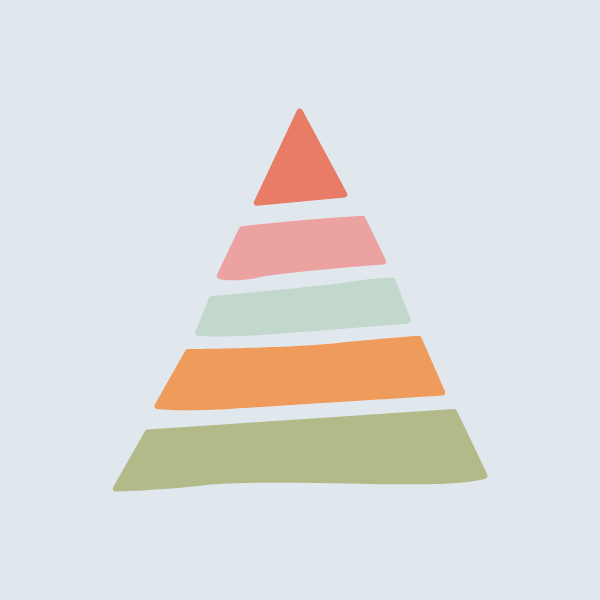
Why do a small number of ads get most of the spend on Meta?
In this episode, we’ll talk about why this is good for your ads – and discuss the probabilistic Bayesian testing paradigm as well, which so you understand how Meta’s algos make these decisions so you can construct your creative testing process more intelligently.
We break this down in today’s episode.
We go into this – and every other aspect of creative testing post-ATT in our new book, which you can download for free: The Definitive Guide to Meta Creative Testing post-ATT.
***
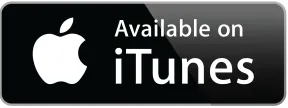
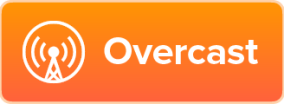
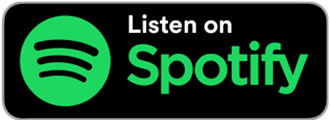

ABOUT ROCKETSHIP HQ: Website | LinkedIn | Twitter | YouTube
FULL TRANSCRIPT BELOW
Today’s episode covers a topic that we address in a lot more detail in our new book: Definitive Guide to Meta Creative Testing in a Post-Identifier World, which covers every aspect of how to run creative tests post-ATT, so you can run your tests in a world of incomplete data to discover winning ads with confidence.
A very common(and often frustrating) phenomenon in Meta ads is that a small number of ads get the lion’s share of ad spend.
This is often frustrating when ads that you want to see statistical significance for don’t get enough spend and performance – so it’s hard to do apples-to-apples evaluation of ads(unless you set up specific split-testing campaigns).
In today’s episode I’ll explain exactly why Meta’s algorithm behaves this way – so you can understand and adapt to these changes in your testing and performance.
Let’s start by understanding the disadvantages of having each ad get the same number of impressions:
- If each ad in an ad set gets the same number of impressions, oftentimes a worse performing ad gets as many impressions as a better performing ad – so your overall performance is far worse than it would be if your best ad got the most spend, and other ads got lesser amounts of spend.
- This is a subtler point, but oftentimes different ads are better for different users. Ad A might be better for some users(say men over 50, who might comprise 50% of your audience), and ad B is better for others(say women between 20 and 30, who might comprise 10% of your audience). You want most men over 50 to see ad A, and women between 20 and 30 to see ad B – and thus you want to have unequal allocation of spends and impressions between ads.
So how does the spend and impression allocation happen in practice? Facebook’s algorithm uses a probabilistic Bayesian testing paradigm, which basically uses past information about performance(‘priors’ – in this case distribution of spend or impressions to ads) to make future predictions(in this case conversions or revenue) – and find the optimal combination of priors that lead to maximum outputs(in this case, it finds the optimal combination of spends distributed among ads that results in the maximum conversions or revenue).
In the beginning(assuming all ads are brand new), the algorithm has no information about priors – and there is no performance history. At this point, the algorithm distributes impressions more or less evenly among all ads in an ad set at this point.
But as soon as the algorithm starts to ‘learn’ and infer which ad has a higher probability of leading to conversions or revenue, it starts to give more impressions to the performant ads, and less impressions to the ads with a lower probability of leading to conversions or revenue.
The algorithm also tries to find the right balance between ‘exploration’ and ‘exploitation’ – with exploration, the algo tries to give impressions to new ads that may not have history in order to ‘discover’ potential winners, and with exploitation, the algo doubles down on a proven ad to maximize performance.
As you can imagine, there can be inaccuracies and challenges – oftentimes because Meta doesn’t have enough performance history on some ads – and at others because Meta’s probability calculations are thrown off by data delays post-ATT.
In spite of these imperfections, hopefully this episode gives you some insight into *why* Meta’s algo behaves the way it does(and why this leads to better outcomes than offering the same number of impressions to each ad in an ad set).
Today’s episode covered a topic that I address in a lot more detail in our new book: Definitive Guide to Meta Creative Testing in a Post-Identifier World, which covers every aspect of how to run creative tests post-ATT, so you can run your tests in a world of incomplete data to discover winning ads with confidence.
You can check out this and our other books at rocketshiphq.com/playbooks.
A REQUEST BEFORE YOU GO
I have a very important favor to ask, which as those of you who know me know I don’t do often. If you get any pleasure or inspiration from this episode, could you PLEASE leave a review on your favorite podcasting platform – be it iTunes, Overcast, Spotify or wherever you get your podcast fix. This podcast is very much a labor of love – and each episode takes many many hours to put together. When you write a review, it will not only be a great deal of encouragement to us, but it will also support getting the word out about the Mobile User Acquisition Show.
Constructive criticism and suggestions for improvement are welcome, whether on podcasting platforms – or by email to shamanth at rocketshiphq.com. We read all reviews & I want to make this podcast better.
Thank you – and I look forward to seeing you with the next episode!