MetricWorks
ABOUT ROCKETSHIP HQ: Website | LinkedIn | Twitter | YouTube
KEY HIGHLIGHTS
🐯 The importance of incrementality for advertisers
💈 Is the last-touch model still relevant?
✂️ How media mix modelling differ for mobile from CPG (consumer packaged goods) companies.
🎒 Why Media Mix Models are more easily applicable to mobile apps than to CPG companies.
🍎 The key input factors for a Media Mix Model for mobile.
👩🏫 How Apple’s IDFA changes accelerated the interest in MMM-based solutions.
💠 How companies can start building media mix models.
⚜️ In what situations and at what thresholds are Media Mix Models useful?
📳 What do outcomes of Media Mix Modeling look like?
KEY QUOTES
What is Media Mix Modeling?
Media mix modeling is a technique that’s been used for decades now in really big brand advertising. Consumer packaged goods, retail, that type of thing.
It’s been used historically as a strategic type of measurement. It’s been adopted by those types of verticals and industries specifically because it works only with aggregated data, which often is all they have access to. And it gives them an understanding of incrementality rather than last touch, which only works for some digital publishers.
Last touch attribution vs. incrementality
Last touch attribution, and even multi-touch attribution, have serious, serious problems. Especially the last touch where the mobile industry has largely fallen for many years now. It really has one fundamental issue, which is it assigns all value to a single campaign. And that campaign is often just randomly chosen with some pretty specific biases towards self attributing networks and naturally high engagement ad units like video and playable that tend to just scoop up a lot of these last clicks whether or not they’re truly adding incremental value to the overall media mix.
Media mix modeling is one way to measure incrementality. But incrementality is a very, very important measure. In fact, I would argue it’s the only important thing to measure.
Why MMMs are more easily applicable to mobile apps than to other types of businesses.
It’s actually more applicable to mobile app companies than it is to other types of companies, interestingly, because there’s just fewer general significant impacts or factors that significantly impact overall app performance or product performance.
You need to understand things like seasonality. That’s one of the biggest keys. You need to understand how seasonality, day of the week, impacts. On weekends you have spikes, on weekdays you have dips, these impact your overall app-wide performance.
Daily data in mobile is very valuable
Another factor there is, mobile tends to have access to very, very consistent daily data. Whereas, other industries that have been using it for many years find it tougher to access that data consistently. So mobile is prime for media mix modeling.
Apple and Google’s privacy changes have accelerated adoption of MMM based solutions
But here we are. Apple’s IDFA changes, similar changes even if later announced by Google, and I think this is the catalyst now where we’re seeing players enter the market with these types of MMM-based solutions. And there’ll be more to come, I think, with an overall industry wide adoption in the next couple of years, really.
When incrementality is truly valuable
Incrementality really comes into play as being truly valuable when there’s more audience overlap. When you’re running on multiple channels, even two, or you’re running many campaigns across one or more channels with a bunch of targeting where there’s audience overlap.
MMM requires a different worldview
The idea for marketers with this sort of worldview – which is quite different than the worldview taken by last touch – is that conversions aren’t the product of a single touch whatever happens to get the last click before conversion.
The problem of multicollinearity
The bigger issue from a data science perspective is multicollinearity. You just can’t be spending, or having the same amount of impressions, for each campaign every single day. That’s the issue because when you have two more campaigns with the same spend and same impressions every day over time, it’s impossible for any regression mechanism – including the one used by media mix modeling – to isolate the effect of any of those campaigns.
Measuring outcomes from MMM based analysis
What if we stopped spending impressions on this campaign completely?
The model would say that your overall app-wide revenue, let’s say, would decrease by a certain amount. That is the amount of revenue that should be “attributed or allocated” to that campaign. And that allows you to and it opens the door for many, many different KPIs that are kind of traditionally last touch KPIs served by the MMP that also account for incrementality which is one of the key benefits of using an MMM.
FULL TRANSCRIPT BELOWShamanth: I’m very excited to welcome Brian Krebs to the mobile user acquisition show. Brian, welcome.
Brian: Thanks a lot Shamanth. Yeah, it’s great to be back.
Shamanth: I’m excited to have you back. I think it’s helpful to give folks a bit of background about how this episode came to be. We had an episode recently about how media mix models are not applicable to a lot of advertisers. And you guys got back and said that’s not necessarily and always true – and, there’s another perspective to all of this. To which my response was, I don’t know what I don’t know.
So here we are. You’re going to tell us about how media mix models could work for mobile apps, how you guys have seen it work, and in what situations they could work. And hopefully we can talk about when it may not work just as well.
So, let’s jump right in. Can you talk about what media mix models are and why marketers should care about them?
Brian: Yeah, that’s a great place to start.
Media mix modeling is a technique that’s been used for decades now in really big brand advertising. Consumer packaged goods, retail, that type of thing. It’s been used historically as a strategic type of measurement. It’s been adopted by those types of verticals and industries specifically because it works only with aggregated data, which often is all they have access to. And it gives them an understanding of incrementality rather than last touch, which only works for some digital publishers.
There’s tons of media properties out there where last touch just isn’t applicable. Obviously TV, influencer marketing, etcetera. Things like that often just are not addressable or trackable in the traditional way. So, these large brands have historically looked at media mix modeling as their avenue to be able to understand the incrementality or incremental value of their various media investments.
That stems off into the topic of incrementality – and your audience is much more well-informed I think, than the average – but just to give a brief overview of incrementality. It’s important because it aligns measurement with business value.
Last touch attribution, and even multi-touch attribution, have serious, serious problems. Especially the last touch where the mobile industry has largely fallen for many years now. It really has one fundamental issue, which is it assigns all value to a single campaign. And that campaign is often just randomly chosen with some pretty specific biases towards self attributing networks and naturally high engagement ad units like video and playable that tend to just scoop up a lot of these last clicks whether or not they’re truly adding incremental value to the overall media mix.
Media mix modeling is one way to measure incrementality. But incrementality is a very, very important measure. In fact, I would argue it’s the only important thing to measure.
Shamanth: Certainly. I think shortcomings of the last touch model have become increasingly clear to a lot of marketers, especially those operating on multiple channels.
To talk about media mix models in a little detail, what I’d love to do is understand how this can be applicable specifically in a mobile context. Because this has traditionally been used by CPG (consumer packaged goods) companies, how a lot of CPG companies would use it to look at a ton of variables which could include fuel prices, whether there’s sports matches going on, whether it’s a weekend or a weekday, and correlate a whole lot of these patterns to revenue. And I know the last time we spoke, you said that on mobile a lot of those variables are not practical and not even available. And you don’t want to look at fuel prices for mobile app downloads.
So what might be some of the variables you want to look at?
Brian: Yeah. Great question.
When you’re looking at a media mix model what you’re really doing is modeling all the significant factors that can potentially impact overall app wide KPIs. Revenue, installs, retention, any of those KPIs that these mobile marketers are using today, only measured by last touch.
You need to understand the lay of the land: anything that could significantly impact your app-wide KPIs. And you’re right, in the CPG world, you need to understand many factors such as competitor pricing, which can have a huge impact on consumer demand for any particular product. These types of factors aren’t very important when it comes to most mobile apps.
So the interesting thing is, media mix modeling is actually more applicable to apps. It’s true that no one solution is one size fits all.
It’s actually more applicable to mobile app companies than it is to other types of companies, interestingly, because there’s just fewer general significant impacts or factors that significantly impact overall app performance or product performance.
You need to understand things like seasonality. That’s one of the biggest keys. You need to understand how seasonality, day of the week, impacts. On weekends you have spikes, on weekdays you have dips, these impact your overall app-wide performance.
Those are significant factors. Month of the year, seasonality, is also quite important. Often towards the end of the year and towards holidays you do see increases in performance. Just seasonal increases having nothing to do with marketing.
Of course, you also have promotions. Often app companies run in-game events in the mobile gaming space. There will be sales promotions across various app categories. And even things like app-store featuring. Those things need to be encoded as ‘holidays’ into the model because they can have significant performance impacts that are unrelated to marketing.
And, obviously, the biggest factor is going to be marketing, often. Not for all app companies, but for many app companies marketing has just a massive impact on their overall app-wide performance. And we encode that in terms of spend, which is your marketing investment, and impressions, which is your marketing reach, into the model to understand marketing’s impact across your various campaigns to the overall app-wide performance.
There are a few other factors that you sometimes see that can be very interesting. For example, app wide, like ARPDAU or some other indicator that helps you understand, kind of rising ships, where there’s a lot of live ops. Sometimes, some live ops will be doing some huge impactful fluctuations in terms of your overall app performance that aren’t really correlated technically with marketing. ARPDAU and related metrics can be a nice control to factor live ops into the equation, when those are very, very often or are frequent and very impactful.
So, it can vary a little bit but those are the main factors that most companies need to understand or model the overall impacts to their app wide performance. And from there, it’s just a matter of isolating that marketing influence.
But, you do need to model everything that can significantly impact app-wide performance in order to accurately isolate the two marketing effects.
Shamanth: So, what I’m hearing you say is, you still need to correlate inputs and outputs. And instead of those inputs being fuel prices or weather patterns, you’re treating very different sets of inputs for mobile apps, which could be seasonality, featuring, live ops, and everything.
And a lot of those are readily available, much more easily than fuel prices.
Brian: It’s true. Especially when you look at competitor pricing. Big brands have access to a lot more data, but it costs them some serious money from a partner like Nielsen or someone like that. That’s data that’s very tough to come by.
The beautiful thing about app companies is that, that doesn’t really impact sales or revenue so much in the mobile space. So it’s this odd thing that there’s this confluence of factors here that makes this even more applicable to mobile than where it’s been used traditionally for many years now.
Another factor there is, mobile tends to have access to very, very consistent daily data. Whereas, other industries that have been using it for many years find it tougher to access that data consistently. So mobile is prime for media mix modeling.
It’s just never focused energies on applying media mix modeling concepts to mobile for a variety of reasons. Honestly we just got hooked into last touch attribution, device-level data.
We have access to it, many industries don’t and it was very, very easy. It took a super simplistic view that made it a very simple engineering problem to solve. In fact, quite a few large mobile companies have rolled their own tracking. It’s just something that is a much more simple problem to solve.
But here we are. Apple’s IDFA changes, similar changes even if later announced by Google, and I think this is the catalyst now where we’re seeing players enter the market with these types of MMM-based solutions. And there’ll be more to come, I think, with an overall industry wide adoption in the next couple of years, really.
Shamanth: You talked about the input variables, in a mobile space. Do they differ by different genres of mobile apps, for lifestyle subscription apps as compared to games? Or do they not?
Brian: Yeah, so they can, they can. Just as an example, if you’re a weather app. Somehow, including weather patterns into the app on a per region basis is probably pretty important because it’s a factor that almost definitely impacts significantly the overall performance of your app.
Probably very, very impactful weather events, like disasters, must also be encoded, but those can be encoded as holidays, sort of what I mentioned as a promotion. Although it sounds cynical, you can encode those dates in the model fairly straightforwardly. Definitely, somehow encoding weather patterns would be important for apps in the weather space.
This is not a one size fits all solution, for sure. Some apps will have other factors necessary to encode into the model to account for other, non-marketing impacts. But, for the vast majority the factors that I’ve mentioned are pretty static across many genres.
Shamanth: Sure. That makes sense. And, let’s just say a team wanted to execute on this approach of correlating revenue outcomes to marketing inputs and of course, extraneous inputs. Where would they begin? What would their first steps look like? What would be some of the statistical techniques they might employ to find a relationship between the inputs and outputs?
Brian: Number one, because media mix modeling has been around for so long and used actively in a variety of huge companies, we get the benefit of years and years of research on the academic side. So number one is, if you have the internal data science talent shifting their focus into the academic area, the available papers we have out after years and years of research is one huge benefit to media mix modeling in general, and kind of an easy way to dip your feet into understanding the concepts at a more scientific level. There’s also case studies, things like that. It’s just been a technique used actively for so long. There’s just a wealth of knowledge that already exists out there.
The other thing is because it is such a well understood technique there is also some open source code out there and frameworks. There’s Facebook’s Robyn. It’s written in R, Robyn spelt with a y. They have an internal team of kind of attritions that have developed this framework in R that gives people a starting point from which to understand media mix modeling and kind of go from there if they understand the R language.
So there’s even some frameworks pre-developed in open source out there as well, that can be used as sort of reference implementations, if you will.
And of course, as I mentioned, I personally believe that this wave is getting a lot of traction in terms of thought leadership and discussion around the industry. I do believe it’ll gain wide adoption as well over time. And we’ll see more and more out of the box SAAS solutions as well. Including the one that we at Metric Works provide.
There’s going to be much easier paths to not only try it out and play with it, but of course also implement it without the headache of developing your own. But developing your own, especially for some app categories, may be the best route. And, there’s quite a few tools at your disposal to do that.
Shamanth: Especially for smaller developers that don’t have a ton of internal resources, I think something like an out-of-the-box solution, something that can be customized, would be valuable.
And speaking of the applicability of these techniques. In order to understand the relationship between inputs and outputs you will need a certain threshold volume of data. For instance, if you are on a single channel, you’re not doing a lot of spend it’s just not meaningful or even necessary.
What’s the threshold at which this becomes valuable or even possible? What’s the threshold at which it’s useful and possible to do media mix modeling analysis?
Brian: Yeah. That’s a great question. And I like the way you posed it, because it is sort of two questions, two different questions. One is where is it useful, where is that threshold? And, the other is where is it even possible?
When you’re looking at useful or valuable, media mix modeling and measuring incrementality rather than last touch in any way using any methodology is obviously only one potential methodology for incrementality.
Measurement is most valuable when there’s a complex media mix. Complexity doesn’t have to be high, but if you’re only running on, let’s say, a single channel and a couple of campaigns on that channel, the audience overlap between those campaigns on that single channel probably isn’t too high.
Incrementality really comes into play as being truly valuable when there’s more audience overlap. When you’re running on multiple channels, even two, or you’re running many campaigns across one or more channels with a bunch of targeting where there’s audience overlap.
When that happens, you’re hitting the same users with ads, just in different properties or inventory. And that causes a situation when potentially not all of that media spend is actually incremental. You may be serving ads to a user multiple times in different properties where some of those ads aren’t actually truly budging the needle on that user’s likelihood to convert.
The idea for marketers with this sort of worldview – which is quite different than the worldview taken by last touch – is that conversions aren’t the product of a single touch whatever happens to get the last click before conversion.
Conversions instead are a product of a very cohesive media mix that provides a consistent message to users across a variety of properties that they spend time in. So really, it’s a refactoring of a complete understanding of how one should be looking at measurement fundamentally.
But yeah, there’s this threshold for usefulness or value. And it really starts at just a couple of channels or a bunch of campaigns with overlap in targeting on a single channel. Where that overlap can cause cannibalization between the various paid media that you’re spending money on.
But there’s also another factor: organic demand. As soon as you have some significant level of organic demand, which obviously for brand new titles that aren’t licensing well-known IP (intellectual property) maybe is quite low. But, even after a few months, you’re going to have some level of organic demand assuming your app is performing well. And these paid media sources can start cannibalizing organic demand as well, not just each other.
So, incrementality becomes ever more important as the media mix becomes more complex, even up to two channels or more. And as organic demand starts increasing.
Then you start having the data science side of the question. What’s even possible? What is that threshold? And the threshold there is quite low. Really,
as long as you’re spending even a couple hundred dollars a day, you’re fine in terms of understanding the coefficients or the incremental contributions of each campaign.
The bigger issue from a data science perspective is multicollinearity. You just can’t be spending, or having the same amount of impressions, for each campaign every single day. That’s the issue because when you have two more campaigns with the same spend and same impressions every day over time, it’s impossible for any regression mechanism – including the one used by media mix modeling – to isolate the effect of any of those campaigns.
Very rarely do you see this problem, especially over a lengthier period of multiple days rather than just a few, because there’s only so much you can control in terms of how much spend you have on each campaign and how many impressions are coming through each campaign.
So many other factors outside of your control, like bid competition and others, are affecting how much you’re actually spending up to your budget and how many impressions you’re getting.
So it’s very rare to run into really, really serious multicollinearity problems over a longer period of time. But multicollinearity problems are more prevalent at super low spend levels. So that’s the bigger factor that you need to look at. But even usually a couple to a few hundred dollars a day is plenty.
Shamanth: And it sounds like if that threshold is low, then the big blocker, if you will, is the expertise and resources to actually execute on this analysis.
Brian: A hundred percent. Yeah.
Shamanth: And as you said, I think there’s steps being taken to make that simpler, to hopefully get that to a place where it could be out of the box or close to it. But it sounds like that is the bigger hurdle for the vast majority of developers today.
Brian: Yes. That’s going to be your main constraint for adopting media mix modeling or similar concepts. And obviously the first real threshold you’re going to run into is the usefulness threshold. Companies that are only spending on a single partner with very little organic demand probably just aren’t going to find any usefulness anyway even by utilizing a third-party SAAS solution.
Shamanth: And if you run this analysis, what do the outcomes of this exercise look like?
Brian: Great question. So there’s a few. You can kind of classify them into two categories, traditional media mix modeling outputs and let’s say mobile-specific outputs.
In your traditional media mix modeling outputs category, you have things that the huge brands have been using media mix modeling for decades. They are using media mix models to understand the ad stop effect. You know, how long do ads, after they’re served or purchased actually affect user behavior. After the ad has been served, usually there’s some declining or decaying effect after an ad has been served where the ad still resonates or affects the user behavior. They like to understand that at least on a channel by channel basis.
They also like to understand the incremental contributions of each individual campaign using what we call a coefficient in the model. But it’s just sort of a number that says, this campaign has a four and this campaign has a two, meaning that the first campaign has doubled the incremental contribution to revenue as the second campaign. So just kind of graphing out on a pie chart what the relative incremental effects are of each campaign. That’s a huge output that’s been kind of traditionally used.
There’s also one that’s derived from both of those outputs which is what-if analysis. That requires a model that understands the S curve. Understands where the spend levels or impression levels on each channel will start hitting diminishing returns due to a maximum reach available on that particular channel or campaign.
But traditional media mix models understand that. And, once you fit a model successfully, you can start doing things like asking the model, “Well, you know the incremental contributions of each of my campaigns, what would happen if I double my budget on this campaign? What would happen if I stopped spending impressions completely on this campaign?”
And you start getting this nice strategic what-if analysis, that can help inform your business decisions. And of course, specifically your marketing decisions.
When you look at mobile, we have some different needs. Number one, decision-making needs to happen on a day by day basis for many, many app companies. Unlike where media mix modeling has traditionally been used in terms of strategic decision-making, where decisions are made on maybe a monthly or a quarterly basis.
Also, processes right now, especially in the marketing or UA side, have been built to rely on pretty specific KPIs. Usually cohorted by installed date, D7 revenue, D7 retention, D7 LTV, D7 ROAS, D14, 21, sometimes even prediction models are used to predict ROAS like 360 days later or 365 days later.
These types of KPIs are the outputs of MMPs. And naturally these processes have led most mobile marketers to rely on that data.
One of the innovations we’ve made at Metric Works, and I think something that’s very important for people who decide to roll their own media mix modeling solution to understand, is to minimize the level of impact to your overall UA product. Translating the model to the same KPIs that process is already relying on, you can swap out the last touch data points coming from the MMP – D7 ROAS, D14 ROAS – with incrementality versions of those same KPIs that are coming from an MMM, simply by doing a very specific type of what-if analysis.
What if we stopped spending impressions on this campaign completely?
The model would say that your overall app-wide revenue, let’s say, would decrease by a certain amount. That is the amount of revenue that should be “attributed or allocated” to that campaign. And that allows you to and it opens the door for many, many different KPIs that are kind of traditionally last touch KPIs served by the MMP that also account for incrementality which is one of the key benefits of using an MMM.
Shamanth: Sure. There’s a lot of legacy measures from the CPG world. But, from what you’re saying, it could make sense to look at what’s the incremental ROAS, what’s the incremental revenue lift from a certain campaign. And to translate that back to more mobile specific terms.
Brian: Exactly. It makes it much more easily actionable.
And on top of that, it gives you a really nice way to look at the status quo. Your last touch, ROAS D7 let’s say, side by side for the same campaigns with an incrementality version of that ROAS.
And you can start analyzing it in a nice way. Where last touch has for many years probably undervalued certain traffic, and of course, overvalued certain traffic, where obviously in those cases your media spend is inefficient.
And there’s ways to even confirm the output of the model. By running ground truth experiments and a variety of ways that don’t require device IDs. That’s one of the big keys for MMM and its resurgence in mobile. It doesn’t require any device level data which makes it compatible with Apple’s and Google’s changes to their platforms in terms of privacy.
But there’s experimental ways to obtain ground truth, rather than modeled truth, without using device-level data. So, I would say that it’s also important to kind of trust those model outputs by constant experiments, as well, to continue validating and improving the model.
Shamanth: Yeah. Brian, this has been very instructive and I know we’ve come up on time.
So this is perhaps a good place for us to wrap.
Thank you so much for being a guest at the Mobile User Acquisition Show again. We’ll link to everything you do in the show notes as well. But for now, thank you so much for being a guest on the show.
Brian: Thanks so much for having me back.
Shamanth: Thank you for listening to the Mobile User Acquisition Show. If any of this was helpful or instructive, I would love for you to leave us a review or rating on iTunes, Stitcher, Overcast, or wherever you get your podcast fix. This podcast takes a ton of time, effort, and love to produce. And I deeply value every review and every piece of feedback that you share.
Thank you for listening. And I look forward to sharing our next episode.
A REQUEST BEFORE YOU GO
I have a very important favor to ask, which as those of you who know me know I don’t do often. If you get any pleasure or inspiration from this episode, could you PLEASE leave a review on your favorite podcasting platform – be it iTunes, Overcast, Spotify or wherever you get your podcast fix. This podcast is very much a labor of love – and each episode takes many many hours to put together. When you write a review, it will not only be a great deal of encouragement to us, but it will also support getting the word out about the Mobile User Acquisition Show.
Constructive criticism and suggestions for improvement are welcome, whether on podcasting platforms – or by email to shamanth at rocketshiphq.com. We read all reviews & I want to make this podcast better.
Thank you – and I look forward to seeing you with the next episode!