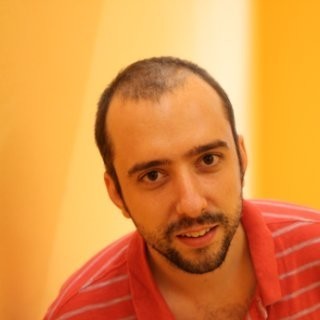
Our guest today is Pau Quevedo, Lead (Programmatic Trading) at Goodgame Studios. We’re thrilled to have Pau today because he’s had tremendous success with programmatic both on web and mobile platforms, and we couldn’t think of anyone better to speak to about how the programmatic ecosystem will be impacted by the impending IDFA deprecation.


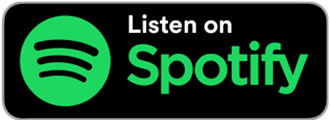

ABOUT: LinkedIn | Goodgame Studios
ABOUT ROCKETSHIP HQ: Website | LinkedIn | Twitter | YouTube
KEY HIGHLIGHTS
💪🏽The main drivers of performance on programmatic.
⚖️The differences between self-served DSPs, managed DSPs, ad networks, and bidders.
🤩The most important piece of information in a programmatic bidstream.
📝The 2 paradigms in programmatic: probabilistic and deterministic.
🖥Machine learning models significantly lag IDFA-based deterministic models.
⛔️Why in-housing programmatic can be a huge challenge.
🤝What questions Pau recommends asking a potential programmatic partner – and what is the most important question in a pre-IDFA-deprecation world.
🤓How Pau’s experience buying programmatic media on the web can inform how things might change with programmatic on mobile post IDFA.
☠️How web advertisers have coped with the upcoming deprecation of third party cookies.
👀The most important challenge for web brand advertisers in a post-cookie world.
👤How first party data can be useful in a post-IDFA world.
📈How CPIs and CPMs might change post IDFA-deprecation.
KEY QUOTES
Performance on programmatic
The main driver of performance nowadays, in my opinion, is the device graph that partners have. So for self DSPs, as I was mentioning before, it has been historically really hard for them to create a device graph compared to the managed services. And that has been one of the main challenges actually — making self-serve work.
Key difference between managed and self-serve DSP
In the self-serve, what they do is that they have machine learning tools that actually take data points made from your own campaign. They model those data points in order to estimate conversion rates or a CPA, and then they bid accordingly to that. That would be the main difference.
The truth is that the self don’t use the device graph because they don’t profile the IDFAs like the managed do.
Bidder programmatic landscape
There’s another solution, which is the bidder. The main difference with the self platforms is that the self platform, you will get an algorithm that has been trained by the different advertisers that are advertising on this DSP. Whereas if you go to the bidder, your algorithm will only be optimizing and getting knowledge based on the data of your campaign. That is the main difference between them.
Models will change post-IDFA
The way that it’s going to be now is that until now we have had these machine learning models in which they told us “Oh, in the bidstream, you get a bid request with 150 keys, 200 keys of information.” And then we model those. But we know that the most important piece of information was the IDFA, which was the deterministic information. We knew something about this IDFA, whereas all the other ones, although they are deterministic, because it’s an iPhone, that I believe that the iPhone will pay is a probabilistic one.
The challenges of an in-house system
The truth is that I find a very, very, very small number of advertisers that actually are running at scale UA in a self-serve platform. It’s really hard to find them unless there are those that are having very strong IPs. But for all the others that are in pure DR Marketing, DSP in housing is a real challenge.
Compare with context
David Phillipson says it’s not that you fail when you run self DSP. The problem is what you compare it to. We are comparing to Facebook — we are not going to be there.
How to select a good DSP
The important thing would be if we are now shifting to an ML environment where they are going to be modeling the bid stream data, you want to have first of all that they are listening to as much data as possible. That means the QPS, the queries per second, that the DSP is connected to. How many bits are they listening to per second, and you want to have as much as possible. Secondly, you want them to have all the other advertisers that are similar to you so that they have algorithms that are trained in similar advertisers to yours.
Questions to ask a DSP
You can ask them questions like, how often do you refresh it? What kind of a regression model are you using? It can give you some insight. Or how many keys are you actually modeling? Or how do you go from the install to the payment? How do you move down the funnel? How does your algorithm take that into account? Some of them go event by event, some of them what they do is they aggregate the different events, and they blend them. You can ask those questions, but the most important one until now has been is it user level data or not? And that will probably go away.
Types of keys in the bidstream
There will come stuff like what device it is, what brand it is, what’s the size of the screen. How many megabytes of RAM do they have? Even how much space would they have left on the phone? Depending on the vertical, you will be interested in different ones. Let’s say you’re in the food industry, you might be more interested in the location exactly — the GPS. For gaming, particularly, we’re interested in the device, time of the day, publisher. We model around 6 or 7. That’s what I’ve done until now. I found it to be not that much, I would have hoped to model more. The more you can model, the better, but around those 5 or 6 are the ones that we’ve been doing.
5 DSP solutions for post-IDFA
So in the web environment, they are coming up with 5 solutions. One will be to go back to contextual. One will be first party data. The other one will be working out some sort of SSP DSP collaboration, and we can make it to actually pass more data that we can actually mix and match. I heard there are similar approaches in the mobile vertical, I heard about that.
Then they have the panels, which I’m not very familiar with. It’s more for big companies. Then they have that Google recently announced that through ML, they’re able to sync when an iOS conversion has actually taken place, they can actually attribute that through ML.
There is a reason advertising flourishes
There is one fundamental thing in the industry, the internet industry, and that is that content is being paid for by advertisers. I don’t care what happened with the IDFA — that is something that is there. People are not willing to pay for content. They’re willing to see ads instead of paying for that, so if we agree that that’s like the gravity of the whole internet, that will apply here still.
That means that if I want to make more money out of my content, I also have to work on who is watching that content. How do I classify them? This will also mean that bigger companies will have a bigger shot at it. We know from the web that all these news companies, now they’re making a big umbrella, so they can have all the first party data united into one. They can do their retargeting, and they can do the selling in a much more efficient way.
Shamanth: I’m very excited to welcome Pau Quevedo to the Mobile User Acquisition Show. Pau, welcome to the show.
Pau: Hey, Shamanth. Thanks a lot for inviting me.
Shamanth: Absolutely. Definitely thrilled to have you because when we’ve spoken prior to this recording, I’ve found a lot of what you’ve said to be very insightful, and of course you do come very highly recommended. For those reasons, I’m thrilled to dive into how programmatic is evolving and how it could change come September, come the post-IDFA regime. All of this is what I’m excited to dive into with you today. A good place to start perhaps would be this: what would you say are some of the key challenges that marketers face while doing user acquisition on programmatic today?
Pau: Well, programmatic means basically the automation of advertising. So inside the programmatic ecosystem, we use the DSP tools in order to buy traffic. There’s basically 2 types of DSPs that we call managed and self. Right now, the biggest challenge that we see in the industry is to actually make self work as managed does. With managed, you’re able to compete with the duopoly and other players, but for self has been a challenge for some. Some companies actually try to in house fully the activities — even building their own bidder. And eventually, they realized the difficulty that it brings, so they moved into a retargeting solution for the programmatic. So, programmatic has been working largely on the web space or retargeting and for branding — performance was a bit harder.
In the mobile ecosystem, we work mostly with performance, so that’s a bit harder as well. Inside that chunk of performance,
the main driver of performance nowadays, in my opinion, is the device graph that partners have. So for self DSPs, as I was mentioning before, it has been historically really hard for them to create a device graph compared to the managed services. And that has been one of the main challenges actually — making self work.
Shamanth: For clarity and understanding, can you define what a self-serve DSP is? How does it differ from a managed DSP, and why a self-serve DSP would not have a device graph?
Pau: The difference between the two goes one level above. The difference between an ad network and a DSP would be the ad network network is selling on a CPI and the DSP is selling on a CPM basis. Between the managed and the self, both will be selling on CPM. CPM is the one that dictates if you’re buying a DSP in a programmatic environment. Out of those two, the main difference would be that in the managed, they actually are running the campaigns themselves. You just upload the creatives and send a couple of emails and your payouts, and they adjust it. They will run the whole show. And you would get some sort of learnings in terms of their transparency, where they’ve been serving what’s pub ID, and maybe which exchange.
Whereas in the self, you will be doing everything on your own. And theoretically, the main difference is that the managed, what they do is, they create a device graph, which means that they understand the IDFA of what each device is doing. And then they would know who is paying now of the different IDFAs.
Whereas in the self, they’re not doing that. What they do is that they have machine learning tools that actually take data points made from your own campaign. They model those data points in order to estimate conversion rates or a CPA, and then they bid accordingly to that. That would be the main difference.
The truth is that the self don’t use the device graph because they don’t profile the IDFAs like the managed do.
And maybe, because if you are actually going to be running your own campaigns, if you’re using a device graph, what’s the point of targeting? I don’t care which app I’m targeting because it’s all about the user.
It’s hard for us to say, “Oh, I’m going to run a self device graph” because the device graph is actually doing the job. It’s doing the full targeting and everything.
Then there’s another solution, which is the bidder. The main difference with the self platforms is that the self platform, you will get an algorithm that has been trained by the different advertisers that are advertising on this DSP. Whereas if you go to the bidder, your algorithm will only be optimizing and getting knowledge based on the data of your campaign. That is the main difference between them.
Shamanth: And when you say in-housing your programmatic does that involve running on a sensor platform or running with a bidder or it could be either?
Pau: It could be either. The truth is that fully in housing programmatic is the bidder. That’s the real truth. But we are fine as long as we feel that self, the self platform could be like an in housing solution. The real truth is that the bid is one that gives you full control. Managed, you don’t get control, but you don’t get risks because they are running the whole show. You move into self, you have a bit more control, but you have a bit more risk. The next step would be to get full control but full risk, and it’s very hard because normally in the bidders, they don’t give you an algorithm, you have to basically tailor it on your own because you have to customize it. That’s only for big companies, or if you actually have a need for it.
Shamanth: Right, and now, starting September, the device graph is going to go away. So how does this ecosystem that you’ve just described, how does it change between managed, self-serve, and bidders?
Pau: A very good question and a tough one. I’ve actually tried all 3 formats. In my journey, trying to in-house programmatics, what I’ve basically done is I’ve been comparing those 3 elements. So I have an idea of what is the difference? The difference is basically that the managed with the device performs way better. The other ones don’t — that’s a clear one.
The way that it’s going to be now is that until now we have had these machine learning models in which they told us “Oh, in the bidstream, you get a bid request with 150 keys, 200 keys of information.” And then we model those. But we know that the most important piece of information was the IDFA, which was the deterministic information. We knew something about this IDFA, whereas all the other ones, although they are deterministic, because it’s an iPhone, that I believe that the iPhone will pay is a probabilistic one.
There’s 2 environments: probabilistic and deterministic. Right now, some of the managed are more into the deterministic, even the Facebook guys. The self are more into the probabilistic because we don’t understand this IDFA. What the next step will be for the managed ones is that, first of all, they have very robust models. They have the IDFA, they understand the other set of strings and other set of keys that come along with that string. So for some time, they will be able to still perform because their models will take time to wear off — that’s obvious. They will do whatever they can by enriching them with fingerprinting information and with the 20% of people who would actually accept those prompts on Apple. They will somehow manage to make those models live longer. But at some point, they will die off. So what’s going to happen then? Then, they are going to have to go and model all the other keys that are within the IDFA. In that moment is when we are all in the same level. So in that moment, we can all say “Okay, now we have to model with our ML techniques, and then we’ll see who is better one or who is able to have more advertisers, get more data, and model better.”
That would be a solution, but I believe that there will be some sort of tracking solution that we will not get there because the truth is, that in modeling just those key elements that you get in the request, it’s gonna be really hard to compete against someone who actually knows the payer like Facebook, so the gap is gonna get way bigger.
Let’s see what happens. In the industry, I’ve been doing work for some time, I’ve been following people like Ari Paparo, and I work with Beeswax as well. In the industry, some people are saying that the whole ML modeling still has to be proved that it works because until now, we’ve been running mostly with the IDFA. But let’s see now what really happens when it’s all pure ML. I still have to see that because I’ve been running those products like a bidder, and it just was so bad in performance compared to the managed ones with the IDFA. There’s such a big gap that I don’t know. They still have to prove it, honestly.
Shamanth: Would you say that the self-serve and the bidder solutions were so bad that, for the vast majority of advertisers, they wouldn’t even work? Would it even be ROI positive? Or do you feel like they’re bad, but they could be a part of the mix going forward?
Pau: I believe those will work for retargeting. But if we only focus on UA, I believe you can get some pockets. You can find some stuff. Before one of the pockets was LAT users because actually you can target those and use fingerprinting — lower CPM. That’s going to be everyone now, that was one of the pockets. An app, an iPad, or maybe doing some sort of analysis of the day parting or playing around smartly with waterfalls within the different publishers. There were some caveats that you can actually use, but they were not scalable.
I have, at least in Europe, good connections with some other gaming companies and other devices regarding programmatic.
The truth is that I find a very, very, very small number of advertisers that actually are running at scale UA in a self-serve platform. It’s really hard to find them unless there are those that are having very strong IPs. But for all the others that are in pure DR Marketing, DSP in housing is a real challenge.
Shamanth: And it’s gonna get much harder.
Pau: It’s gonna get much harder, but at least I mean,
David Phillipson says it’s not that you fail when you run self DSP. The problem is what you compare it to. We are comparing to Facebook — we are not going to be there.
Before, if I went to my CEO and said, Look, this sub DSP brings us this ROI, this managed brings us this ROI — but managed, what is this? Now, in let’s say 6 months, when the models wear out of the managed ones, I think self DSPs are gonna have a bit of the same situation as the managed and maybe we have a shot.
Shamanth: That definitely makes sense. The gap is going to reduce, and it’s going to be a more level playing field as we go forward it sounds like. And obviously, what’s going to become critical going forward is how you evaluate your partners because that device graph or the past experience becomes much less relevant. So how do you recommend that marketers should evaluate potential programmatic partners going forward? What might be some of the questions they might ask in a post IDFA world to form this evaluation?
Pau: In the pre IDFA world, our main focus when we actually onboard a partner and actually talk to them is to find out if they’re doing the device graphs, if they are looking at user level data, to what extent. How many keys of the log-level data are they actually modeling? Are they only doing contextual? And that was one of the main questions. And right now that one’s out, so that we understand the importance of that one.
Now, normally people would ask stuff like, what are the analytics or reporting or the campaign setup? I don’t really care about any of that, as long as it performs.
The important thing would be if we are now shifting to an ML environment where they are going to be modeling the bid stream data, you want to have first of all that they are listening to as much data as possible. That means the QPS, the queries per second, that the DSP is connected to. How many bits are they listening to per second, and you want to have as much as possible. Secondly, you want them to have all the other advertisers that are similar to you so that they have algorithms that are trained in similar advertisers to yours.
Those would be the main elements.
It all will depend on the algorithm. They can tell you whatever they want about how the algorithm is better than the others. At the end, you have to test it because it’s really hard to know.
You can ask them questions like, how often do you refresh it? What kind of a regression model are you using? It can give you some insight. Or how many keys are you actually modeling? Or how do you go from the install to the payment? How do you move down the funnel? How does your algorithm take that into account? Some of them go event by event, some of them what they do is they aggregate the different events, and they blend them. You can ask those questions, but the most important one until now has been is it user level data or not? And that will probably go away.
Shamanth: When you speak of the other keys in the bidstream that they can model their performance off of, can you share any examples of what some of these parameters might be?
Pau: Absolutely. Normally, in this one, it will come from the information of the impression —
There will come stuff like what device it is, what brand it is, what’s the size of the screen. How many megabytes of RAM do they have? Even how much space would they have left on the phone? Depending on the vertical, you will be interested in different ones. Let’s say you’re in the food industry, you might be more interested in the location exactly — the GPS. For gaming, particularly, we’re interested in the device, time of the day, publisher. We model around 6 or 7. That’s what I’ve done until now. I found it to be not that much, I would have hoped to model more. The more you can model, the better, but around those 5 or 6 are the ones that we’ve been doing.
Shamanth: Yeah, in some ways this is going to become much like your SDK networks. Or would that be inaccurate to assume that?
Pau: That’s interesting because somebody was mentioning that the other day. It will be very similar to the SDK networks at first, but the SDK networks work on a historical weekly CPM. So historical net, how they do this is that they calculate what they earned last week, and they set up that as the CPM for this week, and they are not real time. And they’re not going for each user, so they actually don’t really care, the log level data, in that regard. They don’t use them. They might use some keys like phones, carriers, or device phones or something like that, but they don’t model out that much, and they are not useful because they go into packs. So it’s not exactly like that, but they do have a point.
Shamanth: I think that similarity definitely does stand out. You do programmatic buying on the web, and mobile, so how does your experience buying programmatic media on the web inform how things might change with programmatic on mobile post IDFA.
Pau: Well, in the programmatic web, this was announced earlier. They actually said look in March 2021, third party cookies will be out, you guys have to find a solution. They didn’t eradicate it because what has happened here is different. The solutions there are mostly circling around the publisher. They want the publisher to actually enrich their first party data, to structure the first party data. That way they can send signals to us that we know that the user is valuable, not because we know the user, but because the publisher says so.
So in the web environment, they are coming up with 5 solutions. One will be to go back to contextual. One will be first party data. The other one will be working out some sort of SSP DSP collaboration, and we can make it to actually pass more data that we can actually mix and match. I heard there are similar approaches in the mobile vertical, I heard about that.
Then they have the panels, which I’m not very familiar with. It’s more for big companies. Then they have that Google recently announced that through ML, they’re able to sync when an iOS conversion has actually taken place, they can actually attribute that through ML.
In the web environment, what is mostly worrying the advertisers is, apart from the retargeting, which is going to be complicated, it’s the frequency capping — that’s the most and the largest element, and they need the cookies in order to understand the frequency. For branding campaigns that is extremely important. So Google said that they can actually, through ML, determine the frequency capping. So they have different solutions, but they don’t have any that works. Really the only one that actually takes on all the boxes: we’ll be able to retarget, we’ll be able to target first party data, third party data sets, etc, it still is the one that I mentioned that the SSP and the DSP will somehow communicate. It’s basically bypassing, in our case, a mobile the MMP. I’ve heard that MoPub might be working on a solution like that and other SSPs and exchanges.
Shamanth: To just to pick on one aspect of your answer, when you say first party data, can you elaborate on that?
Pau: The first party data, I mean that to go to ad monetization, I’m also in charge of ad monetization at Goodgame Studios. So to take it to our world, that would mean that we actually would have to understand our users and the same way that we actually clustered them between engagement and frequency, that’s how we end up managing monetization. We’ll do it even more, we will try to find out more about our own users.
Then when we’re going to show a rewarded video, then we can actually tell the guys “Hey, look, this user, you don’t know much about him, but we know so much.” We just give it to you through a PMP deal or something. So we actually capitalize on our first party data, which would be a way to take the power back to the publisher.
There is one fundamental thing in the industry, the internet industry, and that is that content is being paid for by advertisers. I don’t care what happened with the IDFA — that is something that is there. People are not willing to pay for content. They’re willing to see ads instead of paying for that, so if we agree that that’s like the gravity of the whole internet, that will apply here still.
That means that if I want to make more money out of my content, I also have to work on who is watching that content. How do I classify them? This will also mean that bigger companies will have a bigger shot at it. We know from the web that all these news companies, now they’re making a big umbrella, so they can have all the first party data united into one. They can do their retargeting, and they can do the selling in a much more efficient way.
That is eventually what will happen in the post-IDFA world is that the big companies are going to be benefited in comparison to smaller companies.
Shamanth: When you say first party data, that could be CNN saying, “Aha, this is 1 million of our most engaged users. We won’t tell you who they are, but we will let you target them.” Is that roughly how it would work?
Pau: Exactly, and we will put you on a different floor, we’ll set up a PMP deal, and we’ll do business.
Shamanth: But you won’t say who they are. That makes sense, and they would probably have a different unique identifier like email IDs internally.
Pau: Exactly, CNN will understand who their users are, who is more valuable to them, and then they would actually tell us, “Hey, you want the best one? Lookalike at 10 bucks CPM.”
Shamanth: Got it, and on mobile, something very similar could happen just with IDFV.
Pau: I don’t know exactly how we would be with a PMP deal, but I think we are moving towards that kind of industry. You can actually do that, we can actually talk to another publisher and tell them, “Hey, I want you to put me on top of your waterfall,” and you can cut deals. That would be the idea now.
Shamanth: Interesting, and you spoke about how content is paid for by advertisers, and that’s the essential reality of how the ecosystem works. Now that the advertiser has less of a guarantee of performance, without the IDFA, the performance is getting worse. How do you think that changes CPMs and conversions, or just the broad advertiser ecosystem, in the short term and long term?
Pau: Moving from a deterministic to a probabilistic, what we’re doing here is we’re introducing noise into the decision making. The moment we have more noise, if you look at the CPI. The CPI is basically a mixture of the CPM and the CVR. So if we look at the CVR, I can expect that we don’t know the conversion rates of the user so much.
We don’t understand the IDFAs so much, we only understand the publisher where it is being served. So I think CPIs go up because we have less information on the user. But on the other hand, I believe CPMs will go down because as we know less from the users, we will not be able to be so high on them because we don’t know them right now. CPMs will go up because “Hey, I know this IDFA is good. I don’t care if I’m bidding at 80, 90, 100 bucks — I’m bidding on it.” Right now, we don’t have that, that will bring a down trend in the whole CPM industry. I also believe CPIs will go up because of what I just said, because there’s more noise into the auction regarding the knowledge of the users, so those two elements I want to see. I believe CPM will go down and CPI will go up, that means that the effect of the noise in the conversion rate will now be higher than the drop in the CPM.
Shamanth: Yeah, and very many variables very many things will shape up over the next couple of months, as we will see. Pau, this was fascinating. I certainly learned a lot, and this is certainly something I’m going to think about as we get closer to September. This is perhaps a good place for us to start to wrap up. As we wrap, can you tell us how people can find out more about you and everything you do?
Pau: Yeah, I work at Goodgame Studios. And you can reach out to me on LinkedIn — Pau Quevedo. I’m always open to a nice talk about UA and sharing. I believe that one thing that was mentioned before is that you’ve mentioned how we should pick a partner in this post IDFA world. One of the things I forgot to mention is that I am actually looking for a partner that will actually help me navigate this madness. I’m looking for someone who’s going to be prepared for this, who understands, and who I can partner with. One of the big issues that I found with the DSPs was that they had a lot of focus on ML and the algorithm, but they didn’t focus on the supply chain. Understanding what’s going on here, the supply path optimization, that kind of thing. I believe that partnering with someone who understands the ecosystem the best will probably help you get better after this storm that is about to hit us. That’s one advice I would give is don’t just try to partner with someone, try to learn with them, try to make them learn with you. And that’s probably my best advice.
Shamanth: Indeed, and we will, of course, put all of this out in the transcript and the show notes. For now Pau, thank you so much for being on the show. Excited to put this out into the world very soon.
Pau: Thanks to you, Shamanth.
A REQUEST BEFORE YOU GO
I have a very important favor to ask, which as those of you who know me know I don’t do often. If you get any pleasure or inspiration from this episode, could you PLEASE leave a review on your favorite podcasting platform – be it iTunes, Overcast, Spotify or wherever you get your podcast fix. This podcast is very much a labor of love – and each episode takes many many hours to put together. When you write a review, it will not only be a great deal of encouragement to us, but it will also support getting the word out about the Mobile User Acquisition Show.
Constructive criticism and suggestions for improvement are welcome, whether on podcasting platforms – or by email to shamanth at rocketshiphq.com. We read all reviews & I want to make this podcast better.
Thank you – and I look forward to seeing you with the next episode!