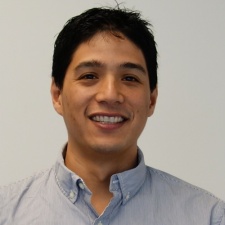
In our conversation today, Chris Farm, co-founder and CEO of Tenjin, talks about the paradigm of attribution modelling. We have previously discussed the flaws of last-touch attribution on the show before.
It is an especially critical time for attribution, as we move away from IDFAs. Chris illustrated how much of the information we feel we are losing can be arrived at from other angles: by using models to estimate these metrics from what we do have available. Models may spell inaccuracy for many marketers, but it’s easy to forget that some of the metrics we use today are also model-based.
This was a fascinating dive into the mechanics of attribution in a world with incomplete data and its many challenges and opportunities. Enjoy!
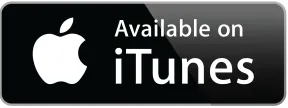
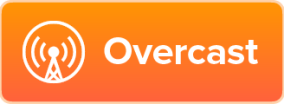
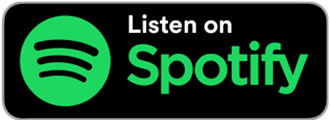

ABOUT CHRIS: LinkedIn | Tenjin
ABOUT ROCKETSHIP HQ: Website | LinkedIn | Twitter | YouTube
KEY HIGHLIGHTS
How attribution has shifted to Apple
The gaps in ROI metrics for developers
How attribution modelling works
The inputs of an attribution model and the downstream metrics they impact
Let’s not forget the IDFV
The IDFV is more powerful that you would expect
Signal-based vs deterministic
How to start with a simple model and then refine with layered variables
The drivers of model accuracy and inaccuracy
We need to change how we define accuracy
Last-click is just one possible model
It is possible to create models without postbacks
How to work with aggregate data for good decision-making
Why attribution modeling is unnecessary when running a single channel
Attribution modelling is especially suited to gaming companies
The difference between attribution modeling and last-touch
Why true multi-touch still doesn’t exist
How to create a V1 model in Excel
Developers will rise to the challenge
KEY QUOTES
There are still signals you can use
SKAdNetwork introduces the concept that there’s no identifier any more that ties it to a specific campaign. But there are signals, for example, like postbacks that are sent to the ad networks that can be shared as raw data. And even signals like counts of postbacks that have come in, or sources of that information, can be helpful in modelling out how you would distribute or allocate the LTVs that you can still measure.
How to use IDFV for attribution modelling
Let’s say you see an IDFV come in today, and then we see 10 postbacks from a specific campaign for a specific conversion value come in, within a time period that’s acceptable by Apple—that 24 to 48 hour window, post conversion value. So let’s say that a conversion value 0 happens today, and then we see 10 conversion value 0s has happened within the next two days. A very simplistic argument for determining a probability might be that that IDFV had a 1 in 10 chance that it’s linked or matched to any of those IDFV.
Awareness is key
The recommendation is you have to have your eye on each type of model, and just the business in general. If you see sales go up in general, and you can correlate it to certain characteristics, it’s helpful. And if not, then iterate on what the best solution is for you.
It’s important to choose the right model for your usecase
I would say—specific to, at least, our types of clients who are more on the gaming side and have much earlier LTV thresholds—you need a different type of modelling than mixed media modelling, simply because mixed media modelling takes too long. You’re running these massive regressions and that requires lots of data to draw conclusions. And so, that’s why you need something in the middle between what IDFA was, and this mixed media modelling, incrementality type solution.
FULL TRANSCRIPT BELOWShamanth: I am very excited to welcome Chris Farm to The Mobile User Acquisition Show. Chris, welcome to the show.
Chris: Thanks. It’s great to be here.
Shamanth: Absolutely, Chris. I’m thrilled to have you also because I feel like you and I first connected in 2015 or so, and I’m glad that our paths have continued to cross.
Chris: It’s a small world for sure.
Shamanth: It’s a small world, and it can seem like an eternity—6 years can seem like forever. I’m also excited and thrilled to talk to you about what’s changing in our world just now. Certainly, a big part of what’s changing is measurement and tracking, and you have very unique perspectives, very interesting insights, all of which I would like to dive into today.
Let’s start by having you tell us about what you mean when you say attribution modelling. How would you define that in the way that you have described it?
Chris: Yeah, sure. So we’re a company that basically tries to help app developers figure out their return on investment. And with the upcoming changes in iOS, a lot of the responsibility has shifted to Apple in order to do the attribution piece of the puzzle. Some of the things that are left hanging are the developers’ understanding what to do with what has been given to them. There’s not a lot of great tools—not a lot of easy usable tools—to understand what most app developers are used to: things like retention and cohorted metrics.
So what we tend to do is focus on the attribution modelling piece, and the way we would describe this is it is a signal-based approach. Since we are no longer expecting to have a lot of IDFA for deterministic attribution anymore, we can take the signals that Apple, through its SKAdNetwork APIs provide, in order to do LTV and ROI estimations for modelling attribution.
We are moving away from the deterministic world, whether it be fingerprinting or IDFA-based attribution, to one which is a lot more privacy-centric, according to Apple, where things are not based on identifiers any more. They’re based on heuristics and signals that they’re able to provide through their APIs. And so, attribution modelling is really this concept—that we’re talking about more frequently with developers—to effectively still keep the metrics they’ve always wanted, which is LTV and ROI, using a new methodology.
Shamanth: Understood. So what you’re saying is: “Look, you’re not going to have exact, precise metrics any more, but those metrics can be modelled, those metrics can be estimated, given the somewhat incomplete information we do have from Apple.” Can you speak to what some of the inputs in these models are? What are some of the variables that are used to compute these metrics?
Chris: Yeah, sure. LTV at the app level can still easily be calculated, and is very privacy-centric, according to Apple, using IDFVs. So it’s very possible to still calculate downstream metrics off of an IDFV, and also cohort on top of those metrics using IDFV. So the analytics for the app level is still very well preserved.
SKAdNetwork introduces the concept that there’s no identifier any more that ties it to a specific campaign. But there are signals, for example, like postbacks that are sent to the ad networks that can be shared as raw data. And even signals like counts of postbacks that have come in, or sources of that information, can be helpful in modelling out how you would distribute or allocate the LTVs that you can still measure.
We basically look at these two types of signals: there is the raw level data, which is SK postbacks—which Apple does send to the ad networks; as well as the aggregate level data that some of the ad networks can still provide. So you can take these two inputs, and try to map that and try to allocate according to the LTVs that have been developed.
Shamanth: Sure. I think something I would underscore in what you said is, you still have app level ROAS and LTV numbers, which I think can be a fact that can be somewhat easy to forget in a lot of the conversation that’s going on. And I know you said it’s based off of IDFV, and I also like to point out to developers that I’m speaking to that that data is available in the analytics systems. It is present in their MMPs, if all they looked at was the overall aggregate numbers. So, in a sense, the IDFV is not a mythical, strange thing; it is what already lives in their systems and is available. I think that’s a good call out, and it’s something that’s helpful and useful for people to understand, and to underscore that a lot of the analytics just doesn’t go away.
Chris: Totally. A good example of using the IDFV to generate what we call attribution modelling—
let’s say you see an IDFV come in today, and then we see 10 postbacks from a specific campaign for a specific conversion value come in, within a time period that’s acceptable by Apple—that 24 to 48 hour window, post conversion value. So let’s say that a conversion value 0 happens today, and then we see 10 conversion value 0s has happened within the next two days. A very simplistic argument for determining a probability might be that that IDFV had a 1 in 10 chance that it’s linked or matched to any of those IDFV.
So there’s a lot of modelling that can go into what SKAdNetwork does provide, as well as some of the other signals that still exist, to determine these types of models.
Shamanth: Sure, sure. And I think that’s a useful, simplistic model, which certainly you can add more variables to; one, if one goes back today, 10 conversion values in the next few days. And obviously, you can layer on geos, not to mention, sources, campaigns, subject to the privacy threshold—which is a whole separate conversation altogether.
In the approach you described, as you pointed out, are calculating probabilities. There is going to be some amount of inaccuracy here. So, what are some of the big drivers of accuracy and inaccuracy? And in what situations do you find: “OK, this is a good predictor; this is a good way to make decisions.” What situations is this a bad way to make decisions?
Chris: We’ve been testing a few of these models in the last, I would say, 3-6 months with actual data and developers, and we’re in this period of time, where we still have the benefit of using IDFA tracking. So we were able to look at some of the error rates on various types of models that we built here. It can go as high as 10% discrepancy, but we find that that’s actually pretty good with various granularity, for example, conversion value is one type of granularity.
But you can also start to layer in other things within the conversion value, like assigning 2 bits for a certain type of signal that you want to give. I know that some developers have used, even more explicitly, install data as a specific driver for a part of those bits that are allocated to you.
So that can help you with attribution as well—we haven’t personally tested those types of models. There’s also the geos that come from the device, and then also sometimes you can start to layer in some of the other dimensions as well, like site ID, that are given to you. That’s actually one of the newer things, I think, that are introduced were the site IDs—or actual app store IDs now. That can change the way things work as we know; some of the ad networks out there obfuscate their site IDs. And this can actually help in some ways.
One thing to also point out: we’re talking about probabilities here. This is very different than what the industry has started—they’ve rehashed this idea of fingerprinting as probabilistic. This is not the same type of probabilities that we’re talking about. We’re talking about building probabilities on top of what’s available by Apple, as opposed to just fingerprinting and the old style of tracking and being probabilistic with that. That’s something to also be mindful of.
Shamanth: Sure. I think it’s important to qualify probabilistic is not fingerprinting. And I know you talked about some of the inputs that go into these models. As of just now, the SANs—just Facebook and Google, at the very least—are not going to share the postbacks with MMPs. And Apple search, ironically yet interestingly, is not going to use SKAdNetwork. Does that mean that in an attribution modelling paradigm, these sources are going to be less accurate than the sources that actually do have SKAdNetwork postbacks?
Chris: Accuracy is actually hard to justify at this point, for a variety of reasons, because everything is modelled. The whole industry has bought into matching last clicks to the install, and to a certain extent that also is modelled. So it just became standard that that was the case, but if you really think about it, that is a model in and of itself. And so what we deem is accurate is up to the marketer.
But I can say that, there are going to be differences in the way each channel can be modelled. So, for example, the SANs who expose less of the SK postbacks, they will expose some of the aggregate data. If you were to visit the Google dashboard, they would show you: “Oh, you got this many conversion values from this campaign.” You could essentially use that data to model off, to try to mesh it together with the data you have about your LTVs. And you can allocate it appropriately.
This can actually all be done in Excel today, where, let’s say you see a specific campaign has 10 conversion values of conversion value one, you can still do that same type of math, if you know that LTV for that specific date.
Shamanth: So there’s gonna be some aggregate data still available, around the conversion values for the SANs. So it’s not like it’s totally high level, totally black box, per se. And I know you talked about: “Look, even last-click is a model.” I don’t want to put words in your mouth, but I think you implied that there’s no such thing as accurate. I’m reminded of a quote—I forget which book this was, or who said this: “All models are inaccurate; some models are useful.” And I may be butchering that.
Chris: That’s a good quote! I’ve never heard of it. But yeah, it’s kind of like that.
Shamanth: Exactly. I think that’s a good point to keep in mind, because, as you said, with last-click everyone said: “Hey, that is the way to go.”
I think the question to ask is: is attribution modelling, with the help of probabilities, more useful than just doing SKAdNetwork blindly? I think that’s what I’m hearing you say, and imply.
Chris: Yeah, I think that we certainly see it in our tests with our developers that it is useful. There’s also a quote: “The one-eyed man is king in a blind world.” And so, you may not have that same level of confidence, but you still have a general direction that you’re shooting for.
There are other things that people have done in the past, such as very simple regression modelling the sales track: roughly in the same way to mix modelling and incrementality, all of these types of solutions, where you run a campaign, you spend a bunch of money, you get some installs, and you see a correlation to sales or revenue.
What we’re developing here does have some of that similarity. It’s somewhere in between this last-click type of deterministic modelling and mixed media type of incrementality modelling. So I think
the recommendation is you have to have your eye on each type of model, and just the business in general. If you see sales go up in general, and you can correlate it to certain characteristics, it’s helpful. And if not, then iterate on what the best solution is for you.
Shamanth: Yeah. And I think that’s the sort of conversation I’m having with people as well lately, because the media mix modelling is not right for a lot of companies. It’s what P&G uses and I will also point out—tell me if you would agree—if an advertiser is very small, they’re running one channel, would it be accurate to say attribution modelling is not really the best fit? Should they just use that one channel with the SKAdNetwork postbacks and make all of their decisions off of that, or would you disagree with that characterization?
Chris: Yeah, I would say so. If you’re just using one channel, use all of those tools in that channel simply because, one, it’s easier; and two, they’re likely optimised for whatever you’re trying to do. So yeah, if you are solely spending on Facebook, for example, and you have no plans to introduce other types of channels, what’s the point of modelling anything? Just use Facebook’s system.
But I would also say, when we talk about starting to introduce other channels, it is very important to try to model the behaviour and the interactions between each of the channels, as best as you can—which is, as you say, like what the old school style, P&G mixed media modelling is about.
I would say—specific to, at least, our types of clients who are more on the gaming side and have much earlier LTV thresholds—you need a different type of modelling than mixed media modelling, simply because mixed media modelling takes too long. You’re running these massive regressions and that requires lots of data to draw conclusions. And so, that’s why you need something in the middle between what IDFA was, and this mixed media modelling, incrementality type solution.
Shamanth: Yeah, just to also double click on some of the things you said about mixed media modelling is great for the P&Gs. Help me understand what about, let’s just say a typical gaming company, makes attribution modelling suited for them?
Chris: Yeah, sure. Gaming companies look to understand their acquisition channels a lot faster. Their LTVs tend to top out much faster for users in their games. As the extension of the LTV goes farther into the future, it’s harder to predict or understand that behaviour, where it’s going to go.
Procter and Gamble, when they run a TV ad, it’s not like someone just gets up and starts buying Procter and Gamble things. They have to go to the store, maybe it crosses their mind a year later, and that’s when they need to try to correlate those things. But for games, you download the game, and then it’s happening right in front of you. That type of LTV is a lot faster, and so you want to be able to have a faster turnaround than something like a mixed media model.
The casual gaming space might be in between, where you might see a Rovio ad—because Rovio users maybe spend money, they have a longer life cycle, their retention is much higher in the yearly type of community—whereas you look at a hyper casual game, everything happens within 14 days, 30 days.
Shamanth: You could argue a lot of things happen within the first 24 hours, and a lot of the signals do get captured by SKAdNetwork but that’s not the complete picture. So what I’m hearing you say is that because the feedback loop, so to speak, of the LTVs is relatively short for the vast majority of consumer apps—not just gaming, I would argue—certainly the vast majority of subscription apps, a lot of signal happens on day 0, even though the LTV plays out over many months. So I can see why this sort of model is certainly more suited.
Just to stay on the track of ‘this is a model’: How does attribution modelling differ from multi-touch attribution? Obviously, we know and understand the differences—and I know the folks will understand—but talk to me about why not do multi-touch attribution; or when does my multi-touch attribution make sense? When this attribution modelling makes sense?
Chris: I think what we’re seeing is that SKAdNetwork has really taken over some of the deterministic parts of attribution. They’re the ones actually determining whether or not an ad network gets credit for something based on its own methodology, which right now is last-click, but I think they’re also going to introduce impression-based type of modelling as well.
Multi-touch, I think, was always this thing on the horizon, where it’s like: “What if you have a TV ad?” And like: “What if you see this thing over here?” You have a lot of different touch points that cannot just be accounted for, and how do you factor that in? So I think what’s happening here is SKAdNetwork has a bunch of signals that it gives you based on whatever it thinks; the networks have a bunch of signals based on whatever it thinks; and you may have your own signals based on whatever you think. So you’re just building a model of whatever’s available to you. I don’t know if that’s actually multi-touch, but it’s just using whatever’s available to you, as fast as you can.
Shamanth: I see, what you’re saying is what is available is limited compared to what you might need for multi-touch?
Chris: True multi-touch, yeah, exactly. And it’s also limited by what may or may not happen with respect to Apple, how they view privacy and all of this stuff down the road. They’ve basically taken a huge chunk of deterministic attribution, and they’re actually pushing the industry to think about things beyond just that. They’re thinking about all these other signal-based approaches, which essentially is multi-touch, but it’s not explicitly determined by the industry in any sort of way.
Shamanth: Right. In the way that you described attribution modelling, for an app developer or mobile app company that wants to build this in house, what does that roadmap or approach or plan look like? And also, is there a lightweight way that they might build an MVP, maybe just in an Excel?
Chris: Absolutely, it can be done in Excel. The simplest way to describe it is you download a bunch of aggregate data and allocate linearly, based off of what’s been downloaded, to your LTVs. That, in itself, is a type of model and you’re using data that is an aggregate form.
I think more sophisticated models include getting raw SK postback data—getting access to whatever data you can get your hands on in terms of what is allowed by Apple—and then just modelling your user behaviour alongside of it. Ironically, where we were in this IDFA state before, you now need a lot more gusto with your own modelling, and data analytics and science than ever before. So it’s not like you actually go back in time; you actually go forward in time, in some respects that you have to think harder about these problems.
Shamanth: Right. I also like that you describe the simpler version of this. So yes, you can get a lot more sophisticated and accurate. But a basic V1, as you described, could happen with an Excel sheet. For people who want to get a taste of what that could look like, can you elaborate on what that approach could look like? So if people wanted to say, see for themselves, a V1 or an MVP, if you can elaborate on that process, that’d be great.
Chris: Yeah, sure. Let’s say you’re running a campaign on Google, and let’s say Google allows you to download a CSV with a bunch of your conversion values, your campaigns and even your geos in some cases. You would see how many conversion values came in for a specific geo, a specific date and a specific campaign. Then you would also look at your actual data from your LTV side of the equation, and just allocate based on those numbers. We had 10 conversions in Russia for this conversion value, 20 in the US about the same conversion value, and then we have X amount of users in both of these, so you can allocate based on those types of heuristics. You could come up with LTVs that are tied to a campaign, but at an aggregate level, which is exactly what Apple’s after. You can still do your LTV math, and all of your estimations and marketing at an aggregate level, just with user privacy.
Shamanth: Certainly, I think that can be a powerful approach to work with what Apple wants, and still get a lot of visibility; and also get a taste of what things could look like if they had the sophistication to actually use the postbacks and build out a more sophisticated model.
Chris: I think one of the biggest fears I’ve seen so far is just that people think that LTV and ROI, and retention are all going away. If you look at the surface of what SKAdNetwork provides, yes, that’s true. The way things were done before, certainly those metrics go away.
But if you look at what you’re given in terms of what you can model after? They don’t actually go away, they just become estimates, and they become models. And you can iterate to a place where you can feel comfortable using certain channels more than others.
I do think that’s the part of reinvention of this industry that is an opportunity for a lot of developers who take that challenge on. Because as we all know, from the beginning of all this, the developers who actually solve that piece faster, end up being the top one percentile. They’re able to figure out their marketing and judge their ROI and ROAS still. And the ones who sort of just back down and say: “I’m flying blind. I’m just going to lower all my CPIs or CPMs.” You’ll get taken advantage of.
Shamanth: The flip side of that is some would also look at this as a risk. But I hear you: it’s how people navigate that risk that can sometimes make a difference.
Chris: My prediction is just that CPMs in the short term; they may come down purely out of fear. But in general, it’s not like people don’t want to play games. Users still want the content, they still want to find it, etc. So there will be a period where some of these developers actually figure it out. And then they’re willing to bid higher than those others, and get more distribution profitably, etc. So there’ll just be more volatility in the CPMs over time. I think targeting is layered in there somewhere, where your targeting gets destroyed in some of these networks—which is a different problem—but if you kept targeting constant and all these other factors, you’d have more volatility.
Shamanth: Certainly, yeah. We are moving into a more uncertain world, but we have some models to guide us, some direction to guide us.
Chris, this is perhaps a good place for us to wrap. I certainly have had a lot of ideas that are food for thought. Certainly things to think about, certainly things to contemplate as we go into this uncertain, short term future. But before we wrap, can you tell folks how they can find out more about you and everything you do?
Chris: Sure, you can just simply drop me a line at cfarm [at] tenjin [dot] io and we can take it from there.
Shamanth: Excellent. We will link to that, and certainly we will link to Tenjin and your LinkedIn, so people can check you out and connect just as well. Wonderful, Chris, this is a wonderful experience. Thank you so much for being on The Mobile User Acquisition Show.
Chris: Thanks Shamanth.
A REQUEST BEFORE YOU GO
I have a very important favor to ask, which as those of you who know me know I don’t do often. If you get any pleasure or inspiration from this episode, could you PLEASE leave a review on your favorite podcasting platform – be it iTunes, Overcast, Spotify or wherever you get your podcast fix. This podcast is very much a labor of love – and each episode takes many many hours to put together. When you write a review, it will not only be a great deal of encouragement to us, but it will also support getting the word out about the Mobile User Acquisition Show.
Constructive criticism and suggestions for improvement are welcome, whether on podcasting platforms – or by email to shamanth at rocketshiphq.com. We read all reviews & I want to make this podcast better.
Thank you – and I look forward to seeing you with the next episode!