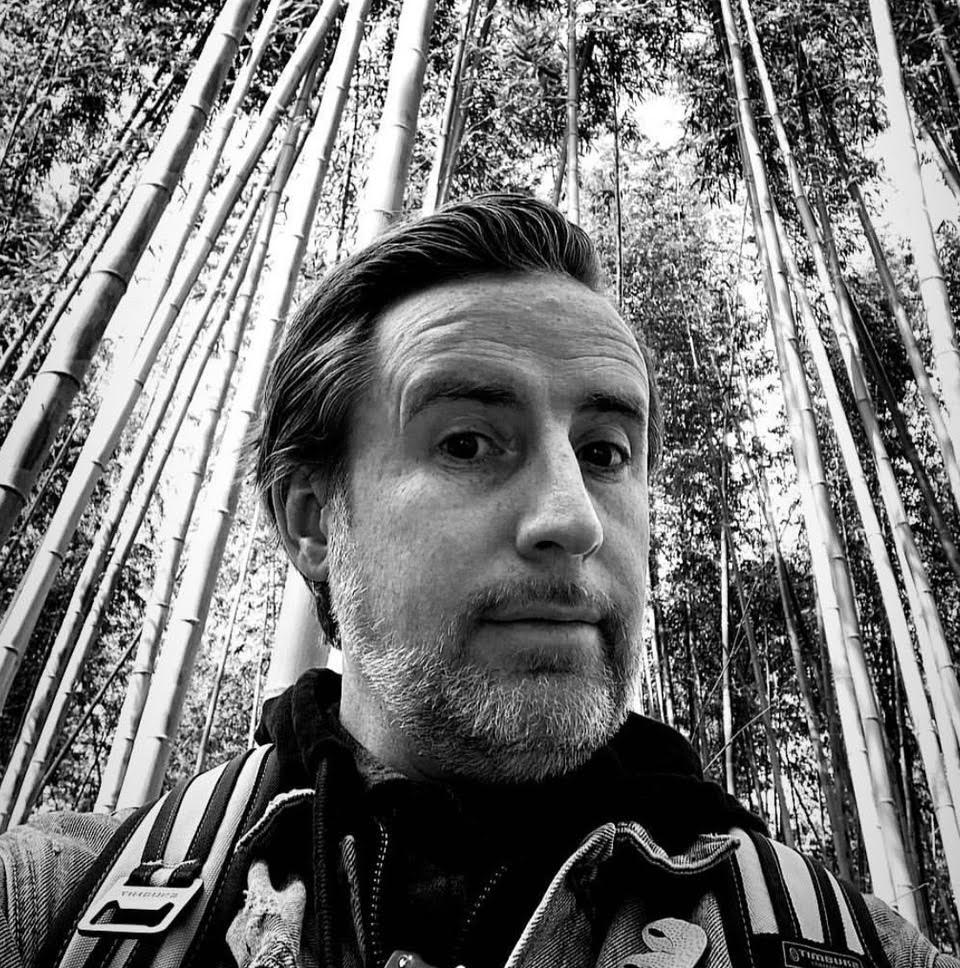
Our guest today is Warren Woodward, Co-Founder & Chief Growth Officer at Upptic.
In 2010 Warren quit his day job in the film industry to go full time into building a one-man performance marketing agency, quickly pivoting to mobile around the dawn of the App Store. He has since built marketing divisions and launched massive apps for companies like Nexon, Wargaming and Blastworks. In 2019 Warren co-founded Upptic, a company focused on providing growth services and growth automation technology to app developers.
Iโm excited for this interview because Warren describes a solution to a significant pain point for marketers – how to optimize their performance on rewarded video networks where there are thousands of source apps. Today Iโm thrilled to explore the elegant and effective approach to cut through this complexity that Warren describes.
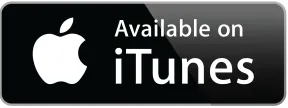
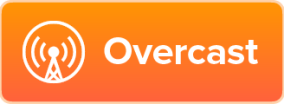
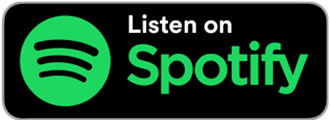

ABOUT: LinkedIn | Upptic
ABOUT ROCKETSHIP HQ: Website | LinkedIn | Twitter | YouTube
KEY HIGHLIGHTS
๐ฃ๏ธ What an engineer at a big ad tech company said that made Warren realize as to what the incentives of ad tech companies were.
๐ค What ad tech algorithms typically optimize for.
๐ What channels is it worth building your own algorithm for.
๐ The key rules that Warren recommends using for optimizing rewarded video networks.
๐ค How to find statistical significance in decisions based on these rules if data is skewed by outliers.
๐ธ Advertisers can lose a lot of money on the long tail of unexplored inventory.
๐ How a marketer should begin to approach codifying their work.
๐จโ๐ป The different levels of automation that a marketer can pursue based on their resource availability.
๐ฏ The optimal workflow – and the fallback workflow that between them can work with nearly every partner.
๐คทโโ๏ธ Do the rules of the algorithm need to change for a completely new network?
๐ Does this approach of building rules based on performance of supply pools work on programmatic traffic?
KEY QUOTES
Where algorithmic loyalties lie
There were a lot of questions from the audience and the engineer kind of stopped and said, โthereโs one thing that you guys should understand about the basic nature of the algorithm. Before anything else, itโs going to make sure that it spends your money. Once it meets that, it will see if it can meet any other goals.”
The endgame of UA automation
What we are trying to do is, take a really great UA managerโs day to day work, codify it into a set of rules, and then build scripts to run those rules.
How build data to rule out guesswork
When you start buying into a source, you have an exploratory bid. And then as you get data on individual placements, you move towards having a bid by placement. And you can set rules to develop when it moves from one to the other and using a system of weighted averages.
A stepping stone to automation
Use the APIs available by the ad networks, or even just to create an automated CSV that you send to your account rep and say, โhereโs your daily bid changes, please enact these changes.โ
Why optimization for programmatic is critical
If you just launch without any sort of optimization process in mind and one of these rewarded pools of inventory, youโre probably going to lose a lot of money. And this is because of the breadth of sub IDs and networks. Now take that and magnify it by a factor of another several thousand, like several orders of magnitude, thatโs what youโre dealing with when you go into the programmatic space.
Shamanth: I’m very excited to welcome Warren Woodward to the Mobile User Acquisition Show. Warren, welcome to the show.
Warren: Hey Shamanth, very good to be here. Thanks for having me.
Shamanth: Yeah, I’m excited to have you because certainly we’ve been in similar circles for a long time. And many many people I know and respect speak very, very highly of you. And, you know, that definitely when I last spoke to you, your very unique and interesting approach to UA definitely stood out. Definitely, there’s many aspects of that that I would love to dive into on today’s call. And today, we’re going to talk about your approach that you called โbuild your own algorithm.โ And to start off, you were inspired to adopt this approach because of something you heard in an interaction with an engineer at a big ad-tech company. Without disclosing too much about the company per se, tell us about this experience and what realization you had after you had this particular experience?
Warren: Yeah, for sure. So, anecdotally, I’m sure a lot of UA professionals had similar experience of using an algorithm provided by a network that’s like, โoh, you know, ad network X has their ROAS algorithm and you try, it just doesn’t work for whatever reason.โ So there’s kind of the sense of like, โokay, well, is it me, or is it them?โ But things really sort of solidified for me and kind of doubled down on this set of beliefs.
Yeah, there was a UA industry meeting where they brought out the engineering team that had designed one of the algorithms for one of let’s just say, one of the two major media platforms. And
There were a lot of questions from the audience and the engineer kind of stopped and said, โthere’s one thing that you guys should understand about the basic nature of the algorithm. Before anything else, it’s going to make sure that it spends your money.”
Once it meets that, it will see if it can meet any other goals. But, you know, that’s its utmost priority at the end of the day to maximize the value of the inventory sold by the network – which I mean makes a lot of business sense on their end. But it kind of rings a bell for me that this creates a clear divide of sorts of whose interest the algorithm is acting in. And kind of reinforces why any savvy media buyer needs to make sure that they are owning as much of that as they can.
Shamanth: Yeah and even though they are performance driven platforms, at the end of the day, at some level, your incentives aren’t a hundred percent aligned with this. In hindsight, it all makes sense, but a lot of us assume that these platforms are acting in our best interests, which isn’t strictly always the case.
Warren: Yeah, I was just gonna say – to kind of paint a picture of how you should think about these algorithms are working, particularly by the big players. Say that you have, let’s make an extreme situation where there is only one buyer for your product. And say that you have a budget of $10 in the campaign, you run the exact same scenario–and scenario one, you have a $10 budget, scenario two, you have a $10,000 budget. So in scenario one, the campaign runs, it knows there’s one likely buyer, the algorithm is very smart and identifies that buyer. And you say, โhey, I spent my $10 budget, I got my optimal outcome. Great. This is excellent.โ
In scenario number two, you’ve got a $10,000 budget, the same situation is only one potential buyer. The algorithm quickly acquires that buyer for you, and then it just spends the rest of your money on sort of the next least bad pool of inventory going on down the waterfall until it assures that it spent all $10,000.
Shamanth: Right. And that also makes sense just structurally how the algorithm is designed to work. And then maybe if it knows that the one buyer is somewhere in the pool, probably spend the other $9,999 first, and then come to the buyer and just say, โOh, this is your total CPA.โ And, you know, certainly the approach you advocate and you’ve adopted has been that you just say, look, considering the algorithms aren’t necessarily on our side, let’s just build our own algorithm. Let’s take matters into our own hands. What channels did you pick to build your algorithm on? And we’ll certainly talk about what the algorithm is, how you might build it, but what channels did you pick? And why did you pick these channels?
Warren: Yeah, for sure. Maybe it’s worth quickly touching on things like where you can have less effect with this, which are the major players in the space, your Facebook’s, your Google etc, where it’s a rather opaque system, you don’t have a lot of control, you do have to trust their algorithm. And in that case, I say it’s more of like, I was joking, it’s kind of like animal husbandry like horse whispering where you just have to kind of learn how the algorithm works, what inputs to give it and what that’s gonna produce back – in a way, it’s very unscientific.
But there’s a lot of pools of inventory that we say are much more dumb. You know, there’s a lot that you can still buy in a fully manual way. And for us, like the obvious place to start these explorations of building our own system was the rewarded video networks. This is like your Unity, ironSource, AppLovin, Vungle, etc. and the reason that we wanted to try to build an approach for these is a couple things. They’re all kind of the same at the end of the day, so you can take one strategy and port it to the rest. And there’s also a lot of transparency, and you can do a lot of micromanagement. You can bid for each app in those networks on an individual basis. So, the combination of scale and accessibility meant that this is where we want to start our explorations. And I guess the last factor was there were not a lot of really proven existing tools for this.
Shamanth: And because this is almost like an open market, right, and there isn’t a lot of optimization already happening, it certainly makes sense, that’s where you want to start, right. And when you say, build your own algorithm, what is the general approach? What are some of the rules that you would set up on these rules of inventory, rewarded video networks?
Warren: Yeah, for sure. So there’s a few different approaches you can take here. There are a few players that are doing really interesting work in the space. One company that I do like is a company called Bubbleye, and they’re doing a very ML based approach to buying on these sources. And it can work pretty well. We wanted to make something that was a little more bread and butter that could take kind of past learnings and implement them on future campaigns. So as you alluded to, we built a system based off of rules. So just coding some simple logic for how you bid for individual placements on the network, and then building a script to run that logic perpetually, to optimize your campaigns without using human oversight. So really,
what we are trying to do is, take a really great UA managerโs day to day work, codify it into a set of rules, and then build scripts to run those rules.
Shamanth: What might some of these rules be? Are there examples that you can think of?
Warren: Yeah, I mean, the one that probably everyone will get is, you know, ROI or ROAS based rules. So, looking at all of the individual placements on a network, looking at the value of the users that are coming from those individual placements, and using that to make judgments on the appropriate bid and the expected lifetime value of different sub segments. Another one that probably is universal to all apps – and I should probably clarify, when we started this, we intentionally wanted to build really basic rules that you could apply to any app that weren’t specific to gaming only or a particular product. So retention is another really common one that you can easily build rules around – like your day 1 retention, day 2 retention can be very early signals. And another one is like onboarding events like, completing a tutorial in a game or completing a free registration, another might be buying in a subscription app, for example.
Shamanth: Now, it makes sense that you could build these rules around ROAS, or retention or onboarding events, that all makes sense. Now, many of these channels have thousands, tens of thousands or more publishers and apps. And oftentimes, you can just have like a handful of purchases. Like I’ve worked on campaigns where you just see, oh, 200% ROAS, and there’s just one guy buying $500 IAPs. So, how do you account for some of these outliers? Or how do you solve for statistical significance in building some of these rules?
Warren: Yeah, that’s a great question. And it’s definitely an area where in my career, I’ve lost my share of money, like learning the hard way about how to deal with that problem. One interesting study that one of my teams did a while back while researching this area was to look at where in the networks we were losing money. Because we knew that, we were doing our optimizations, we could see, where there was significant sample size, we could say, โokay, cool, this is the right bid for this publisher, this is the right one for this one.โ And we saw that we’re actually just losing most of our money in that vast ocean of publishers that we didn’t have enough data to make decisions on.
If you’re losing so much money in the long tail, it doesn’t necessarily matter what profit you’re driving out of the top publishers because it can be drowned out at the end of the day because of the scale of the long tail on some of these networks. So, there’s a few ways that you can approach it, that we do in our own logic. One is just thinking about a system of exploration versus refinement. So
When you start buying into a source, you have an exploratory bid. And then as you get data on individual placements, you move towards having a bid by placement. And you can set rules to develop when it moves from one to the other and using a system of weighted averages.
So, if it’s like, say that in a given app, you want a sample size of 100, before you completely trust the data from that placement, you would say as you move on the spectrum for one install to 100, you can you move your bid from the default bid for exploration to the proper bid for that app. Another way that you can potentially approach this is also think about using the hierarchy of campaigns to your advantage.
So you can say, if we’ve got enough data, at this smallest level of granularity at that sub publisher level, make the decision based on that data. If that data is too light, go up one level to maybe we’re using a geo based assumption. And if that’s too light, then maybe go up one level further to your cluster of geos for a certain bid. And you can use that same system of weighted averages there.
Shamanth: Yeah. And I think it’s interesting that you mentioned that you guys were losing money, not on bad publishers, because you clearly blocked those out – but you were losing money on publishers that you just didn’t have enough data. And it was just inconclusive. And I also realized, that’s kind of crazy that on a lot of the networks, the exploratory bid is the same as your baseline bid. So you’re basically saying, look, these are somewhat proven pockets of inventory. I’ll bId x, this is somewhat exploratory, we will bid the exact same thing. And which doesn’t make sense and sounds like what you’re doing is turning that logic on its head and saying, let’s treat proven bids as sort of proven publisher inventory as separate from exploratory inventory – and just treat exploratory almost like test budgets.
Warren: That’s correct.
Shamanth: That makes sense. And, you know, that certainly explains why a lot of your approaches can be very effective. And, you know, for building out some of these algorithms, I imagine, you would require some sort of engineering and data science resources. So for a marketer that’s looking to build out an algorithm like this, what sort of resources should they look to acquire on the team?
Warren: Yeah, that’s a great question. And that’s something I really like to stress, obviously our company Upptic provides services like this for developers, but you don’t necessarily need to be dependent on a third party to do this – you can do a lot with a little. I’m a big believer in 80:20 situations like finding that 20% of work that gives you 80% of value. And it’s definitely the case when it comes to building simple automation systems for optimization. So, you can have a lot of value by simply building this set of rules that you can enact via a spreadsheet even.
And the way that you should consider starting this is to just like, try to write out your process of how you do optimization. So a lot of times people will find that they actually do have rules that they’re working with as they do their day to day work. They’ve just never exactly written them out. Semi experienced UA campaign managers can look at a set of data and say like, โoh, well, I should bid that publisher up, I should block that one, I should put this one down.โ
But they might not know exactly what logic is driving out, it’s just a feeling, it’s reps of experience. So, try to write out your own logic as a set of rules at play. You know, why did you block this one? Why did you bid this one up, and that’s a good place to start. And then once you write those rules out, you can simply download a CSV of data and use that logic to work through the CSV.
As a next step, hopefully, you have some sort of data science resource, or some sort of engineering resource, the next step would be figuring out a way to actually run that process automatically. We’ve done simple versions of this by building light Python scripts to run this process for us. And then one step beyond that is figuring out a way to
Use the APIs available by the ad networks, or even just to create an automated CSV that you send to your account rep and say, โhere’s your daily bid changes, please enact these changes.โ
Each network is a little unique as far as technical ability.
So, think of the two things is very separate, one, think about your logic and making your logic as good as possible, and you’ll get a lot of value from just doing that and distributing it to your team, getting your most junior level to be using the same logic, your most junior level employee to be using same logic as your most seasoned employee.
And then separate from that, think about, okay, how can we streamline this process? How can I make it to where I don’t have to even open up the spreadsheet, that I don’t even have to contact the ad network? And once you know that it’s working, and can actually see the results. That’s when I think you should really put a priority on automating that as much as possible. But you don’t have to have an engineering resource. And that’s what I really want to stress like you can you can do a lot with a little here.
Shamanth: So as long as you’re clear about codifying what is repeatable, bringing in the engineering resource is essentially amplifying that codification. And what do you need to have happen on the channel side to enable all of this. Do the channels need to have some sort of an API connection? How do you think about that?
Warren: So we always think of – what is our ideal state for the system to work? And then what’s our fallback, generic strategy that we can apply to a network that has no technical capabilities whatsoever. I guess the thing that you do need in any situation is a certain level of transparency. Luckily, every major rewarded video ad network does at least identify each sub publisher on the network. Some do not use an English language name, some use an ID number, that’s still fine.
You just need to identify each one uniquely. So there’s basically, two ends to this workflow, the optimal and then the fallback. So optimal is that the networks have read & write API, which you can be using to write your process directly back to the network. And then the fallback that we adopted was basically just producing automated CSV output that would be emailed to a point of contact, that could enact it. And so when we approach any network with this, we say, โhey, can we do our desired way of operating?โ No, we can’t or we can for a time being because we’re waiting for this new feature to roll out. So, we’ll use the fallback mechanism of just providing a list of bids and sending that to the network.
Shamanth: Right. And if you’re working with a new network that you don’t have a connection with, does that take some non trivial amount of work to sort of customize your algorithm for the new network, assuming it’s the same set of rules?
Warren: Yeah, so one reason that we have focused in the rewarded video area is a lot of the inventory is the same across the networks. So, there is one key area to differentiate. If you’re using traditional rewarded video, we haven’t found that you actually need to adapt your rules by network, you might need to play with sort of your risk mitigating factors like the aggression that you’re exploring to give the network for example, network A, had a ton of hyper casual inventory and you’re buying for a mid core game, you might want to have a very cautious exploratory bid. But at the end of the day, the logic that will drive to profitability should be the same amongst all networks. It’s just a question of the balancing speed of maneuvering the campaign from losing money to profitability, balancing the speed with your ability to absorb the bleeding of the learning period.
Shamanth: Yeah. And speaking of new channels, would this exact same approach work for programmatic via perhaps a DSP. Why or why not?
Warren: Yeah. So think about – we mentioned in the beginning of the call that idea, that
If you just launch without any sort of optimization process in mind and one of these rewarded pools of inventory, you’re probably going to lose a lot of money. And this is because of the breadth of sub IDs and networks. Now take that and magnify it by a factor of another several thousand, I don’t know, like several orders of magnitude, that’s what you’re dealing with when you go into the programmatic space.
So that same way that you have a lot of upfront pain when you start in a rewarded video, that’s going to be that much harder when you go into programmatic because there’s so much inventory available. So while in theory, the same approach will work there is going to be a lot more bleeding. Another reason that we haven’t put as much of our efforts in this space is there are more third party tools available, tools like Beeswax that you can use to implement your own algorithm for programmatic inventory. So yes, you can do it, it’s more painful, and the solutions are a little more advanced on market for the area.
Shamanth: Sure and I imagine, it’s also a little trickier on programmatic because you could certainly get that data at the pub ID level but the really powerful algorithms on programmatic have to happen at the user level. And I imagine that opens up a whole new Pandora’s box about user consent.
Warren: Yeah, sure and that’s a good point. And with us being a third party ourselves, we just work with developers, we want to make sure that we built a system that wasn’t dependent on holding individual user data, because that is, with all of the current sensitivities around that, we didn’t want that to be a liability for our company and our partners, potentially.
Shamanth: Certainly, certainly but the algorithm you guys have built absolutely does enable you guys to ride massive oceans of traffic that some of the rewarded video eventually presents and significantly mitigates the performance risk there. And I could see how that can be very, very effective – I can see how that is something that all of our listeners can absolutely adopt for their own apps and games.
Warren, this has been incredibly instructive. Certainly, I’ve learnt just a completely fresh perspective about how to approach what can often seem like a fairly intimidating vast pool of traffic. This has been incredible. Thank you so much for being on the Mobile User Acquisition Show. As we wrap up, can you tell our listeners how they can find out more about you and your work?
Warren: Yeah, for sure. So, you can find out more about our company Upptic, upptic.com, it’s spelled upptic and, in short, we’re a company that helps developers to grow their apps in a very profit focused way, between providing both consultation and execution services for actually running all aspects of your growth marketing, as well as providing tech that can be licensed for things such as App Store Optimization and campaign optimization.
Shamanth: Sure and we will link to Upptic and your LinkedIn in the show notes along with the transcript. For now, thank you so much for being on the Mobile User Acquisition Show.
Warren: It’s been my pleasure. Thanks so much.
A REQUEST BEFORE YOU GO
I have a very important favor to ask, which as those of you who know me know I donโt do often. If you get any pleasure or inspiration from this episode, could you PLEASE leave a review on your favorite podcasting platform โ be itย iTunes, Overcast, Spotify or wherever you get your podcast fix. This podcast is very much a labor of love – and each episode takes many many hours to put together. When you write a review, it will not only be a great deal of encouragement to us, but it will also support getting the word out about the Mobile User Acquisition Show.
Constructive criticism and suggestions for improvement are welcome, whether on podcasting platforms โ or by email to shamanth at rocketshiphq.com. We read all reviews & I want to make this podcast better.
Thank you โ and I look forward to seeing you with the next episode!