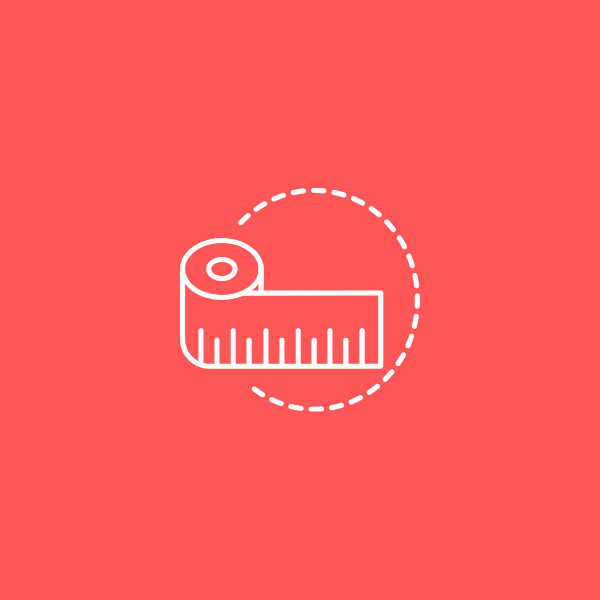
MMM based incrementality models: Is it the light at the end of the tunnel?
Post IDFA, we were quite skeptical about employing MMM based incrementality analyses in our measurements. Our opinions have changed over time and today, we believe that these models have helped us in our measurements significantly.
However, it’s not always hunky-dory. There have been quite a few challenges that we encountered on the way. On the upside, we’ve also figured out how to overcome these challenges.
In this episode, we go over the key limitations of employing MMM based incrementality analysis and include steps to mitigate these limitations.


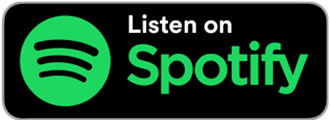

ABOUT ROCKETSHIP HQ: Website | LinkedIn | Twitter | YouTube
FULL TRANSCRIPT BELOW
One of the things we’ve changed our mind about in the last few months is incrementality analyses based on media mix modeling.
You can look back at some previous episodes to see our initial skepticism – but now we do definitely believe that incrementality models based on MMMs is a huge part of marketing measurement, especially on iOS where measurement is fundamentally broken.
Yet in our own day to day use of these models, we’ve noticed that these are not silver bullets. These models are but one tool in a marketer’s arsenal – so it’s helpful to be mindful of the limitations of these models(even though we find these models to be enormously impactful).
In today’s episode we’ll talk about some of the practical challenges that we recommend marketers be wary of – as they implement MMM based incrementality models. We also recommend ways to mitigate some of these limitations below. Without further ado, here we go:
- Correlation isn’t (always) causation
If you start 3 different campaigns on Facebook, increase budgets on Snapchat, start testing a new channel, and it’s Black Friday – and you see an incremental lift in your revenue or performance, it’s hard to isolate which ones of these led to your revenue lift, and which ones did not.
Some models have techniques to estimate the individual contributions – but you still have to be careful about the fact that you dont conclusively know this.
The solution: intentional experimentation
How we recommend mitigating this is by being intentional about the ‘experiments’ you run – and the impact you measure from these. So: if you want to measure the impact of let’s say a CPA campaign on Facebook, make sure you make a big or significant change in the CPA campaigns – and try not to change other variables in your marketing mix by too much.
- Creative impact is hard to isolate.
Most models typically account for changes at campaign and channel level, and what this can sometimes mean is that creative changes arent directly accounted for by these models. This is understandable because models find it hard to account for very granular changes(like at the creative level, where spends can be small compared to campaign or channel levels).
However because creative is a very very critical lever in impacting marketing performance, not measuring creative impact can be a huge handicap.
The solution: bake creative changes into experimentation cadence
As mentioned above, intentional experimentation is a big part of our approach. Because models dont naturally account for creatives, we make sure to bake creatives and creative changes into our experimentation.
Practically, here’s what that looks like: if an early creative concept is showing promise in early testing, we set it up along with its variants in a new campaign, give it significant budgets and ensure we measure the incremental impact of this as a part of our experimentation cadence.
- Platforms’ learning phases can give muddy results
You might run an experiment with a new campaign, channel or optimization type that may eventually end up being hugely incremental. However because of the way the platforms operate, the campaigns need to accumulate enough signal before they start to perform and yield results. This is much more the case with Google UAC, to a lesser extent with Facebook ads – and certainly the case with many programmatic channels.
What that means is that you might sometimes see poor incrementality – but that could just be because the platform is in its ‘learning phase’ – and you might make a wrong decision.
The solution: give experiments enough time to get platforms out of their learning phase
What we make sure to do is give platforms at least a week after big changes to see the changes stabilize and result in some consistency of performance. This avoids the trap of your seeing poor performance out of the gate just because the platform is still stabilizing.
- Results can be inconclusive
Sometimes we just see results that aren’t clear wins or losses. You might see incremental installs but cannibalization in purchases as a result of starting a new campaign – and you might wonder if these were a result of it being a weekend or a holiday.
What’s clear is that incrementality analysis is not a silver bullet. It isn’t always going to give a clear cut signal in the way that deterministic IDFA based attribution used to(it’s a different thing that deterministic attribution had a different set of problems) – so it’s important to realize and be prepared for occasional ambiguous results.
The solution: use incrementality models alongside SKAN reporting
We typically review incrementality analyses alongside SKAN metrics. Because both provide one part of the picture, we try to combine the insights from both to inform our next steps. For instance, we sometimes see inconclusive incrementality but strong SKAN performance – or the other way around: and decide to double down on a channel or campaign; or ‘re-experiment’ with it in our incrementality experiment cadence.
In summary, especially in the absence of identifiers, MMM based incrementality analyses are a huge step forward for measurement – but it’s important to note that these aren’t perfect, and that it’s important to think through ways to mitigate their limitations.
A REQUEST BEFORE YOU GO
I have a very important favor to ask, which as those of you who know me know I don’t do often. If you get any pleasure or inspiration from this episode, could you PLEASE leave a review on your favorite podcasting platform – be it iTunes, Overcast, Spotify or wherever you get your podcast fix. This podcast is very much a labor of love – and each episode takes many many hours to put together. When you write a review, it will not only be a great deal of encouragement to us, but it will also support getting the word out about the Mobile User Acquisition Show.
Constructive criticism and suggestions for improvement are welcome, whether on podcasting platforms – or by email to shamanth at rocketshiphq.com. We read all reviews & I want to make this podcast better.
Thank you – and I look forward to seeing you with the next episode!