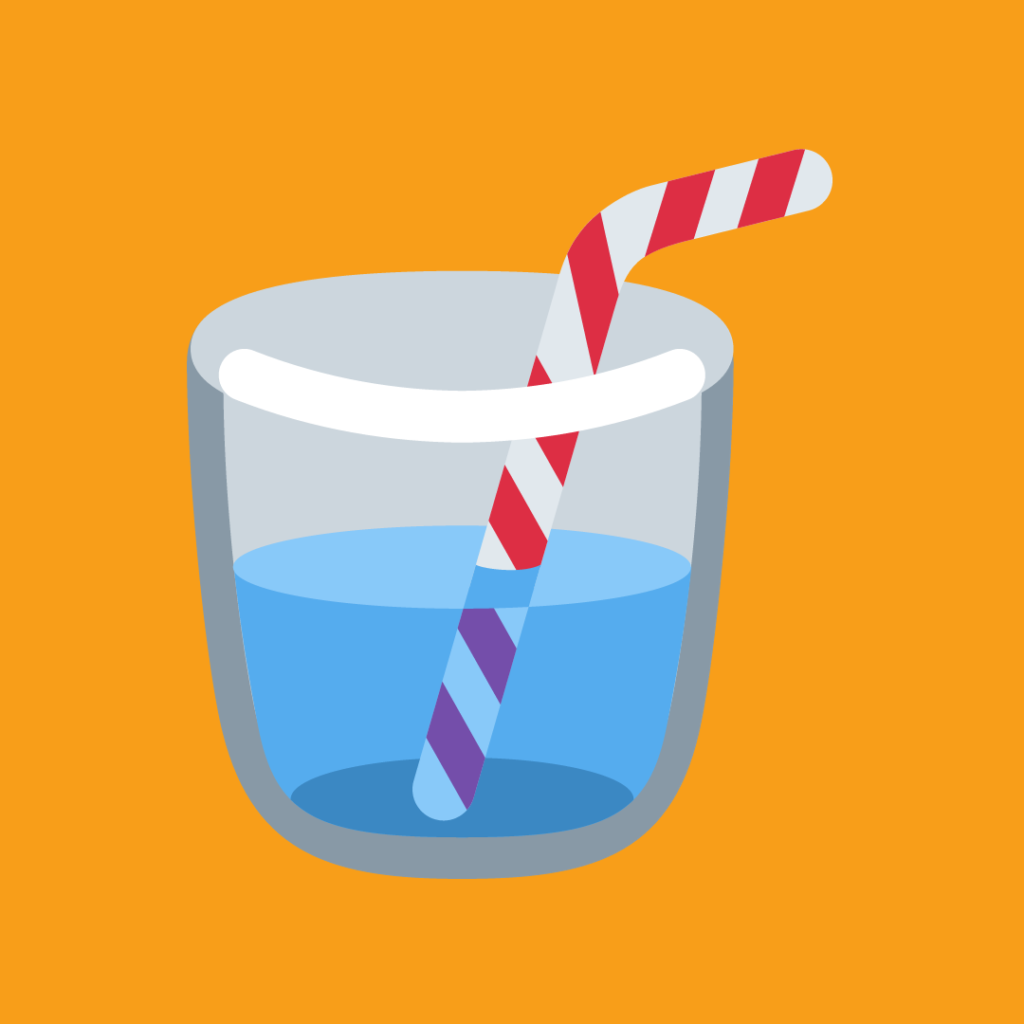
In this episode we dive into the sometimes frustrating mechanics of how Meta allocates spend between ads in an ad set – and what we recommend doing about this.
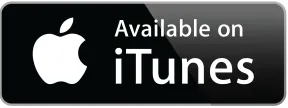
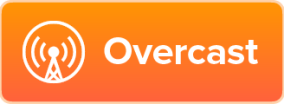
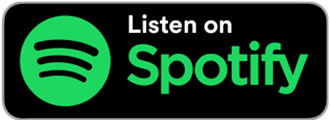

ABOUT ROCKETSHIP HQ: Website | LinkedIn | Twitter | YouTube
FULL TRANSCRIPT BELOW
A common challenge that can be a source of a lot of frustration for marketers is how Meta seems to allocate spend between different ads. This can be a huge source of frustration because oftentimes marketers and their creative teams spend a ton of time on research, understanding user motivations, reviewing user research, direction, production, post-production, and putting together ads with painstaking amounts of detail only to find that the algorithm gives very, very little spend to these ads.
That can be so frustrating, and while a lot of marketers understand that the algorithm gives more spend to better ads and less spend to worse ads, it’s still perplexing why ads that, in theory, should work and work well, don’t seem to get any spend in practice.
To understand this, it’s worth unpacking how Meta’s algorithm works under the hood. Let’s get into it today. Meta allocates spend between ads by means of the Bayesian Bandits paradigm. Now, that is a very cool name for an algorithm or an approach and how it works is through an explore-exploit approach.
What that means is as soon as an ad is introduced into an ad set, it goes into explore mode, which means the algorithm starts to explore if the ad has enough propensity to attract clicks, installs, registrations, purchases, or whatever metric you’re optimizing for. The moment the algorithm concludes that the ad is actually attracting enough events, which could be clicks, registrations, installs, purchases, or whatever you are optimizing for, the algorithm shifts to exploit mode. The algorithm then says basically, “Hey, this ad is getting a lot of purchases, let me give it as much spend as possible.”
Now, the reason why historical ads get a lot of spend is because the algorithm has a lot of conviction that these ads are attractive, resulting in purchases and installs, and funnels a lot of spend towards these. A new ad, which has very little history, is pitted against an existing ad, and the new ad has so little history that it gets very, very little spend. T
he reason the algorithm is constructed like this is because if the algorithm were to offer an equal amount of spend to all new ads, it would blow through your budgets very, very quickly, and you would blow through your budgets by spending them on unproven, untested ads which could result in poor performance.
That is why the Bayesian Bandits algorithm, while frustrating, is perhaps the best possible approach given the circumstances of testing new potential ads. That is why we recommend testing all new ads separately from existing ads in a separate testing ad set. I hope this gave you some understanding and insight into why and how Meta’s spend allocation algorithm works and what you can do about it.
BEFORE YOU GO
I have a very important favor to ask, which as those of you who know me know I don’t do often. If you get any pleasure or inspiration from this episode, could you PLEASE leave a review on your favorite podcasting platform – be it iTunes, Overcast, Spotify, or wherever you get your podcast fix. This podcast is very much a labor of love – and each episode takes many many hours to put together. When you write a review, it will not only be a great deal of encouragement to us, but it will also support getting the word out about the Mobile User Acquisition Show.
Constructive criticism and suggestions for improvement are welcome, whether on podcasting platforms – or by email to shamanth@rocketshiphq.com. We read all the reviews & I want to make this podcast better.
Thank you – and I look forward to seeing you with the next episode!